Lightweight Fault Prediction Method for Edge Networks
IEEE INTERNET OF THINGS JOURNAL(2024)
摘要
The occurrence of faults increases in edge networks as the service types and component architecture become increasingly complex. Traditional centralized cloud fault prediction technology cannot be directly applied to edge network systems that are typically required to handle real-time data due to their high-computational complexity. Therefore, this article proposes a lightweight fault prediction algorithm for edge networks that realizes fault prediction with cross-layer cooperation. First, for edge devices with limited computation resources, the time feature lightweight extraction method of brain neurology fusion long short term memory (LSTM) based on scene reappearance mechanisms is proposed, which solves the single-step dependency problem of neurons and improves the accuracy. And the LSTM neuron connection method based on pulse dynamics is designed. This method prunes the structure of the LSTM network based on relevant knowledge of biological neurology to realize a lightweight time feature extraction model of fault information. Then, on the edge server side, a spatial feature lightweight extraction method based on a two-way residual structure is proposed. This method uses decomposition convolution to reduce the number of network parameters and realize a lightweight model. Finally, the spatio-temporal correlation features of the extracted fault information are spliced to realize fault prediction. To verify the effectiveness of the proposed model, we compare the improved model with the existing fault prediction model. The experiments show that the algorithm proposed in this article has higher accuracy, lower complexity and lower memory requirements. Therefore, it has high-deployment potential in edge network scenarios.
更多查看译文
关键词
Feature extraction,Servers,Predictive models,Prediction algorithms,Telecommunications,Probability,Neurons,Edge networks,fault prediction,feature extraction,spatio-temporal correlation
AI 理解论文
溯源树
样例
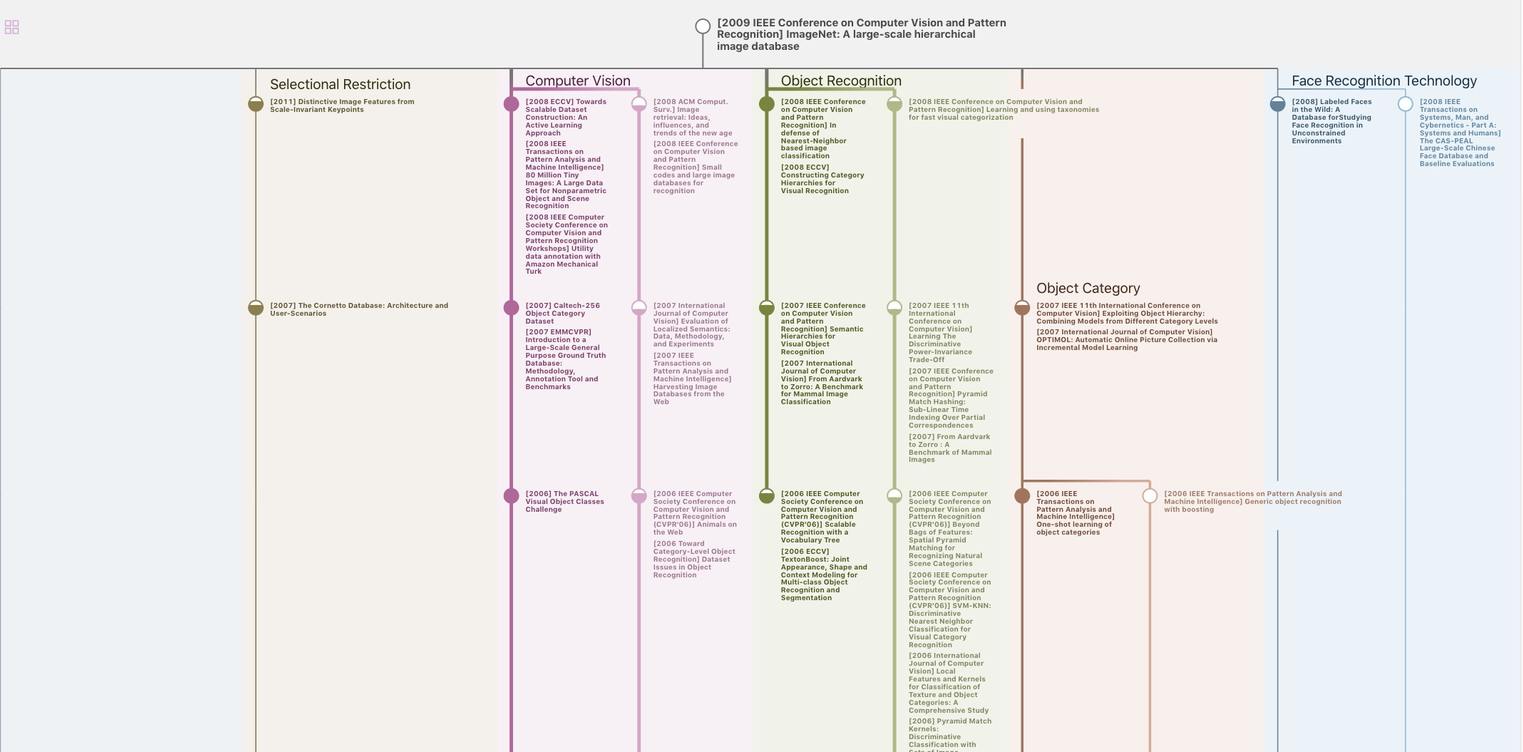
生成溯源树,研究论文发展脉络
Chat Paper
正在生成论文摘要