Attention-based Intrinsic Reward Mixing Network for Credit Assignment in Multi-Agent Reinforcement Learning
IEEE Transactions on Games(2023)
摘要
Credit assignment is a critical problem in cooperative Multi-Agent Reinforcement Learning (MARL). To address this problem, current studies mainly rely on the intrinsic reward, which is directly summed with the global reward to generate a total reward. However, such kinds of intrinsic reward functions ignore the dependence among agents and inevitably limit the adaptivity and effectiveness of MARL methods. In this paper, we propose a novel method, Attention-based Intrinsic Reward Mixing Network (AIRMN), for credit assignment in MARL. Specifically, we design a new intrinsic reward network on the basis of the attention mechanism, in order to enhance the effectiveness of teamwork. Besides, we devise a new mixing network that combines the intrinsic and extrinsic rewards in a nonlinear and dynamic manner, so as to adapt the total reward to the variation of the environment. Experimental results on the battle games of StarCraft II demonstrate that AIRMN outperforms the state-of-the-art methods in terms of the average test win rate, and also validate that AIRMN can dynamically return the precise intrinsic reward to each agent based on their contributions to the team cooperation, thereby better dealing with the credit assignment problem.
更多查看译文
关键词
Multi-Agent Reinforcement Learning,Attention Mechanism,Mixing Network,Intrinsic Reward,Credit Assignment
AI 理解论文
溯源树
样例
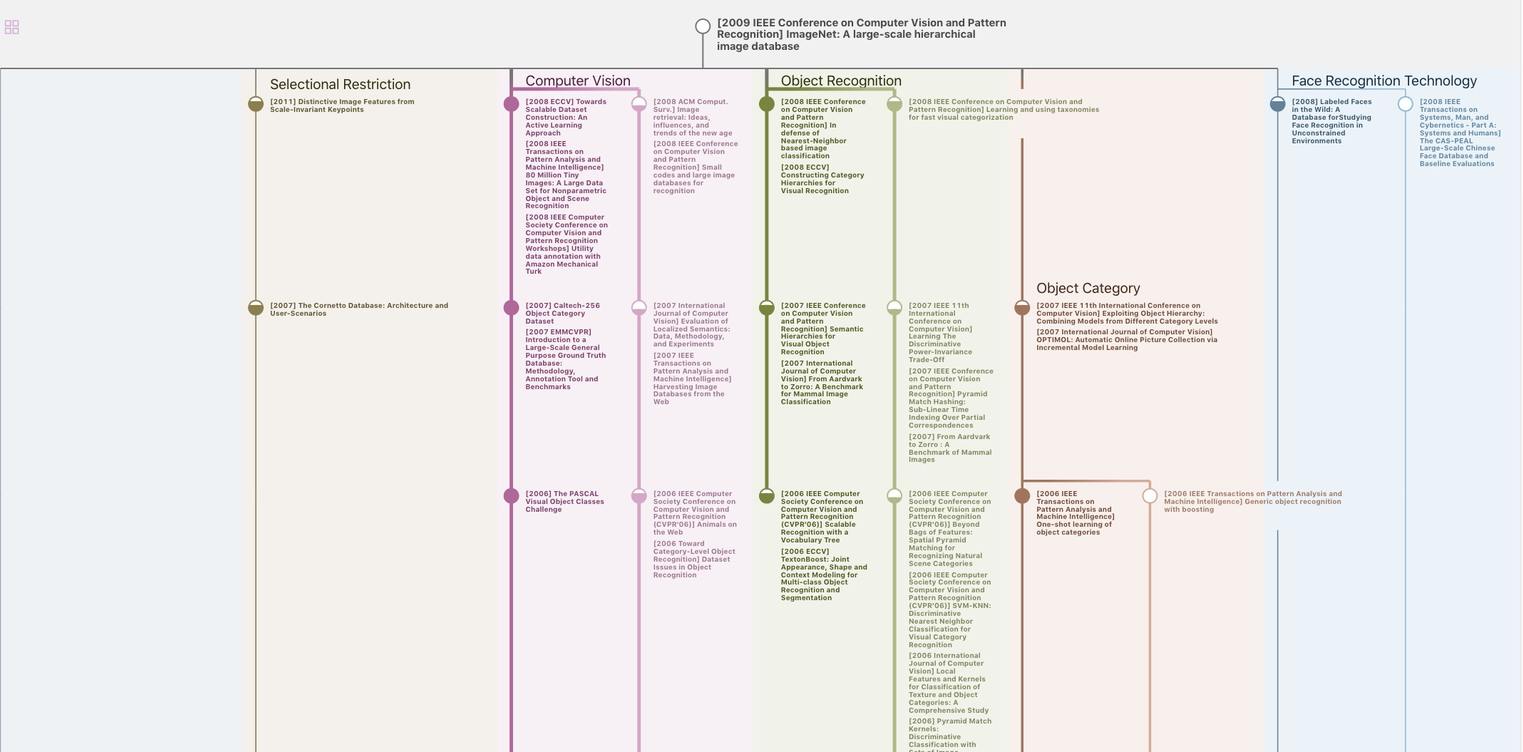
生成溯源树,研究论文发展脉络
Chat Paper
正在生成论文摘要