Automatic Modulation Classification for OFDM Systems Using Bi-Stream and Attention-Based CNN-LSTM Model
IEEE COMMUNICATIONS LETTERS(2024)
摘要
Existing deep learning (DL) models for automatic modulation classification (AMC) of orthogonal frequency division multiplexing (OFDM) signals consider only spatial or temporal attributes, ignoring feature interaction, which limits their ability to classify higher-order modulation formats. To address this, we propose a bi-stream and attention-based convolutional neural network and long short-term memory network (CNN-LSTM) for AMC. It can efficiently extract special and temporal features from the in-phase and quadrature (IQ) samples and the amplitude and phase (AP) of the received signal for AMC. Each stream consists of a CNN, LSTM, and attention module to extract features from signal patterns, which improves the classification performance. The proposed AMC can classify M-ary phase-shift keying (M-PSK) and higher-order M-ary quadrature amplitude modulation (M-QAM) formats in the presence of randomized carrier frequency offset, symbol timing offset, phase offset, and unknown channel state information. The proposed model outperforms existing models in terms of classification accuracy and complexity. Finally, the proposed model has been validated on the real-time dataset generated by the radio frequency testbed.
更多查看译文
关键词
Automatic modulation classification,deep learning,CNN,LSTM,attention,bi-stream,OFDM
AI 理解论文
溯源树
样例
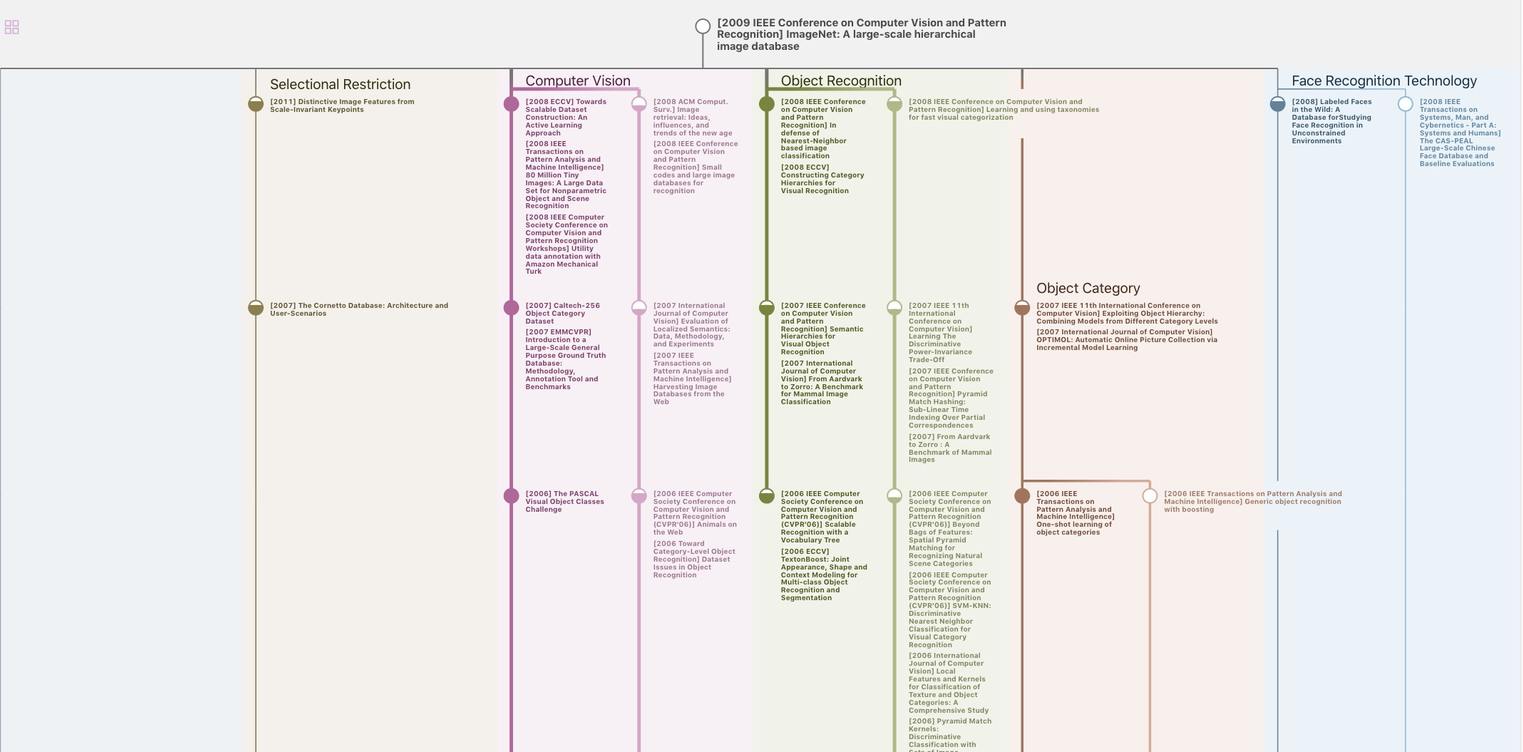
生成溯源树,研究论文发展脉络
Chat Paper
正在生成论文摘要