Deep Learning-Driven Anomaly Detection for Green IoT Edge Networks
IEEE transactions on green communications and networking(2023)
摘要
The widespread use of sensor devices in IoT networks imposes a significant burden on energy consumption at the network’s edge. To address energy concerns, a prompt anomaly detection strategy is required on demand for troubleshooting resource-constrained IoT devices. It enables devices to adapt their configuration according to the dynamic signal quality and transmission settings. However, obtaining accurate energy data from IoT nodes without external devices is unfeasible. This paper proposes a framework for energy anomaly detection of IoT nodes using data transmission analysis. We use a public dataset that contains peer-to-peer IoT communication energy and link quality data. Our framework first utilizes linear regression to analyze and identify the dominant features of data communication for IoT transceivers. Later, a deep neural network modifies the gradient flow to focus on the dominant features. This modification improves the detection accuracy of anomalies by minimizing the associated reconstruction error. Finally, the energy stabilization feedback provides nodes with insight to change their transmission configuration for future communication. The experimental results show that the proposed approach outperforms other unsupervised models in anomalous energy detection. It also proves that redesigning the conventional loss function by enhancing the impact of our dominant features can dramatically improve the reliability of the anomaly detection method.
更多查看译文
关键词
Peer-to-peer computing,Energy consumption,Wireless sensor networks,Optimization,Internet of Things,Protocols,Data communication,Transmission protocol,anomaly detection,edge networks,unsupervised learning,IoT,energy consumption,power consumption,link quality,deep learning,low power,wireless sensor networks,smart city
AI 理解论文
溯源树
样例
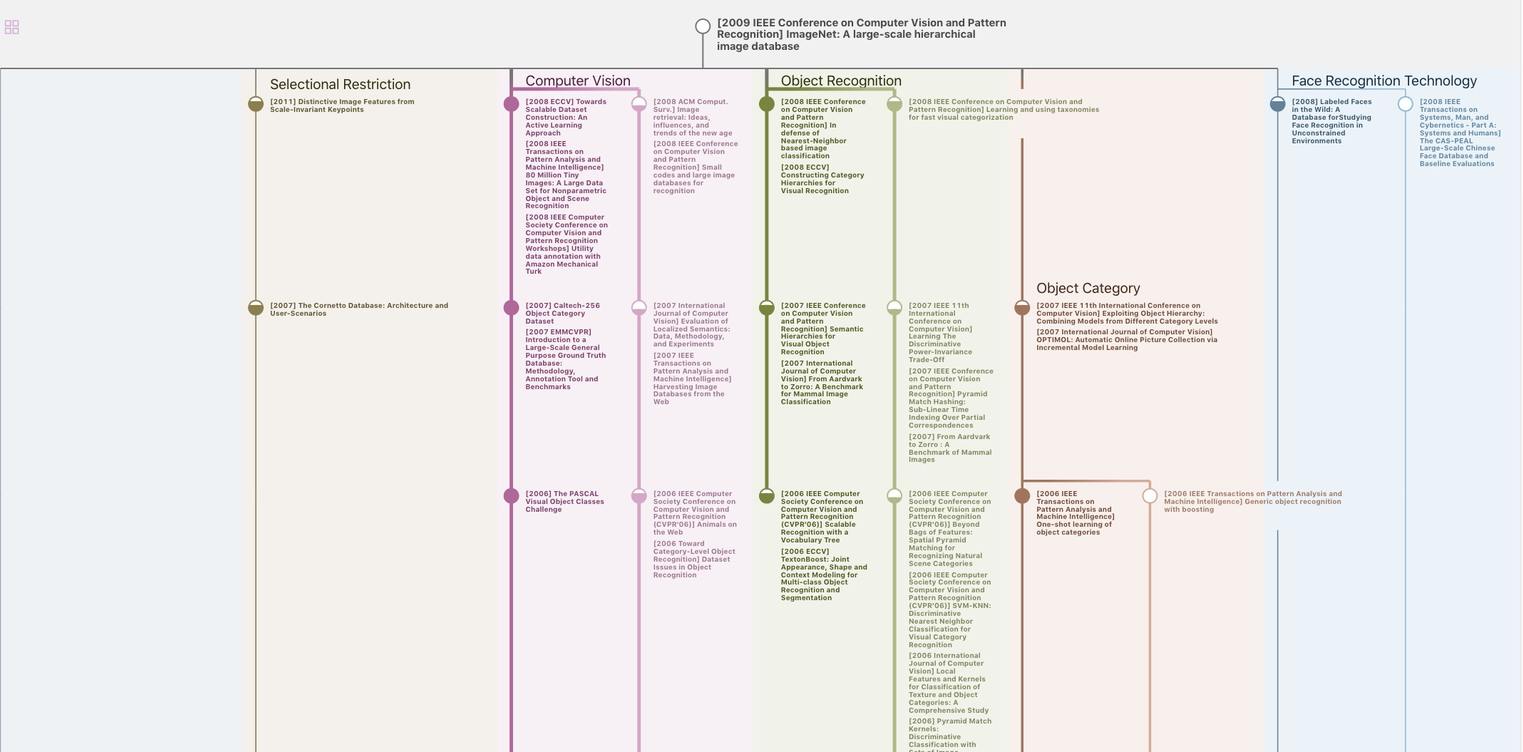
生成溯源树,研究论文发展脉络
Chat Paper
正在生成论文摘要