Boosting Semi-Supervised Object Detection in Remote Sensing Images With Active Teaching
IEEE GEOSCIENCE AND REMOTE SENSING LETTERS(2024)
摘要
The lack of object-level annotations poses a significant challenge for object detection in remote sensing images (RSIs). To address this issue, active learning (AL) and semi-supervised learning (SSL) techniques have been proposed to enhance the quality and quantity of annotations. AL focuses on selecting the most informative samples for annotation, while SSL leverages the knowledge from unlabeled samples. In this letter, we propose a novel AL method to boost semi-supervised object detection (SSOD) for remote sensing images with a teacher-student network, called SSOD-AT. The proposed method incorporates an RoI comparison module (RoICM) to generate high-confidence pseudo-labels for regions of interest (RoIs). Meanwhile, the RoICM is utilized to identify the top-K uncertain images. To reduce redundancy in the top-K uncertain images for human labeling, a diversity criterion is introduced based on object-level prototypes of different categories using both labeled and pseudo-labeled images. Extensive experiments on DOTA and DIOR, two popular datasets, demonstrate that our proposed method outperforms state-of-the-art methods for object detection in RSIs. Compared with the best performance in the SOTA methods, the proposed method achieves 1% improvement in most cases in the whole AL.
更多查看译文
关键词
Prototypes,Remote sensing,Object detection,Uncertainty,Labeling,Training,Annotations,Active learning (AL),remote sensing,semisupervised object detection (SSOD),teacher-student framework
AI 理解论文
溯源树
样例
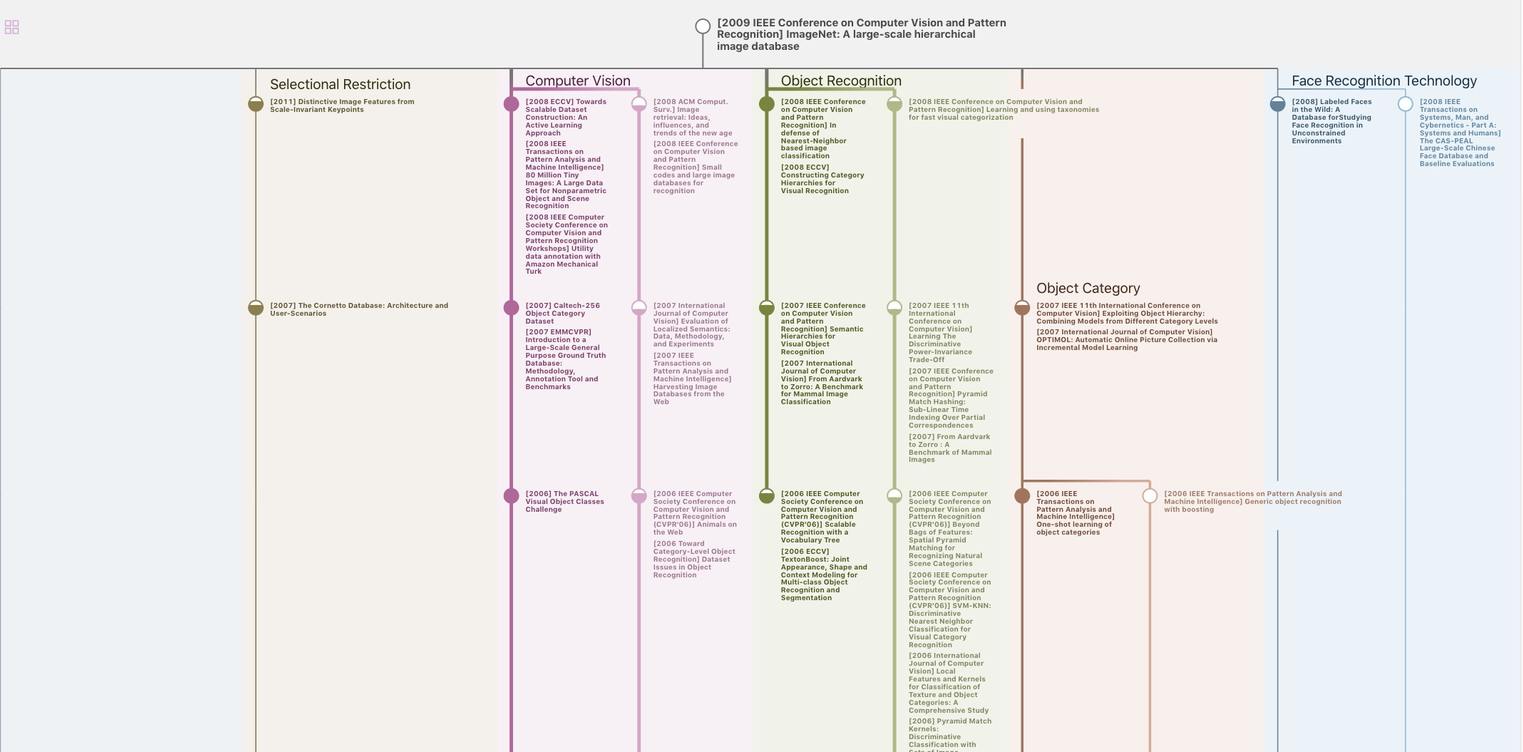
生成溯源树,研究论文发展脉络
Chat Paper
正在生成论文摘要