Metro Flow Prediction with Hierarchical Hypergraph Attention Networks
IEEE Transactions on Artificial Intelligence(2023)
摘要
Graph convolutional neural networks, which aim to learn the spatial correlation within multivariate time-series data, have achieved significant advancements in traffic prediction. Despite the proliferation of various spatio-temporal graph neural networks, a fundamental question of the pre-defined graphs still remain to be explored: how can the graph be dynamically optimized as time shifts? Especially for tasks such as rail transit where travel patterns of passengers change periodically. In other words, the spatial correlation of multivariate traffic time-series data is closely intertwined with temporal features, which are tricky to reflect through static graphs with handcrafted association. To adaptively learn the spatial correlation in feature domain, we propose Hierarchical Hypergraph Attention Networks (HHGAT). The hierarchical nature of the framework is reflected in both temporal and spatial dimensions. At different time spans, the model explores the hidden hyperedges with a local hypergraph attention mechanism and optimizes the hypergraph with a global attention mechanism. The node-level prediction results are then fused after the downstream tasks of spatio-temporal hypergraph convolutional networks. The effectiveness and accuracy of the proposed model are evaluated on historical datasets of Beijing and Hangzhou. Compared to the baselines, the proposed approach consistently demonstrates superior performance in various evaluation scenarios.
更多查看译文
关键词
Attention mechanism,graph neural network,hypergraph learning,traffic prediction
AI 理解论文
溯源树
样例
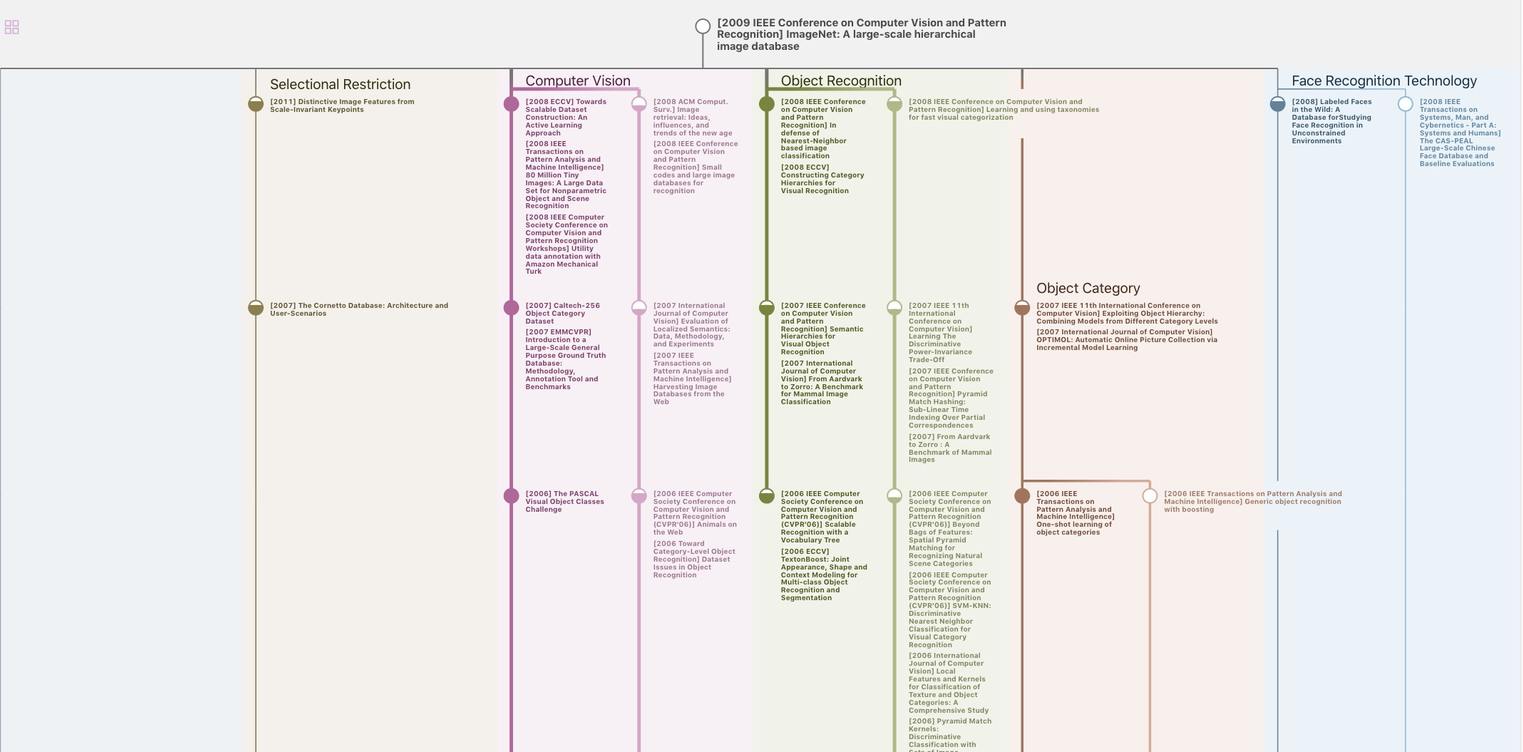
生成溯源树,研究论文发展脉络
Chat Paper
正在生成论文摘要