Patch Similarity Self-Knowledge Distillation for Cross-view Geo-localization
IEEE Transactions on Circuits and Systems for Video Technology(2023)
摘要
Cross-view geo-localization is an extremely challenging task due to drastic discrepancies in scene context and object scale between different views. Existing works normally concentrate on aligning the global appearance between two views but underestimate these two discrepancies. In practice, only a small region in the retrieved aerial image can be matched to the whole query ground image (
i.e
. scene context change). On the other hand, the retrieved aerial images are only able to describe the coarse-grained information but the query ground images can capture the fine-grained details (
i.e
. object scale change). In this paper, we propose a novel self-distillation framework called Patch Similarity Self-Knowledge Distillation (PaSS-KD), which provides the local and multi-scale knowledge as fine-grained location-related supervision to guide cross-view image feature extraction and representation in a self-enhanced manner. Specifically, we develop an auxiliary image-to-patch retrieval task to explore the scene context change and devise a multi-scale patch partition strategy to sense the object scale change across views. Additionally, our self-distilling framework can be removed to avoid additional computation cost at the inference stage. Extensive experiments show that our method not only achieves the state-of-the-art image retrieval performance on the CVUSA and CVACT benchmarks, but also significantly boosts the fine-grained localization accuracy on the VIGOR dataset. Remarkably, for 10 meter-level localization, we improve the relative accuracy by a factor of 0.8× and 1.6× on the VIGOR dataset under same-area and cross-area evaluation, respectively.
更多查看译文
关键词
Geo-localization,self-knowledge distillation,feature representation,image retrieval
AI 理解论文
溯源树
样例
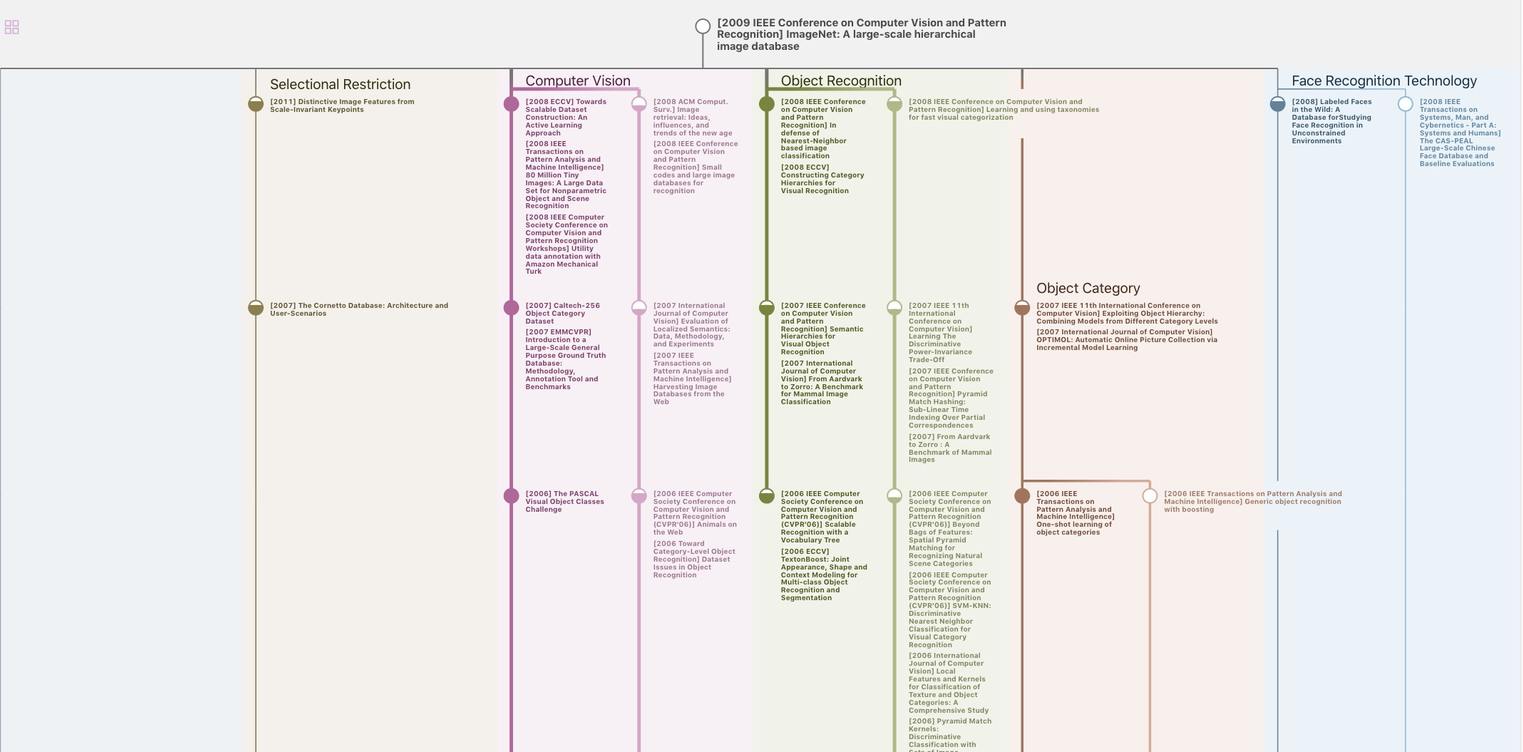
生成溯源树,研究论文发展脉络
Chat Paper
正在生成论文摘要