Robust Gaze Point Estimation for Metaverse With Common Mode Features Suppression Network
IEEE Transactions on Consumer Electronics(2024)
摘要
Gaze point estimation is an essential technology in extended reality devices, and plays a vital role in the metaverse with consumer health. A challenging issue is the great variation underlying the gaze-related encode information, which makes current methods difficult to generate gaze point with stable quality among different persons. To this end, we propose a robust framework to generate high-accuracy gaze point from appearance. Specifically, eyes and face regions are fused into network to enrich high-level features. Then, we develop a common mode features suppression network (CMFS-Net) to predict the gaze bias between the input image and standard image. It is based on the differential amplifier. In addition, a Point-Mean algorithm is designed to generate the estimated gaze point from candidate points. The performance of the CMFS-Net is evaluated on three gaze estimation datasets, GazePC, GazeCapture, and Rice TabletGaze. Among them, the GazePC is collected by ourselves which composes 41.25K images from 165 participants. Experimental results demonstrate that our model is effective and robust for appearance-based gaze point estimation, and has advantages over peer methods.
更多查看译文
关键词
Metaverse,Consumer Health,Gaze Point Estimation,Deep Learning,Features Suppression
AI 理解论文
溯源树
样例
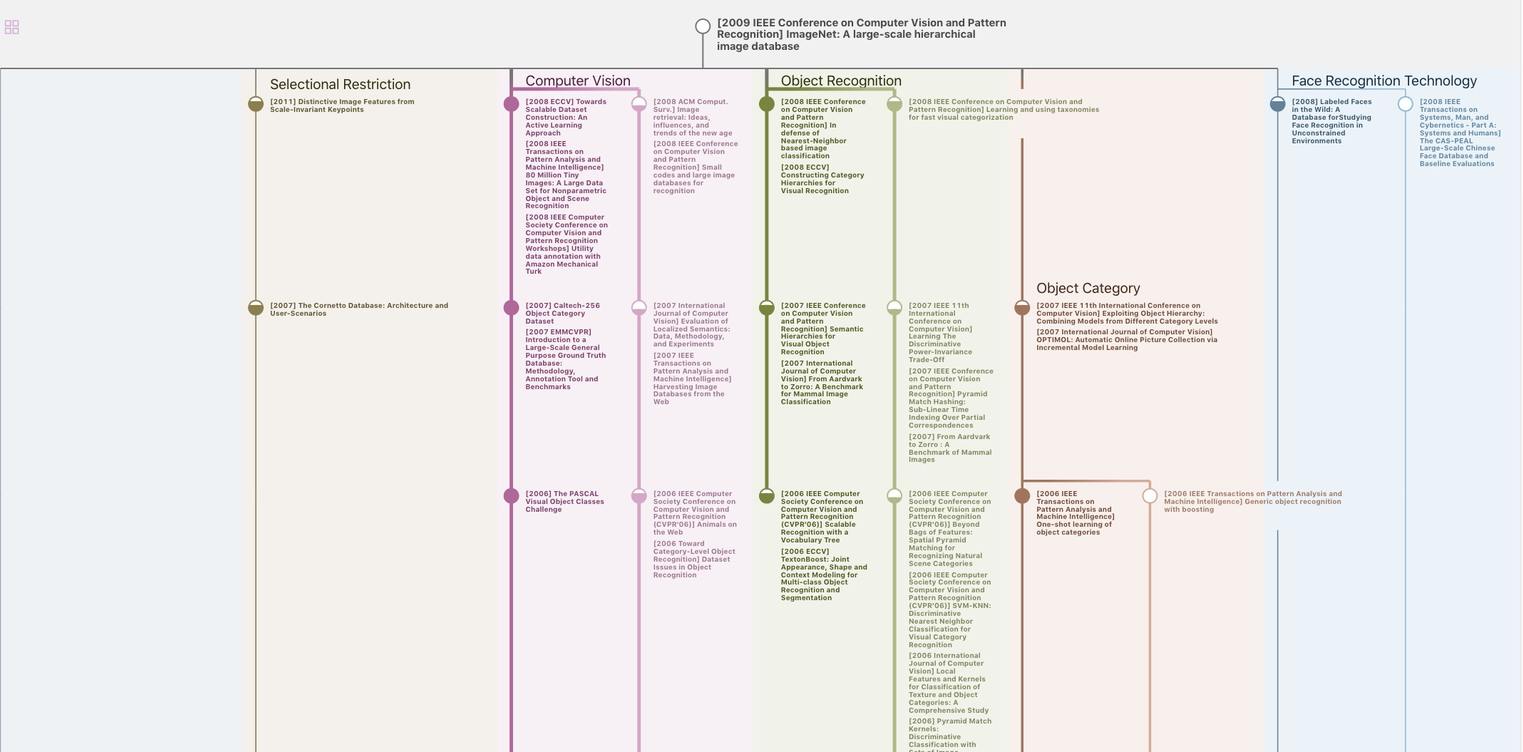
生成溯源树,研究论文发展脉络
Chat Paper
正在生成论文摘要