Few-Shot Learning With Multi-Granularity Knowledge Fusion and Decision-Making.
IEEE Trans. Big Data(2024)
摘要
Few-shot learning (FSL) is a challenging task in classifying new classes from few labelled examples. Many existing models embed class structural knowledge as prior knowledge to enhance FSL against data scarcity. However, they fall short of connecting the class structural knowledge with the limited visual information which plays a decisive role in FSL model performance. In this paper, we propose a unified FSL framework with multi-granularity knowledge fusion and decision-making (MGKFD) to overcome the limitation. We aim to simultaneously explore the visual information and structural knowledge, working in a mutual way to enhance FSL. On the one hand, we strongly connect global and local visual information with multigranularity class knowledge to explore intra-image and inter-class relationships, generating specific multi-granularity class representations with limited images. On the other hand, a weight fusion strategy is introduced to integrate multi-granularity knowledge and visual information to make the classification decision of FSL. It enables models to learn more effectively from limited labelled examples and allows generalization to new classes. Moreover, considering varying erroneous predictions, a hierarchical loss is established by structural knowledge to minimize the classification loss, where greater degree of misclassification is penalized more. Experimental results on three benchmark datasets show the advantages of MGKFD over several advanced models.
更多查看译文
关键词
Few-shot learning,multi-granularity,knowledge fusion,decision-making
AI 理解论文
溯源树
样例
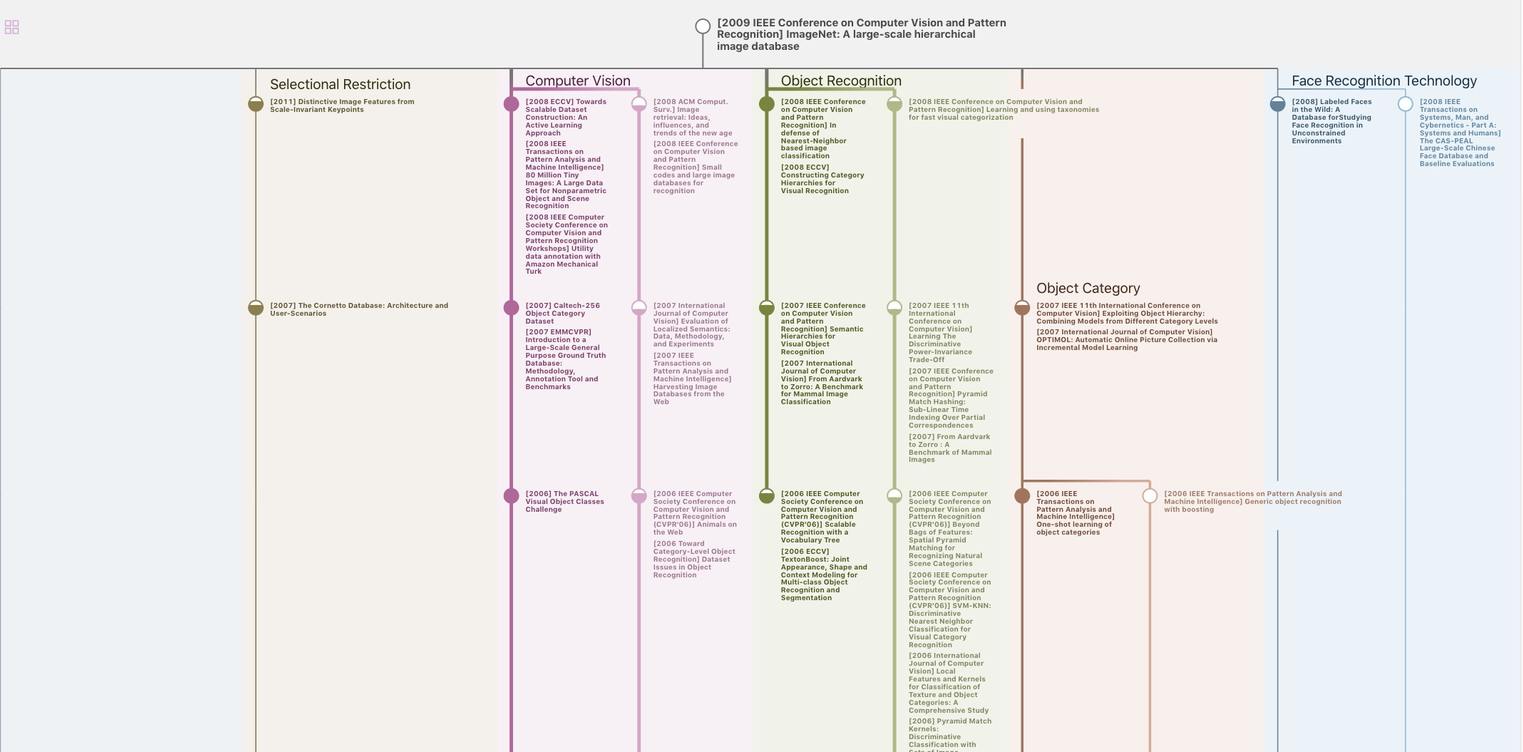
生成溯源树,研究论文发展脉络
Chat Paper
正在生成论文摘要