Fast Aging-Aware Timing Analysis Framework With Temporal-Spatial Graph Neural Network
IEEE Transactions on Computer-Aided Design of Integrated Circuits and Systems(2023)
摘要
With the downscaling of CMOS technology, device aging induced by hot carrier injection and bias temperature instability effects poses severe challenges to timing analysis of digital circuits. In this work, a fast aging-aware timing analysis framework based on temporal-spatial graph neural network is proposed for the first time. The temporal-spatial graph neural network takes gated tanh unit (GTU) as the temporal network to extract devices’ degradation from dynamic biases, and takes inductive GraphSAGE as the spatial network to obtain whole graph information from circuit topology and output circuit aging delay. With comprehensive comparison among the network candidates, the combination of gated tanh unit (GTU) and GraphSAGE presents the highest accuracy in predicting the standard cell aging delay. Owing to the superior features capture capability, this framework significantly improves the aging prediction efficiency under various operation conditions, especially facing the iterations of usage scenario, design version and process design kit. Compared with the conventional flow, the average acceleration ratio of our temporal-spatial network in predicting aging delay is more than 200 times. Furthermore, this framework is demonstrated with ADDER and FIFO circuits in timing analysis at the end of life. Thus, this work is helpful to the aging-aware circuit design in nano-scale technology.
更多查看译文
关键词
aging-aware timing analysis,aging delay,circuits reliability,temporal networks,spatial network
AI 理解论文
溯源树
样例
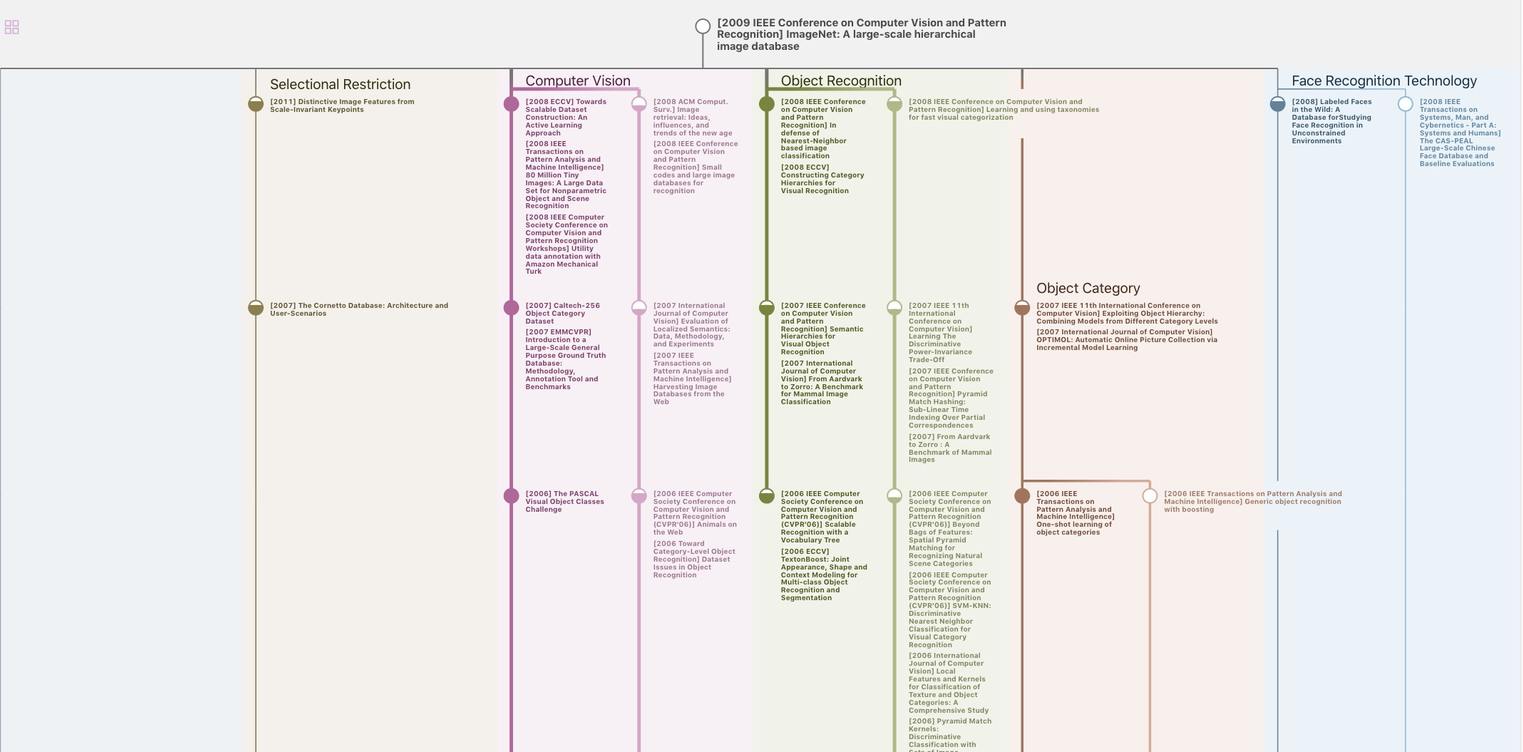
生成溯源树,研究论文发展脉络
Chat Paper
正在生成论文摘要