Cooperative Deep Reinforcement Learning Enabled Power Allocation for Packet Duplication URLLC in Multi-Connectivity Vehicular Networks
IEEE Transactions on Mobile Computing(2024)
Abstract
Ultra reliable low latency communication (URLLC) in vehicular networks is crucial for safety-related vehicular applications. Mini-slot with a short packet that carries only a few symbols is used to reduce the transmission time interval and enable quick scheduling for URLLC that requires extremely low latency. However, a single air interface transmission of URLLC packets may fail due to the high mobility of vehicles. Leveraging multi-connectivity technologies, the real-time reliability of URLLC can be greatly enhanced without relying on packet retransmission. In this paper, we propose a multi-connectivity URLLC downlink transmission scheme for vehicular networks, where the URLLC packet is duplicated and transmitted over multiple independent wireless links to improve packet reliability. Specifically, we design a multi-agent cooperative deep reinforcement learning algorithm, called transformer associated proximal policy optimization (TAPPO), to achieve real-time robust power allocation for multi-connectivity URLLC with imperfect channel state information (CSI). The transformer neural network architecture is employed to share the information among multiple links serving the same URLLC user and choose appropriate transmit powers, enabling cooperation to ensure reliability while minimizing inter-cell interference and energy consumption. Extensive simulation results validate the effectiveness of multi-connectivity packet duplication for URLLC and proposed TAPPO for power allocation.
MoreTranslated text
Key words
URLLC,multi-connectivity,vehicular networks,deep reinforcement learning,transformer
AI Read Science
Must-Reading Tree
Example
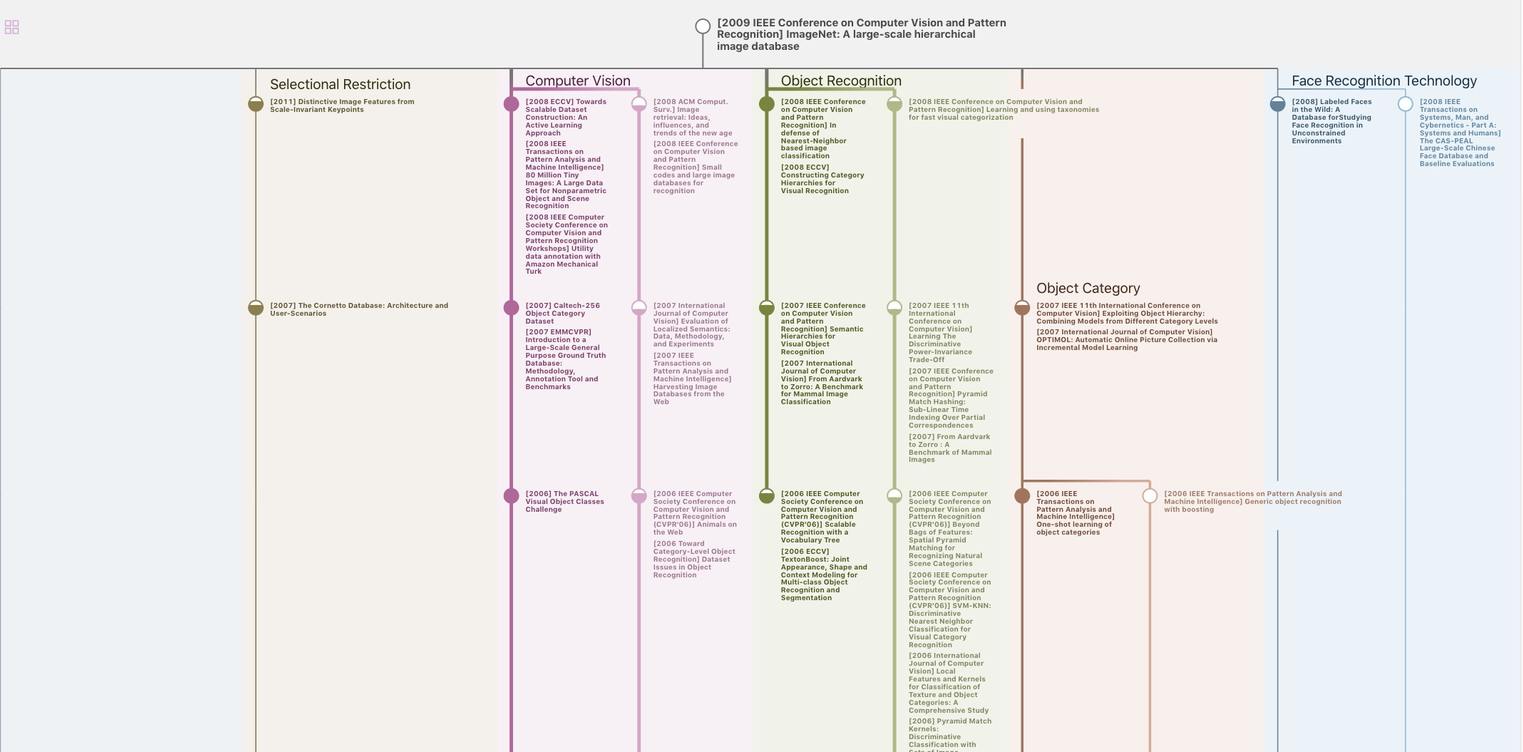
Generate MRT to find the research sequence of this paper
Chat Paper
Summary is being generated by the instructions you defined