Enhancing Grid-Interactive Buildings Demand Response: Sequential Update-Based Multi-Agent Deep Reinforcement Learning Approach
IEEE internet of things journal(2024)
摘要
Demand response (DR) programs aim to improve grid reliability, reduce customer costs, and manage building energy demand. However, coordinating autonomous demand response in grid-interactive buildings faces challenges due to uncertainties in system model parameters, complex energy demands, and interdependent operational constraints. Specifically, the energy management problem in grid-interactive buildings is formulated as a Partially Observable Markov Decision Process (POMDP), where each building has only local information and no knowledge of other buildings’ behavior. This makes achieving consistent coordinated energy management challenging. To address these issues, this paper proposes a novel approach called Sequential Update-based Multi-Agent Deep Reinforcement Learning (SUMADRL). This approach updates the policy of each building sequentially, considering the updates already made by previous buildings. This ensures that the joint policy update of buildings is always highly aligned with the overall energy optimization goal and improves coordinated energy management among buildings. Additionally, the sequential update scheme provides theoretical guarantees for achieving monotonically improving rewards and converging to Nash equilibrium, enhancing credibility and stability. Experimental results show that the proposed method significantly reduces energy costs, achieves efficient load shaping, and demonstrates superior effectiveness and stability in demand response compared to the Multi-Agent Deep Deterministic Policy Gradient (MADDPG) algorithm.
更多查看译文
关键词
Buildings,Energy management,Costs,Demand response,Behavioral sciences,Thermal loading,Computational modeling,Demand response (DR),grid-interactive buildings,multiagent deep reinforcement learning (MADRL),sequential update scheme
AI 理解论文
溯源树
样例
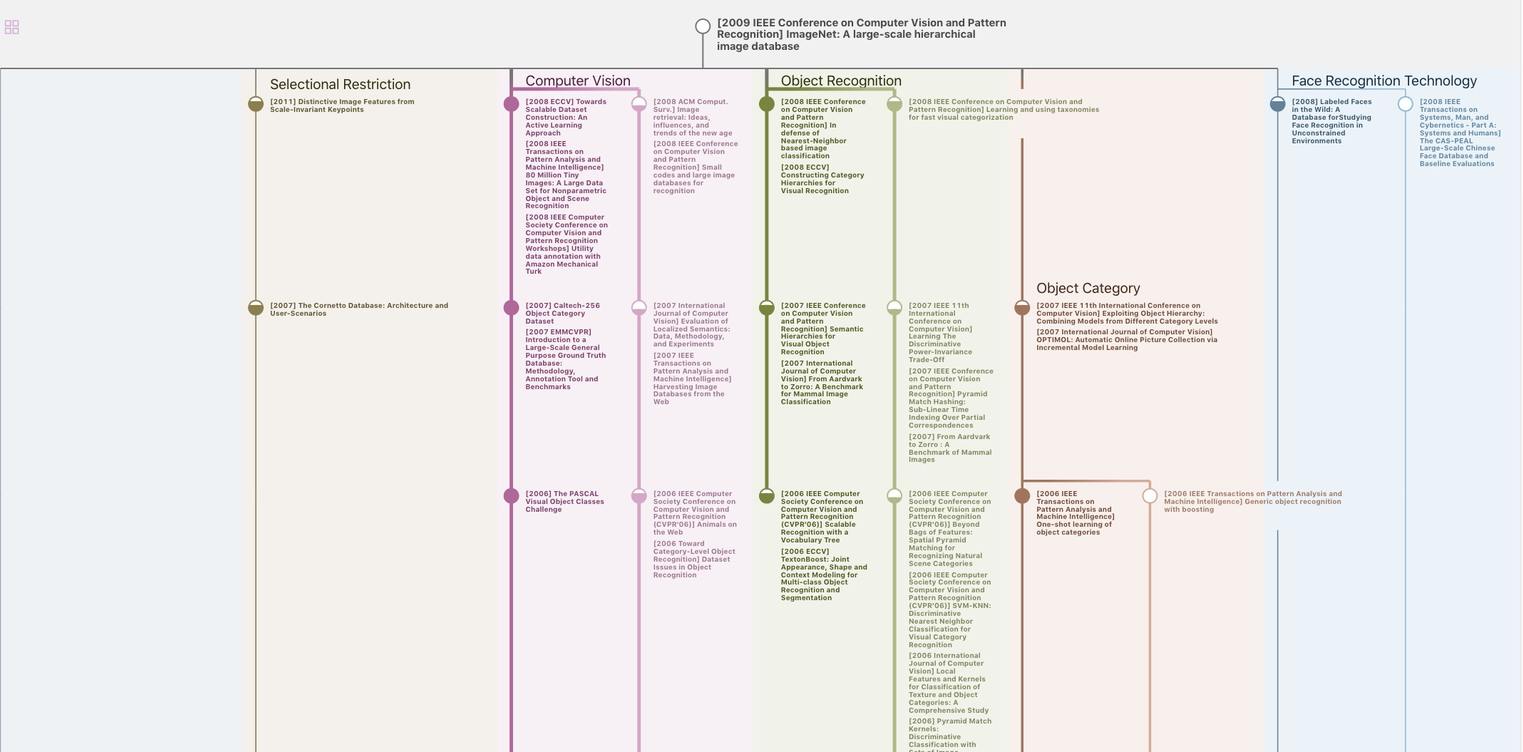
生成溯源树,研究论文发展脉络
Chat Paper
正在生成论文摘要