Defending Video Recognition Model Against Adversarial Perturbations Via Defense Patterns
IEEE Transactions on Dependable and Secure Computing(2023)
摘要
Deep Neural Networks (DNNs) have been widely successful in various domains, but they are vulnerable to adversarial attacks. Recent studies have also demonstrated that video recognition models are susceptible to adversarial perturbations, but the existing defense strategies in the image domain do not transfer well to the video domain due to the lack of considering temporal development and require a high computational cost for training video recognition models. This paper, first, investigates the temporal vulnerability of video recognition models by quantifying the effect of temporal perturbations on the model's performance. Based on these investigations, we propose Defense Patterns (DPs) that can effectively protect video recognition models by adding them to the input video frames. The DPs are generated on top of a pre-trained model, eliminating the need for retraining or fine-tuning, which significantly reduces the computational cost. Experimental results on two benchmark datasets and various action recognition models demonstrate the effectiveness of the proposed method in enhancing the robustness of video recognition models.
更多查看译文
关键词
Defense patterns,video adversarial defense,robust video recognition
AI 理解论文
溯源树
样例
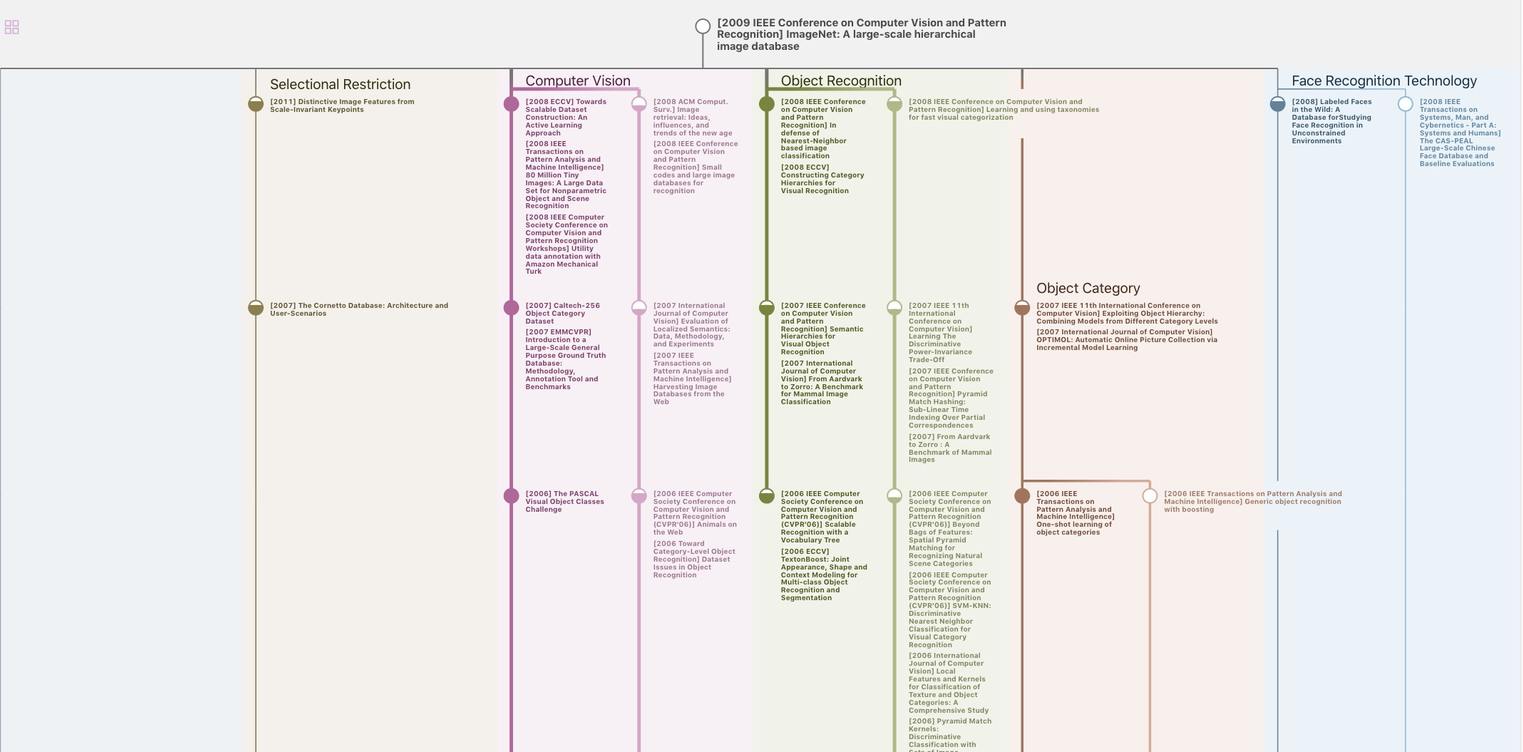
生成溯源树,研究论文发展脉络
Chat Paper
正在生成论文摘要