Spatial-temporal Multi-scale Constrained Learning for Mmwave-Based Human Pose Estimation
IEEE transactions on cognitive and developmental systems(2024)
摘要
It is a challenging task to reconstruct human pose from millimeter wave (mmWave) radar point clouds due to their sparsity and sensitivity to multipath noise. In order to enhance the inference ability of deep learning models in processing sparse radar point clouds, a learning paradigm with spatial and temporal multi-scale constraints is proposed, utilizing prior relationships in skeletal structure and joint movements in both time and space to constrain the learning of pose sequences. Specifically, the proposed spatial multi-scale constraint block learns the spatial constraint relationships of human joints using three different scales: adjacent joint constraint, part-level kinematic constraint, and global joint constraint, by which the spatial joint constraint features are aggregated by fusion gate mechanism. On the other hand, the temporal multi-scale constraint block is devised to learn the temporal constraint relationships of the joint trajectories using the information of the joint itself and local context information in time domain. Compared with the single-scale constrained learning paradigm, the potential advantage of the proposed method is that it can reduce the impact of random missing and noise in radar data. Finally, the effectiveness and superiority of the proposed method are fully demonstrated through the experimental results on two public datasets of human pose estimation based on mmWave radar.
更多查看译文
关键词
Radar,Point cloud compression,Millimeter wave communication,Feature extraction,Pose estimation,Sensors,Three-dimensional displays,Human pose estimation,multiscale joint constraint,sparse mmWave point clouds,spatial-temporal multiscale constrained learning
AI 理解论文
溯源树
样例
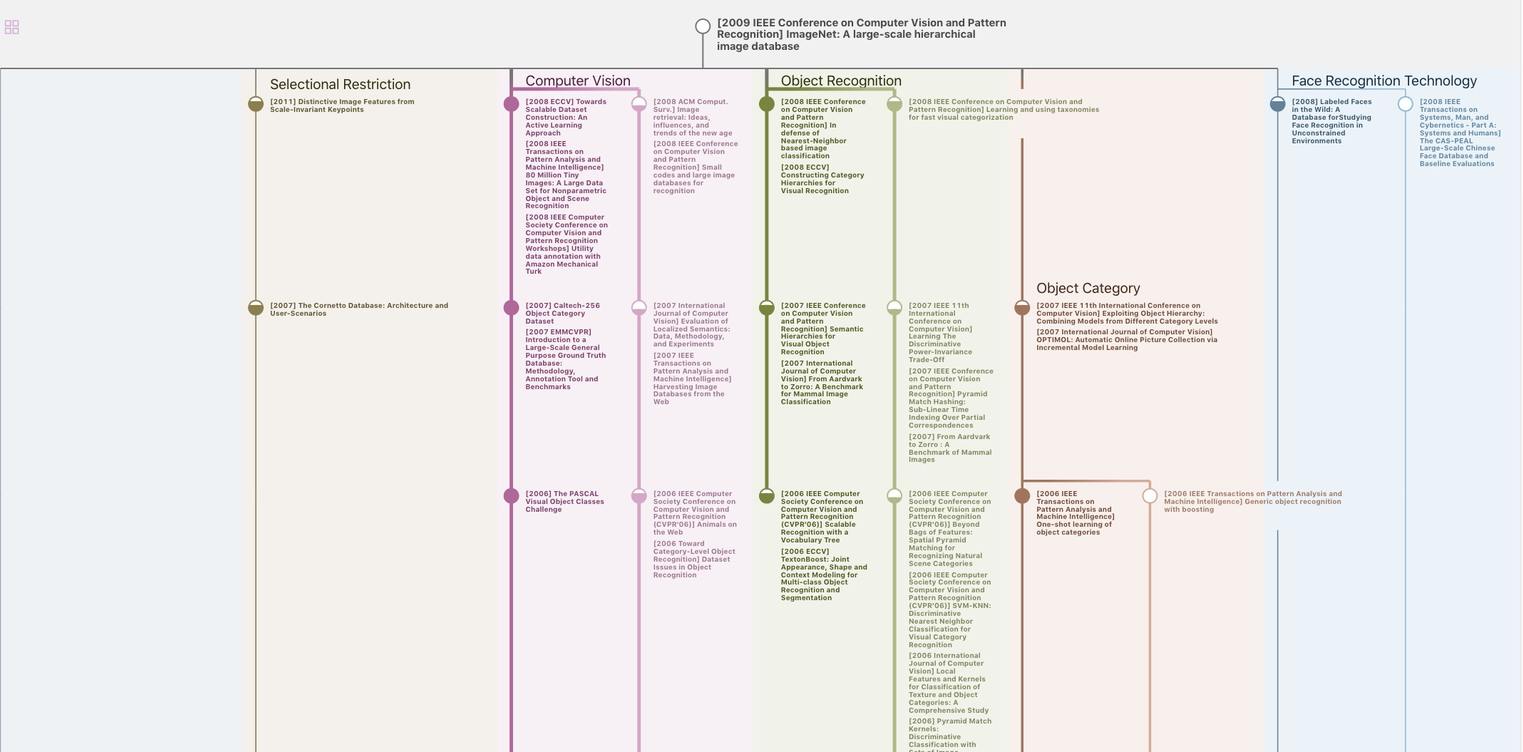
生成溯源树,研究论文发展脉络
Chat Paper
正在生成论文摘要