EFCPose: End-to-End Multi-Person Pose Estimation with Fully Convolutional Heads
IEEE Transactions on Circuits and Systems for Video Technology(2023)
摘要
Mainstream methods of multi-person pose estimation are not end-to-end. Recently, some methods build an end-to-end framework based on the DETR framework, aiming to eliminate the need for hand-crafted modules like heuristic grouping and NMS post-processing. However, these DETR-based methods suffer from a heavy memory burden of processing the high-resolution backbone feature maps with transformers. In this paper, we propose an end-to-end multi-person pose estimation method with a fully convolutional network, termed EFCPose. Different from DETR-based methods, it directly predicts instance-aware poses in a pixel-wise manner with lightweight convolutional heads, avoiding the heavy memory burden. Overall, our method adopts the center-offset formulation and a one-to-one label assignment strategy to achieve the multi-person pose estimation in an end-to-end manner. The main contribution of our fully convolutional heads includes two aspects. On the one hand, we propose an unaligned center-offset representation to learn more reliable semantic centers to replace the inconsistent geometric centers, improving the performance of instance detection. On the other hand, we propose a novel regression strategy named limb-aware adaptive regression, which leverages separate adaptive points to convert challenging long-range offsets into simplified short-range offsets and incorporates limb constraints to elevate the regression quality of joint offsets. Compared with current DETR-based end-to-end methods, EFCPose avoids high computational complexity and achieves higher accuracy. Extensive experiments on COCO Keypoint and CrowdPose benchmarks show that EFCPose outperforms other state-of-the-art bottom-up and single-stage methods without flipping augmentation.
更多查看译文
关键词
Human pose estimation,multi-person,end-to-end,deep learning
AI 理解论文
溯源树
样例
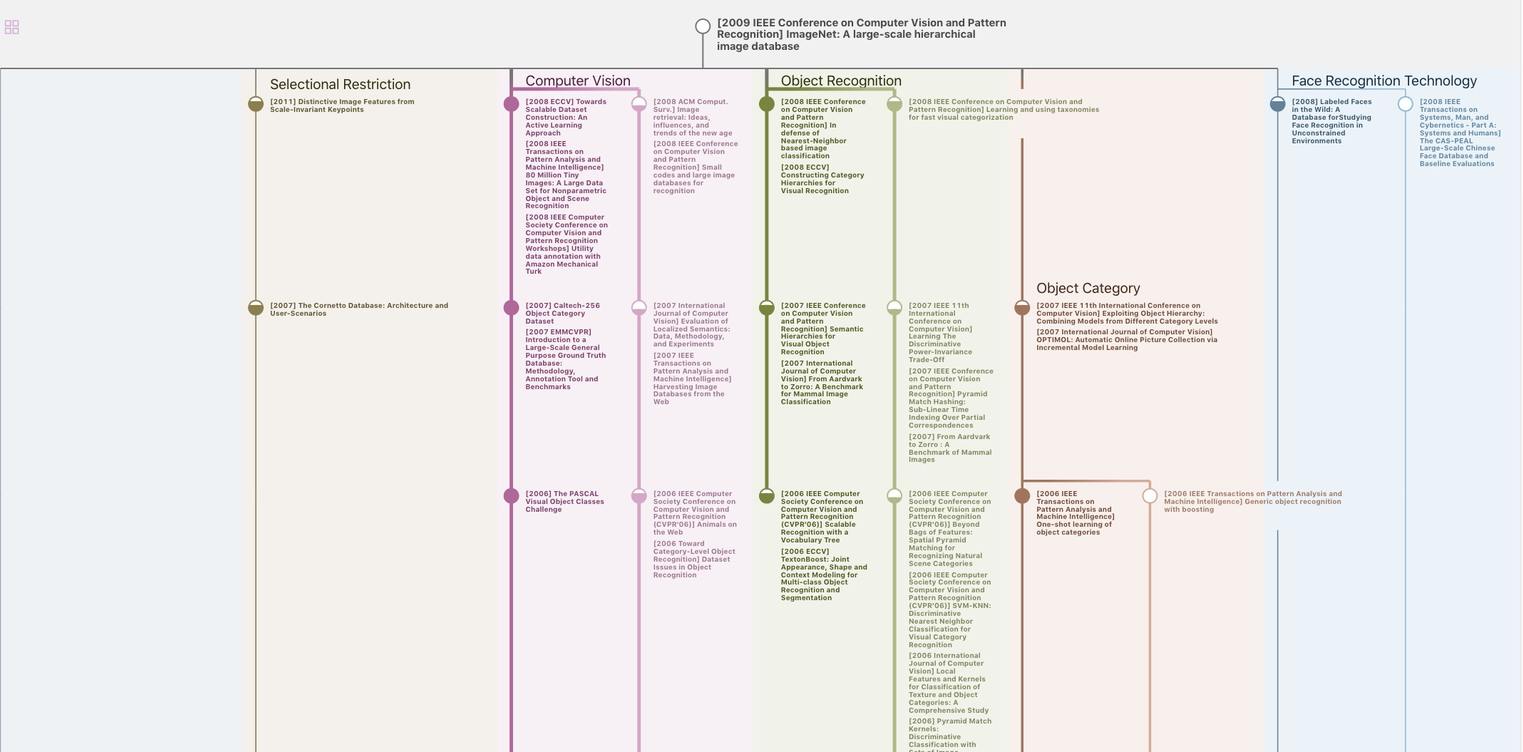
生成溯源树,研究论文发展脉络
Chat Paper
正在生成论文摘要