Dynamic Threshold Distribution Domain Adaptation Network: A Cross-Subject Fatigue Recognition Method Based on EEG Signals
IEEE TRANSACTIONS ON COGNITIVE AND DEVELOPMENTAL SYSTEMS(2024)
摘要
Electroencephalogram (EEG) has been widely used in driver fatigue recognition due to its high-resolution and noninvasive characteristics in recent years. However, EEG signals have strong variability and significant individual differences, which put forward extremely high requirements on the generalization ability of the model in practical use. To address these issues, a dynamic threshold distribution domain adaptation network (DTDDAN) is proposed to classify the EEG signals for driver fatigue recognition. In the model, the domain discriminator is introduced to alleviate the marginal distribution difference of different domains, and a new loss, Jensen-Shannon loss (JS loss), is applied to relieve the conditional distribution difference of different domains to learn cross-subject invariant deep features of EEG signals. In addition, we propose a dynamic threshold pseudo label strategy to assign pseudo labels to the samples of the target domain in the training process of the model. This strategy can make sure that the high-quality unlabeled data of the target domain contribute to the model training and the model can fully learn the information of the target domain. With extensive experiments on our self-constructed EEG-based fatigue driving data set, competitive performance is achieved for the cross-subject classification task, with average classification sensitivity, specificity, and accuracy of 89.8%, 93.8%, and 92.2%, respectively. And it is shown that our model can effectively extract cross-subject invariant EEG signals deep features by the experimental results.
更多查看译文
关键词
Fatigue,Electroencephalography,Feature extraction,Brain modeling,Adaptation models,Task analysis,Vehicles,Domain adaptation,electroencephalogram (EEG),fatigue recognition,pseudo label
AI 理解论文
溯源树
样例
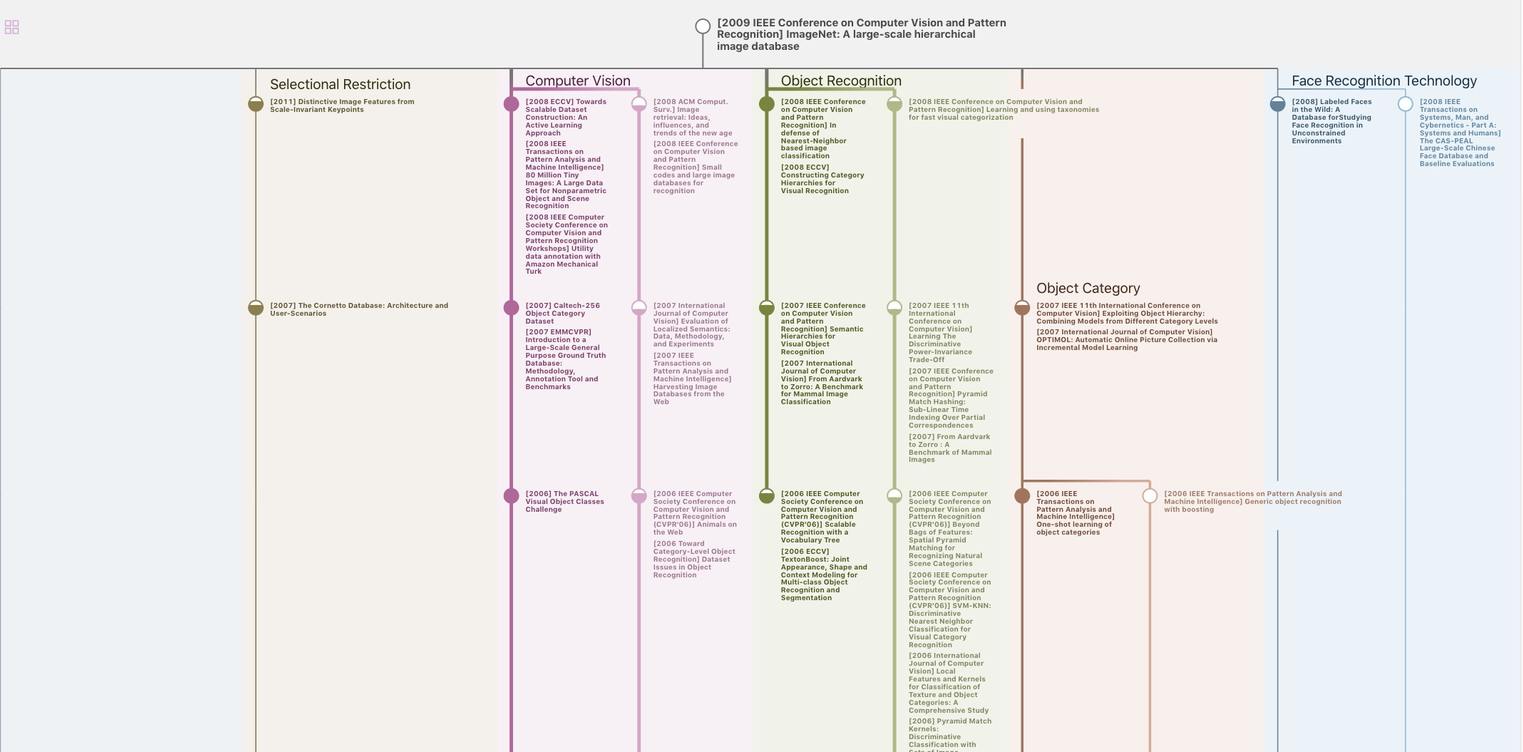
生成溯源树,研究论文发展脉络
Chat Paper
正在生成论文摘要