Hybrid Batch-Normalized Deep Feedforward Neural Network Incorporating Polynomial Regression for High-Dimensional Microwave Modeling
IEEE TRANSACTIONS ON CIRCUITS AND SYSTEMS I-REGULAR PAPERS(2024)
摘要
This paper proposes a new hybrid structure and microwave modeling method that combines polynomial regression with batch-normalized deep feedforward neural network (BN-DFN) to be used in high-dimensional microwave circuit modeling. Utilizing the proposed BN-DFN method results in a remarkably faster training procedure compared to the conventional DFN. In addition, the superiority of the BN-DFN method over DFN in terms of accuracy prepares this opportunity to perform high-dimensional microwave modeling using fewer training data in comparison with the modeling with conventional DFN. The results show that a data reduction of about 40-80% can be achieved for microwave applications used in this paper using the proposed method. Also, in this paper, a hybrid polynomial regression BN-DFN (HPBN-DFN) is proposed to further improve the accuracy of the proposed BN-DFN method. The proposed HPBN-DFN method fine-tunes the predicted values of the BN-DFN by passing them through a polynomial regression stage for increasing accuracy. The proposed methods are verified through two high-dimensional parameter-extraction modeling examples of microwave filters.
更多查看译文
关键词
Couplings,Batch normalization,deep learning,deep neural networks,high dimension,microwave modeling,polynomial regression,parameter extraction
AI 理解论文
溯源树
样例
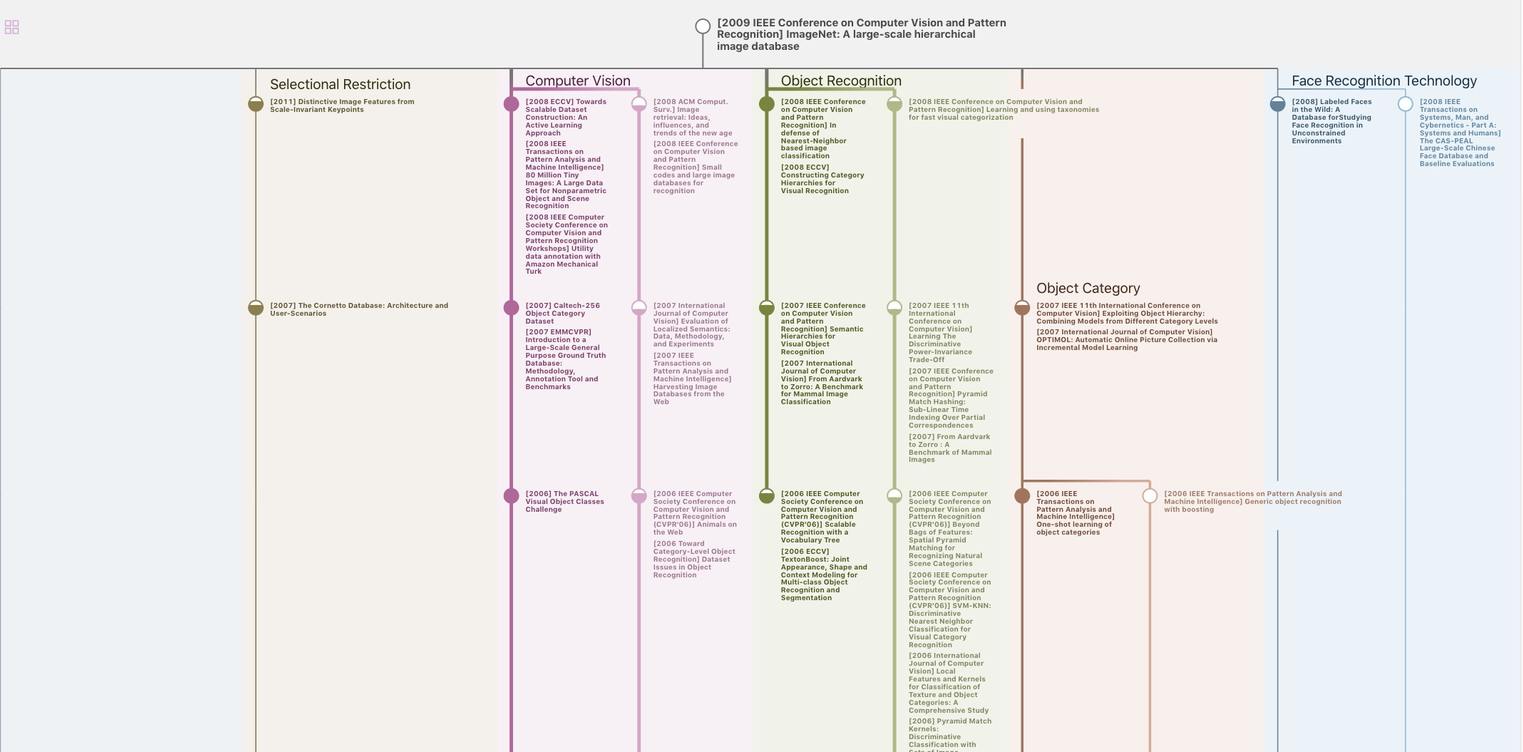
生成溯源树,研究论文发展脉络
Chat Paper
正在生成论文摘要