Iterative Q-Learning for Model-Free Optimal Control With Adjustable Convergence Rate
IEEE TRANSACTIONS ON CIRCUITS AND SYSTEMS II-EXPRESS BRIEFS(2024)
摘要
In this brief, a novel accelerated Q-learning algorithm is developed to address optimal control problems for discrete-time nonlinear systems. First, the accelerated Q-learning scheme is proposed by introducing the relaxation factor. Note that the relaxation factor leads to the adjustability of the convergence rate. Second, the convergence of the Q-function is analyzed with different relaxation factors. Third, the adjustable Q-learning scheme is developed with guaranteed convergence, which can adaptively change the value of the relaxation factor. Finally, the simulation results demonstrate the effectiveness of this proposed algorithm.
更多查看译文
关键词
Convergence,Q-learning,Optimal control,Iterative methods,Adaptation models,Heuristic algorithms,Power system dynamics,Adaptive dynamic programming,fast convergence rate,nonlinear systems,optimal control
AI 理解论文
溯源树
样例
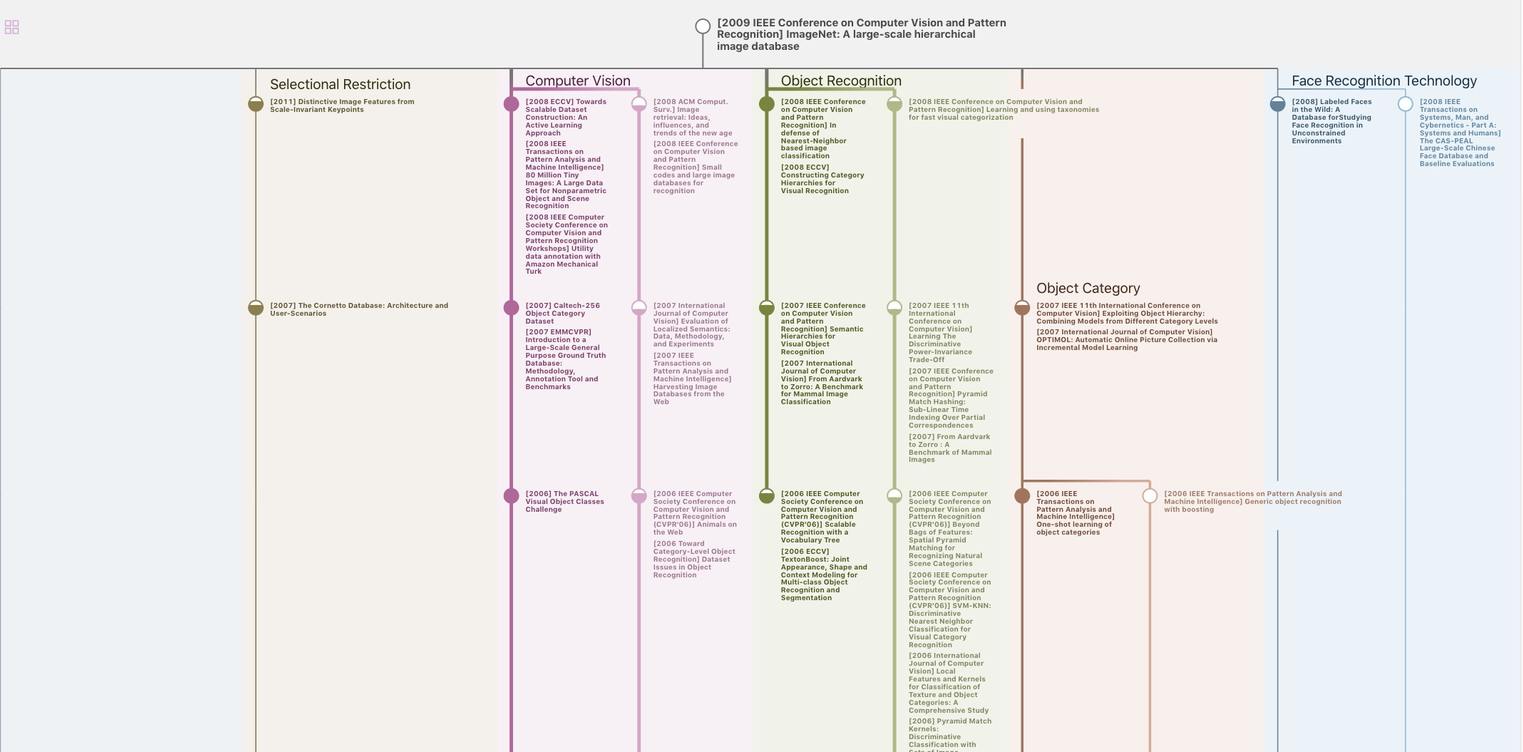
生成溯源树,研究论文发展脉络
Chat Paper
正在生成论文摘要