Optimizing Multi-Tier Cellular Networks With Deep Learning for 6G Consumer Electronics Communications
IEEE Transactions on Consumer Electronics(2024)
摘要
The imminent arrival of 6th Generation (6G) consumer electronics wireless networks heralds a paradigm shift in communication necessitated by diverse quality-of-service (QoS) demands. Meeting these demands efficiently requires novel strategies, particularly within multi-tier cellular networks encompassing femto cells, pico cells, macro cells, and device-to-device (D2D) links. However, managing channel assignments and computational loads in such underlay networks poses challenges. Additionally, due to the inherent randomness, selecting a communication mode between cellular and D2D and associating users with specific access points are complex tasks. Leveraging advancements in artificial intelligence (AI), we propose novel Deep Learning (DL) techniques for joint mode selection, user association, and channel assignment (MUC) in C-RAN (multi-Tier). Using deep neural networks (DNNs), our approach streamlines decision-making without exhaustive computations. To train the DNN model, we introduce an efficient Individual Channel Allocation (ICA) algorithm, a path-loss-based user-association method, and a heuristic mode selection technique collectively named JMUC. These techniques are applied in a random environment for a large number of times, and each time, their outputs are added to a set of samples, which is then used to train our DNN model using a supervised DL technique. Simulation outcomes demonstrate the effectiveness of the presented DL-based JMUC approach.
更多查看译文
关键词
6G,Deep Learning,Channel Allocation,joint Mode-selection,User-Association,Channel-Assignment
AI 理解论文
溯源树
样例
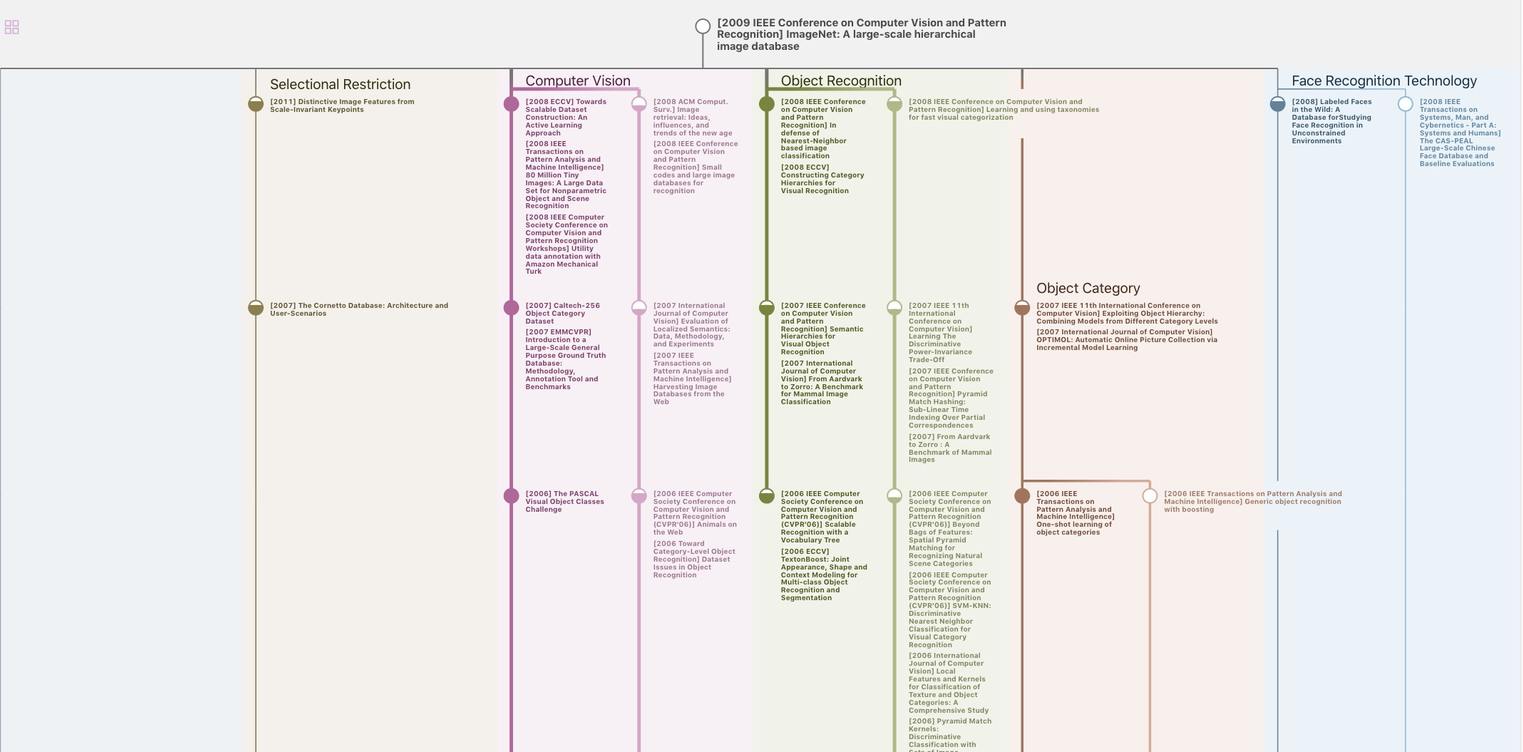
生成溯源树,研究论文发展脉络
Chat Paper
正在生成论文摘要