An Automatic Neural Network Architecture-and-Quantization Joint Optimization Framework for Efficient Model Inference.
IEEE Trans. Comput. Aided Des. Integr. Circuits Syst.(2024)
摘要
Efficient deep learning models, especially optimized for edge devices, benefit from low inference latency to efficient energy consumption. Two classical techniques for efficient model inference are lightweight neural architecture search (NAS), which automatically designs compact network models, and quantization, which reduces the bit-precision of neural network models. As a consequence, joint design for both neural architecture and quantization precision settings is becoming increasingly popular. There are three main aspects that affect the performance of the joint optimization between neural architecture and quantization: quantization precision selection (QPS), quantization aware training (QAT), and neural architecture searching (NAS). However, existing works focus on at most twofold of these aspects, and result in secondary performance. To this end, we proposed a novel automatic optimization framework, DAQUDAQU is an ancient liquor fermentation process., that allows jointly searching for Pareto-optimal neural architecture and quantization precision combination among more than 1047 quantized subnet models. To overcome the instability of the conventional automatic optimization framework, DAQU incorporates a warm-up strategy to reduce the accuracy gap among different neural architectures, and a precision-transfer training approach to maintain flexibility among different quantization precision settings. Our experiments show that the quantized lightweight neural networks generated by DAQU consistently outperform state-of-the-art NAS and quantization joint optimization methods.
更多查看译文
关键词
Neural architecture search,network quantization,automatic joint optimization,efficient model inference
AI 理解论文
溯源树
样例
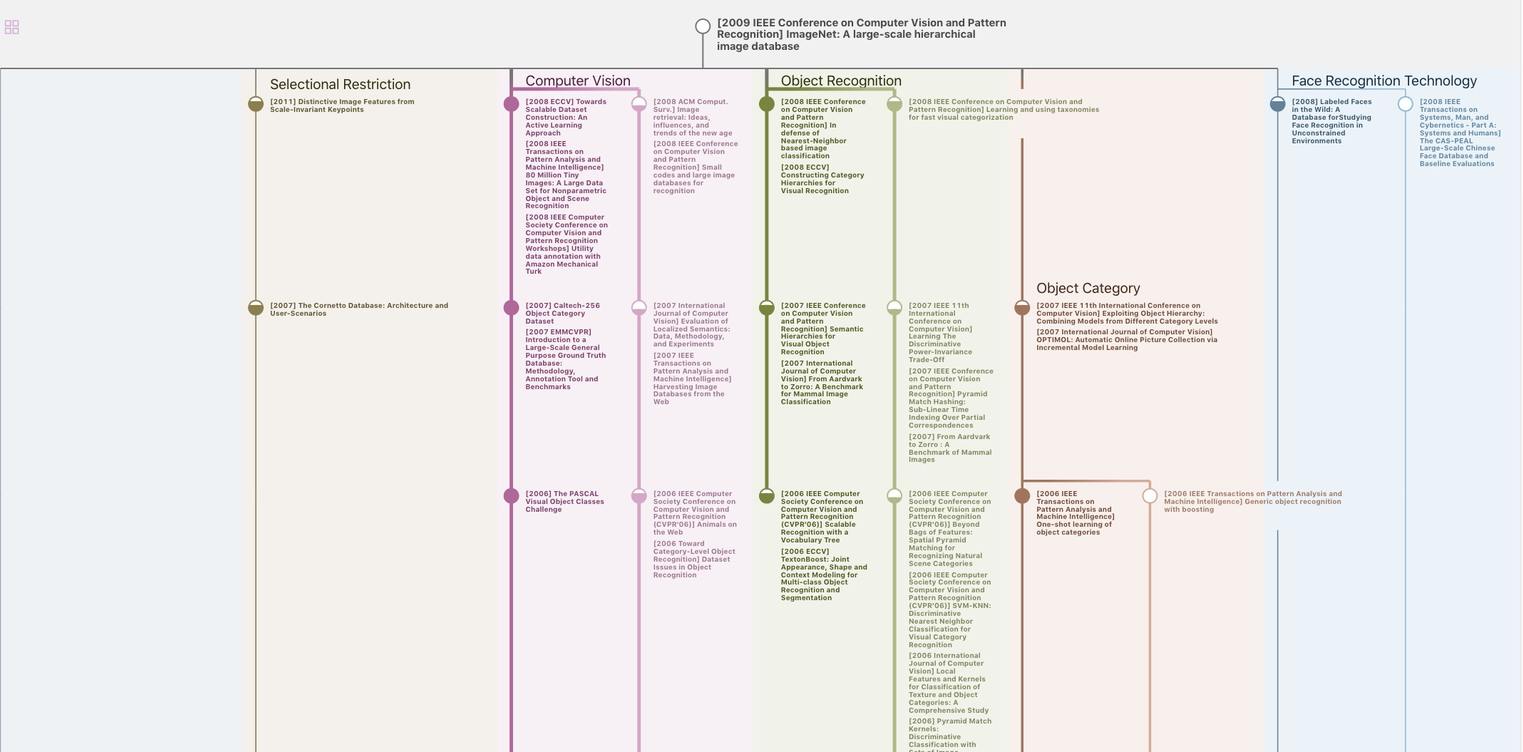
生成溯源树,研究论文发展脉络
Chat Paper
正在生成论文摘要