Sustainable Scheduling of Distributed Flow Shop Group: A Collaborative Multi-Objective Evolutionary Algorithm Driven by Indicators
IEEE Transactions on Evolutionary Computation(2023)
摘要
Sustainable scheduling within the manufacturing field has garnered substantial attention from both academia and industry. The escalating market demands have heightened requirements on the flexibility of production modes, multi-zone, and multi-objective. In this context, our study explores the intricacies of the multi-objective distributed flow shop group scheduling problem with sequence-dependent setup times, aiming to concurrently optimize makespan and total energy consumption (DFm|group, sdst|#(Cmax, TEC) ). Firstly, a mathematical model is constructed to analyze problem characteristics. Subsequently, we introduce a collaborative multi-objective evolutionary algorithm driven by indicators (CMOEA/I). In CMOEA/I, an indicator-driven approach is proposed for solution selection, which approximates the Pareto front based on the convergence indicator, while screening potential solutions based on the spread indicator. Furthermore, a collaborative model and local search are developed by incorporating the intrinsic linkages of factories, groups, and jobs. Additionally, to further explore the potential non-dominated solutions, a speed variation strategy is devised based on the pivots of decreasing speed to save energy and increasing speed to reduce makespan. An extensive set of simulation experiments is conducted on a diverse range of test instances. Through meticulous statistical analysis, the outcomes demonstrate that the CMOEA/I exhibits efficacy when contrasted with other advanced algorithms.
更多查看译文
关键词
Sustainable,group scheduling,distributed flow shop,multi-objective optimization,performance indicator
AI 理解论文
溯源树
样例
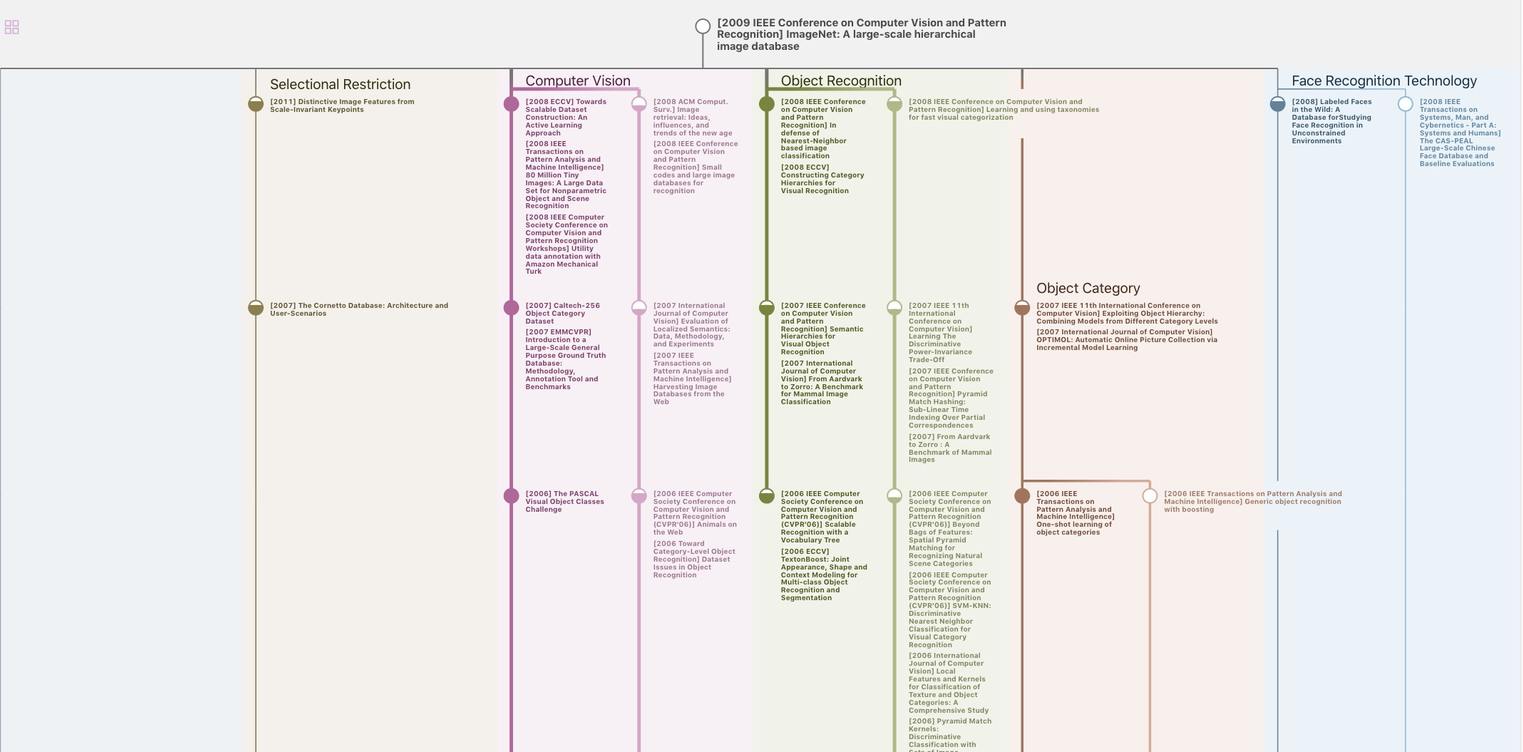
生成溯源树,研究论文发展脉络
Chat Paper
正在生成论文摘要