Quantitative Diagnosis of Internal Short Circuit for Lithium-Ion Batteries Using Relaxation Voltage
IEEE TRANSACTIONS ON INDUSTRIAL ELECTRONICS(2024)
摘要
Internal short circuit (ISC) fault can significantly degrade a lithium-ion battery's lifetime, and in severe cases can lead to fatal safety accidents. Therefore, it is critical to diagnose the ISC fault in its early stage for preventing early ISC from evolving into serious safety accidents. In this article, we develop a purely data-driven method using machine learning algorithms for diagnosing the early ISC fault based on different relaxation voltage features. The 15, 30, 45, and 60-min relaxation voltage features are extracted and preliminarily selected to train and verify the Gaussian process regression (GPR) model. Furthermore, the particle swarm optimization algorithm is used to optimize the input features for improving the accuracy of the ISC diagnosis. Three typical data-driven approaches including back propagation neural network, long short-term memory network, and support vector regression are applied to compare the model performance with the GPR model. The results show that the GPR model performs better than the other three models, whose diagnostic error is less than 6.45%.
更多查看译文
关键词
Fault diagnosis,internal short circuit (ISC),lithium-ion batteries,machine learning
AI 理解论文
溯源树
样例
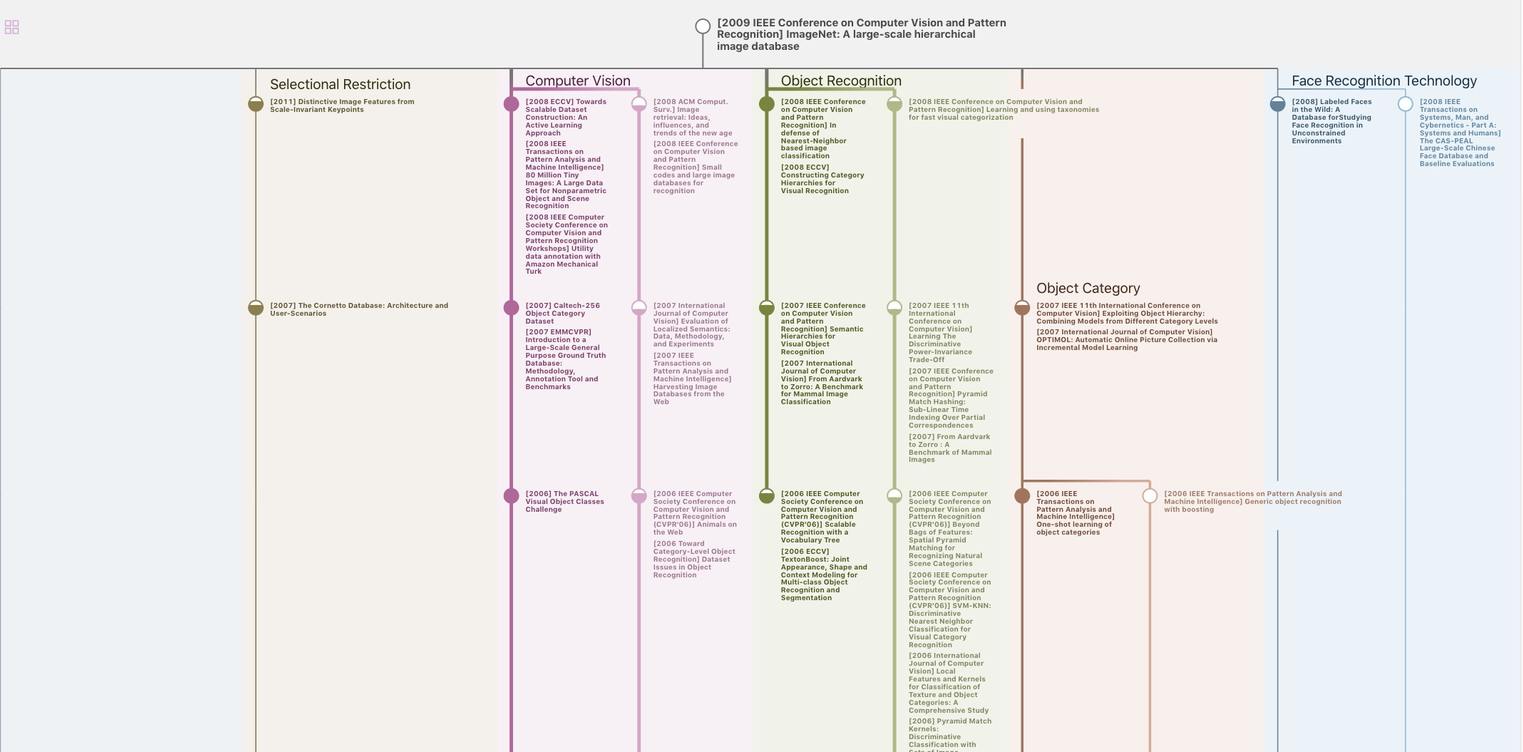
生成溯源树,研究论文发展脉络
Chat Paper
正在生成论文摘要