PDEs Discovery for Soft Sensors Using Coupled Physics-Informed Neural Network With Akaike’s Information Criterion
IEEE Transactions on Circuits and Systems II: Express Briefs(2023)
摘要
Partial differential equations (PDEs) are the model candidates for the soft sensors in industrial processes with spatiotemporal dependence. However, the gaps often exist between idealized PDEs and practical situations. Discovering proper PDE structures can remedy the gaps. To this end, a coupled physics-informed neural network with Akaike’s information criterion (CPINN-AIC) is proposed to discover proper PDE structures for soft sensors. First, CPINN is trained through the hierarchical training strategy for approximating the solutions and source terms satisfying PDEs. Consequently, AIC is used to select the proper combination of differential operators. Finally, the feasibility and effectiveness of the proposed CPINN-AIC for soft sensors are verified using an artificial dataset obtained from simulation with heat equation and a practical vibration dataset from our wear experiment platform compared with some state-of-the-art methods. The proposed CPINN-AIC can be used as a data-driven method to discover proper PDE structures and concurrently approximate solutions satisfying PDEs, which rescues the conditions of inexactly governing equation structures.
更多查看译文
关键词
Akaike’s information criterion,coupled physics-informed neural network,hierarchical training strategy,partial differential equations with unknown structures,soft sensors
AI 理解论文
溯源树
样例
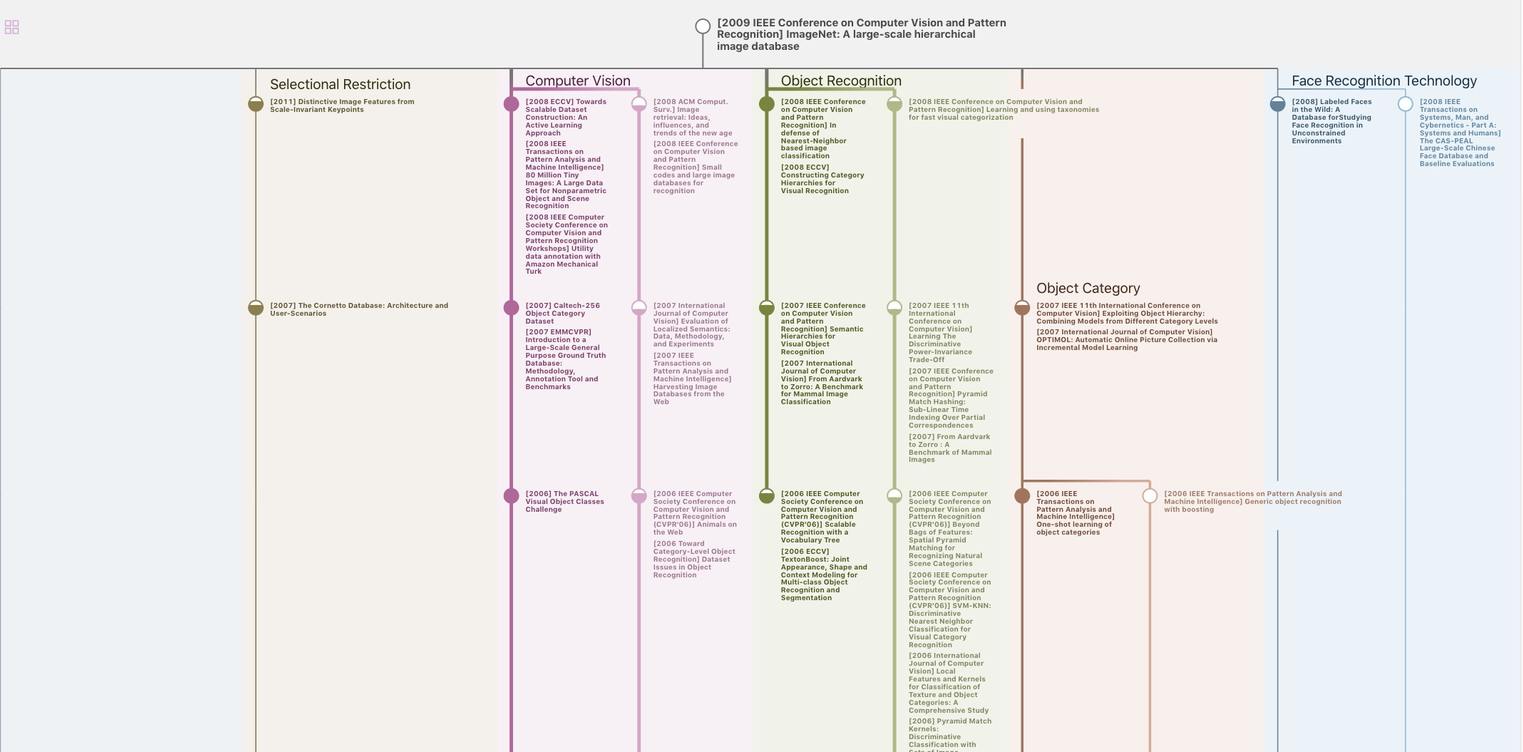
生成溯源树,研究论文发展脉络
Chat Paper
正在生成论文摘要