Radar-Based Human Activity Recognition Using Dual-Stream Spatial and Temporal Feature Fusion Network.
IEEE Trans. Aerosp. Electron. Syst.(2024)
摘要
Over the past few years, radar-based human activity recognition (HAR) has emerged as a prominent research area. The typical approach involves converting radar data into image data, such as micro-Doppler images, and then feeding them into networks for activity classification. However, relying solely on a single type of feature may restrict the ability of the networks to recognize activities accurately. To address this limitation and make the most of spatiotemporal features in the data, a dual-stream spatial and temporal feature fusion (DSTFF) network that leverages attention mechanism is proposed, which comprises a temporal feature extraction patial feature extraction stream (SFES) network. Additionally, a coordinates-based spatial attention mechanism (CSAM) is introduced to enhance the accuracy and efficiency of extracting deep spatial features. It focuses on the key spatial information from both horizontal and vertical directions of the feature map, which means range and velocity information in reality, respectively. Furthermore, it effectively connects the information across each channel. Meanwhile, a radar HAR (RadHAR) dataset based on two-dimensional (2D) and three-dimensional (3D) data is created by using a millimeter-wave radar. The evaluation experiments of the CSAM and the DSTFF network are carried out on the public dataset collected by the University of Glasgow (UOG) and the RadHAR dataset, respectively. The experimental results show that the CSAM exhibits strong generalization ability, and the DSTFF network presents effectiveness and superiority with the accuracy of 97.10%, which is higher than those of the classical and state-of-the-art networks.
更多查看译文
AI 理解论文
溯源树
样例
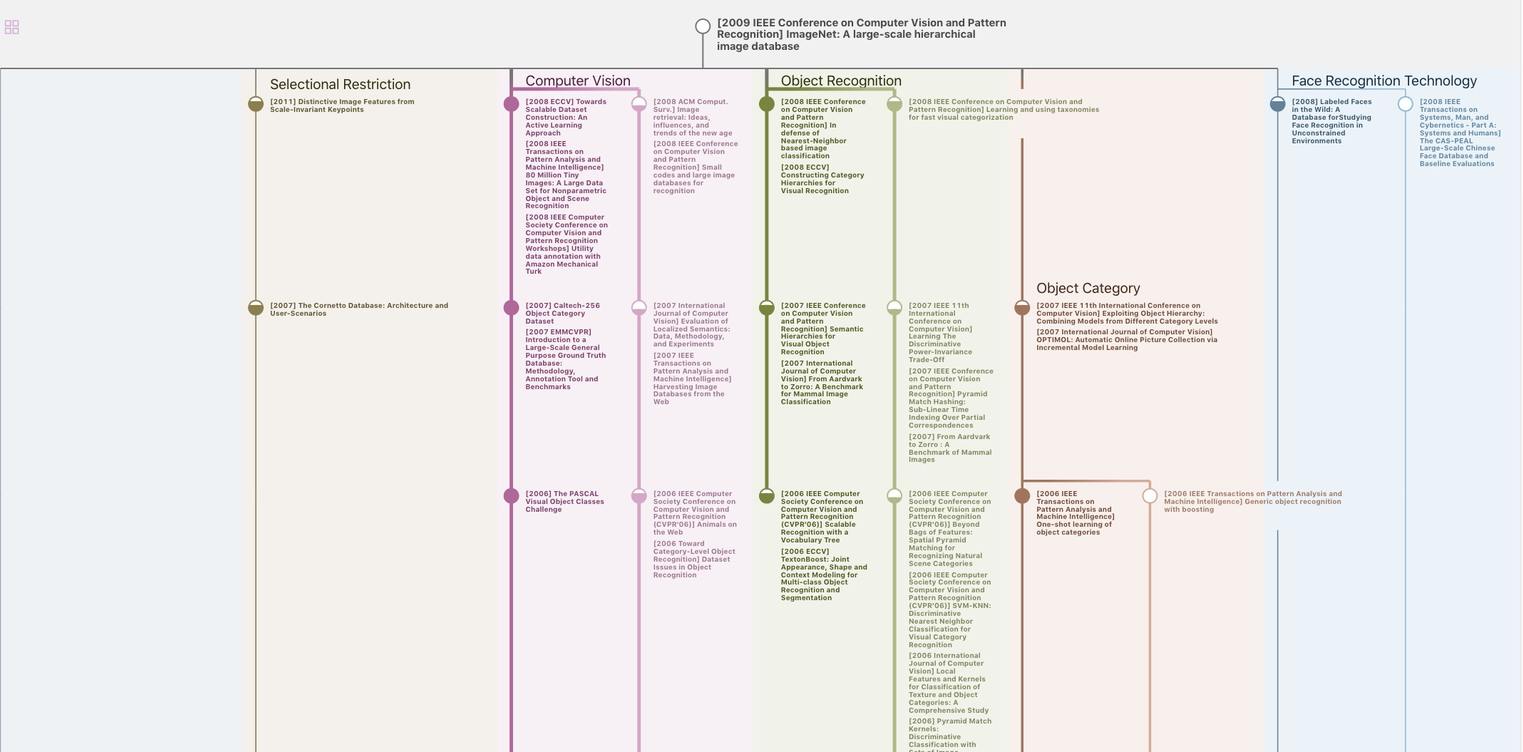
生成溯源树,研究论文发展脉络
Chat Paper
正在生成论文摘要