FedStar: Efficient Federated Learning On Heterogeneous Communication Networks
IEEE Transactions on Computer-Aided Design of Integrated Circuits and Systems(2023)
摘要
The proliferation of multi-media applications and increased computing power of mobile devices have led to the development of personalized artificial intelligent (AI) applications that utilize the massive user-information residing on them. However, the traditional centralized training paradigm is not applicable in this scenario due to potential privacy risks and high communication overhead. Federated learning (FL) provides an option to these applications. Nevertheless, the heterogeneity of computing and communication latency among devices have posed great challenges to building efficient learning frameworks. Existing optimizations on FL either fail to speed up training on heterogeneous devices or suffer from poor communication efficiency. In this paper, we propose FedStar, an efficient FL framework that supports decentralized asynchronous training on heterogeneous communication networks. Considering the heterogeneous computing power in the network, FedStar supports running heterogeneity-aware local steps on each device. What’s more, considering the heterogeneous communication latency and possibly unreachable communication path between some devices, FedStar generates a decentralized communication topology that can achieve maximal training throughput. Finally, it adopts weighted aggregation to guarantee high convergence accuracy of global model. Theoretical analysis results show the convergence behaviour of FedStar under non-convex settings. Experimental results show that FedStar can achieve a speedup of 4.81× than the state-of-the-art FL schemes with high convergence accuracy.
更多查看译文
关键词
Artificial intelligent,federated learning,heterogeneous networks,edge computing
AI 理解论文
溯源树
样例
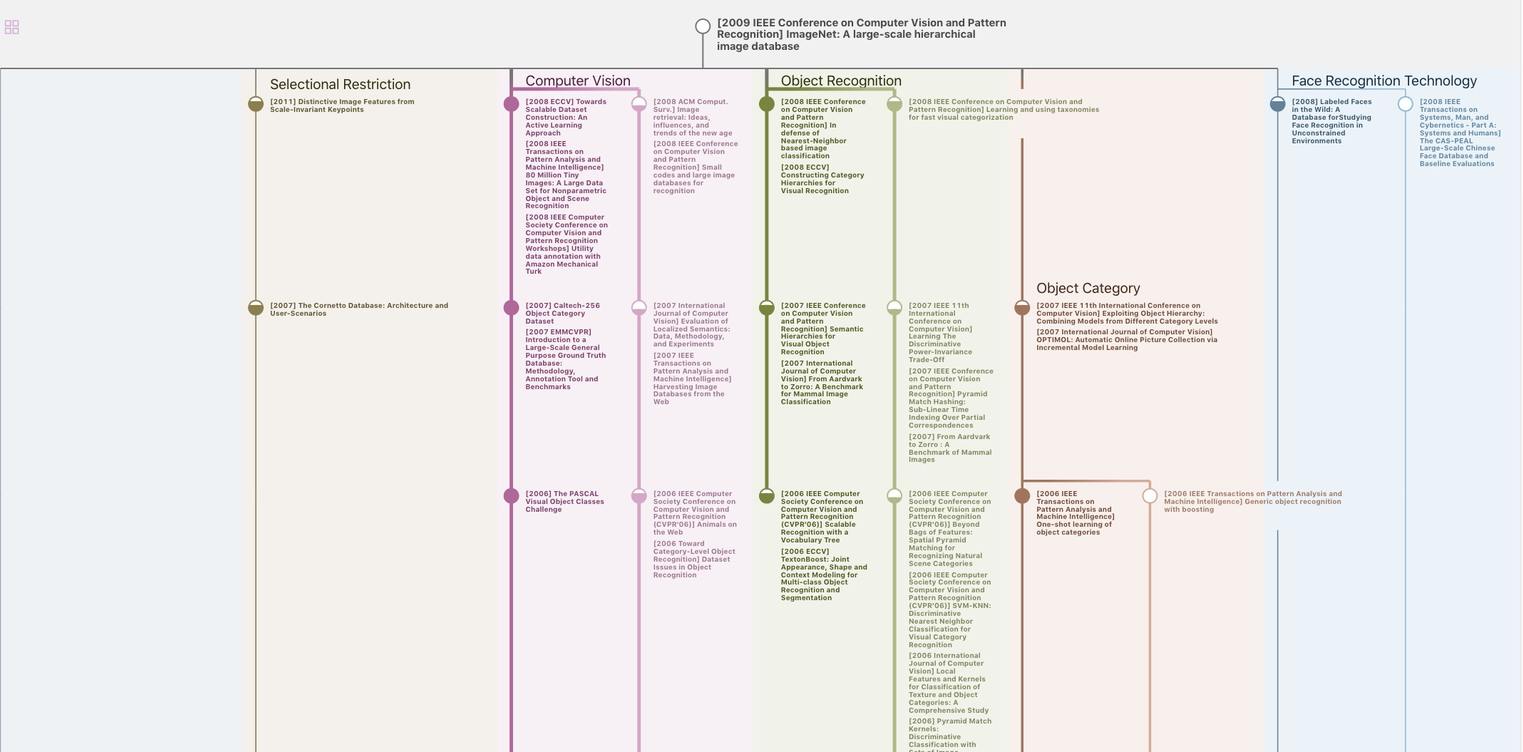
生成溯源树,研究论文发展脉络
Chat Paper
正在生成论文摘要