State of Health Estimation for Second-Life Lithium-Ion Batteries in Energy Storage System With Partial Charging-Discharging Workloads
IEEE TRANSACTIONS ON INDUSTRIAL ELECTRONICS(2024)
摘要
Echelon utilization in energy storage systems (ESSs) has emerged as one of the predominant solutions for addressing large-scale retired lithium-ion batteries from electrical vehicles. However, high unit-to-unit health variability and partial charging-discharging workloads render the state of health (SOH) estimation of these second-life lithium-ion batteries (SL-LIBs) in ESSs a crucial and challenging issue. Existing SOH estimation methods are commonly focused on new batteries with consistent health state and complete charging-discharging workloads, while the estimation methods for SL-LIBs have been rarely developed. To fill this gap, this article proposes a novel SOH estimation method with specially designed features and calibrated uncertainty quantification for SL-LIBs. Joint features are first introduced for extracting useful SOH information from the cycling data of SL-LIBs under partial charging-discharging workloads. Then, Bayesian neural network with uncertainty calibration is used to generate SOH estimation results, which can quantify the estimation uncertainty caused by unit-to-unit health variability. To demonstrate the proposed method, a total of 36 retired NCM-18650 power batteries are cycled under 9 different partial charging-discharging workloads. A case study on this SL-LIB lab-test dataset reaches the best results of 2.13% mean absolute percentage error and 0.0178 root-mean-squared error, as well as well-calibrated estimation uncertainty.
更多查看译文
关键词
Bayesian neural network,echelon utilization,energy storage system,second-life lithium-ion batteries,state of health
AI 理解论文
溯源树
样例
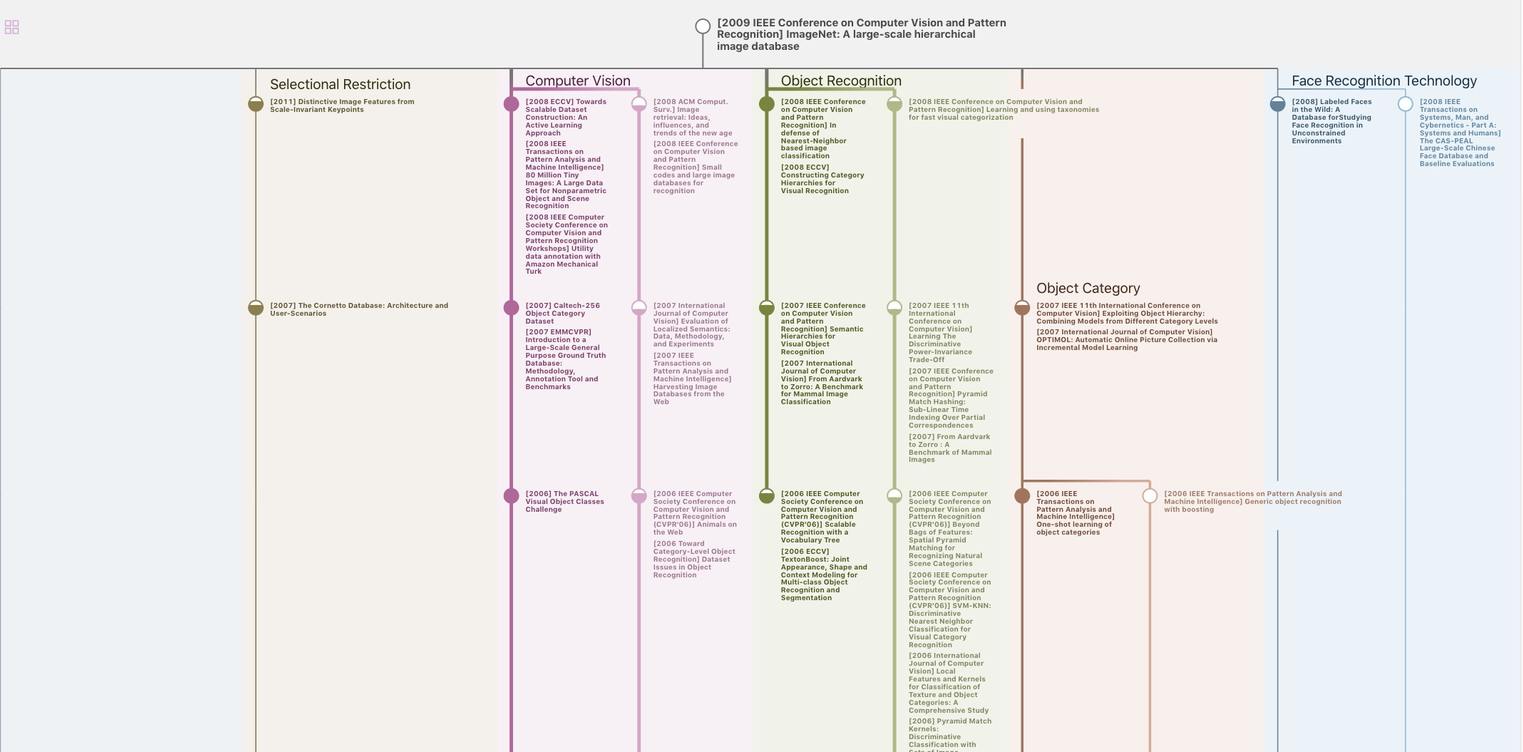
生成溯源树,研究论文发展脉络
Chat Paper
正在生成论文摘要