UAV-Assisted Real-Time Video Transmission for Vehicles: A Soft Actor-Critic DRL Approach.
IEEE Internet Things J.(2024)
摘要
With the rapid development of Internet of things (IoT) and mobile communication technology, Internet of vehicles (IoV) has potential prospects in providing live streaming services and improving the quality of experience (QoE) of ground mobile vehicles (GMVs). However, when the communication link is blocked in emergency situations such as road congestion and base station (BS) paralysis, it is still an arduous task to provide realtime high-definition video for vehicle users. Fortunately, as the aerial mobile BS, unmanned aerial vehicle (UAV) is expected to be deployed for emergency communication recovery in extreme scenarios. But high mobility of GMVs and UAV leads to dynamic changes of network topology, how to establish a stable communication link between UAV and GMVs to ensure the QoE of vehicle users has become a huge challenge. Therefore, this study proposes a novel live video transmission scheme based on scalable video coding (SVC). By jointly optimizing the UAV trajectory, video layer selection, and bandwidth resource allocation, our objective is to maximize long-term QoE while reducing UAV’s flight energy consumption. To maximize QoE, time delay and bitrate switching at GMVs’ receiver are set as penalty items while maximizing the reward of video quality. The joint optimization problem is modeled as a Markov decision process (MDP), and is solved by the soft actor-critic (SAC) deep reinforcement learning (DRL) algorithm, which is based on the maximum entropy framework. Extensive simulation results based on real-world datasets show that compared with other DRL algorithms, the proposed scheme has excellent performance in all respects.
更多查看译文
关键词
UAV,scalable video coding,trajectory optimization,energy consumption control,soft actor-critic
AI 理解论文
溯源树
样例
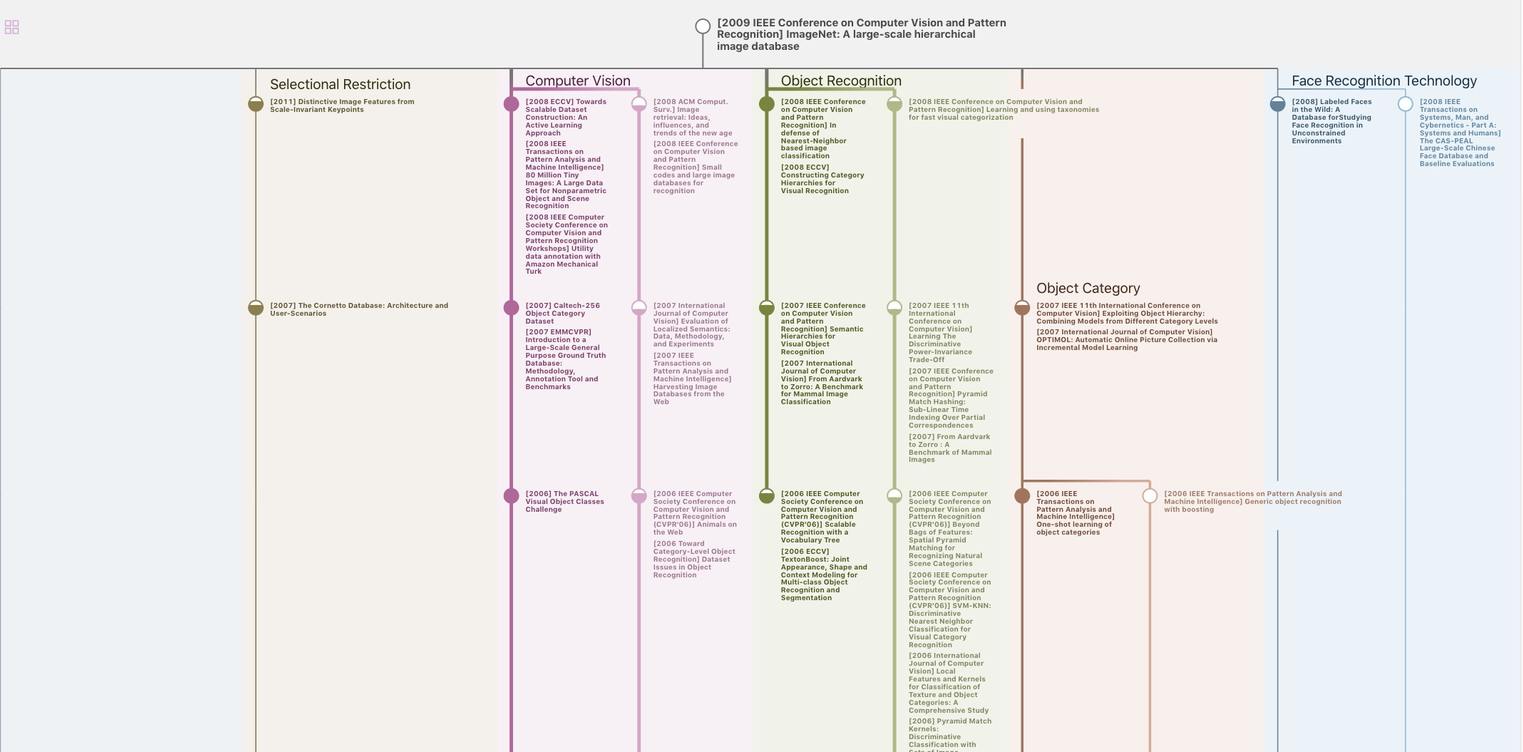
生成溯源树,研究论文发展脉络
Chat Paper
正在生成论文摘要