Attention-Based Interval Aided Networks for Data Modeling of Heterogeneous Sampling Sequences with Missing Values in Process Industry.
IEEE transactions on industrial informatics(2024)
摘要
In complex process industries, multivariate time sequences are omnipresent, whose nonlinearities and dynamics present two major challenges for soft sensing of important quality variables. Consequently, due to the potent representational capabilities, nonlinear dynamic models like gated recurrent unit (GRU) and long short-term memory (LSTM) networks have been used for data sequence modeling. Though it is a common occurrence in many industrial plants, data series with heterogeneous sample intervals and missing values cannot be directly handled by these dynamic algorithms. To this end, attention-based interval-aided networks (AIA-Net) are proposed in this article to adaptively model the temporal information for heterogeneous sampling sequences with missing values in the processes industry. It includes two main mechanisms, which are named attention-based time-aware dynamic imputation and interval-aided time-aware network, respectively. The reduction rate is introduced by the attention-based time-aware dynamic imputation to apply the effects of time intervals and is used in the imputation of missing data. The interval-aided time-aware network includes time intervals in the model structure and uses a sampling interval gate to correct the temporal correlations in time series. The proposed AIA-Net is successfully applied to a real hydrocracking process to predict the C5 and C6 content in the light naphtha.
更多查看译文
关键词
Logic gates,Data models,Time series analysis,Predictive models,Mathematical models,Industries,Soft sensors,Gated recurrent unit (GRU),heterogeneous sampling,long short-term memory (LSTM),missing values,prediction,soft sensor,time interval
AI 理解论文
溯源树
样例
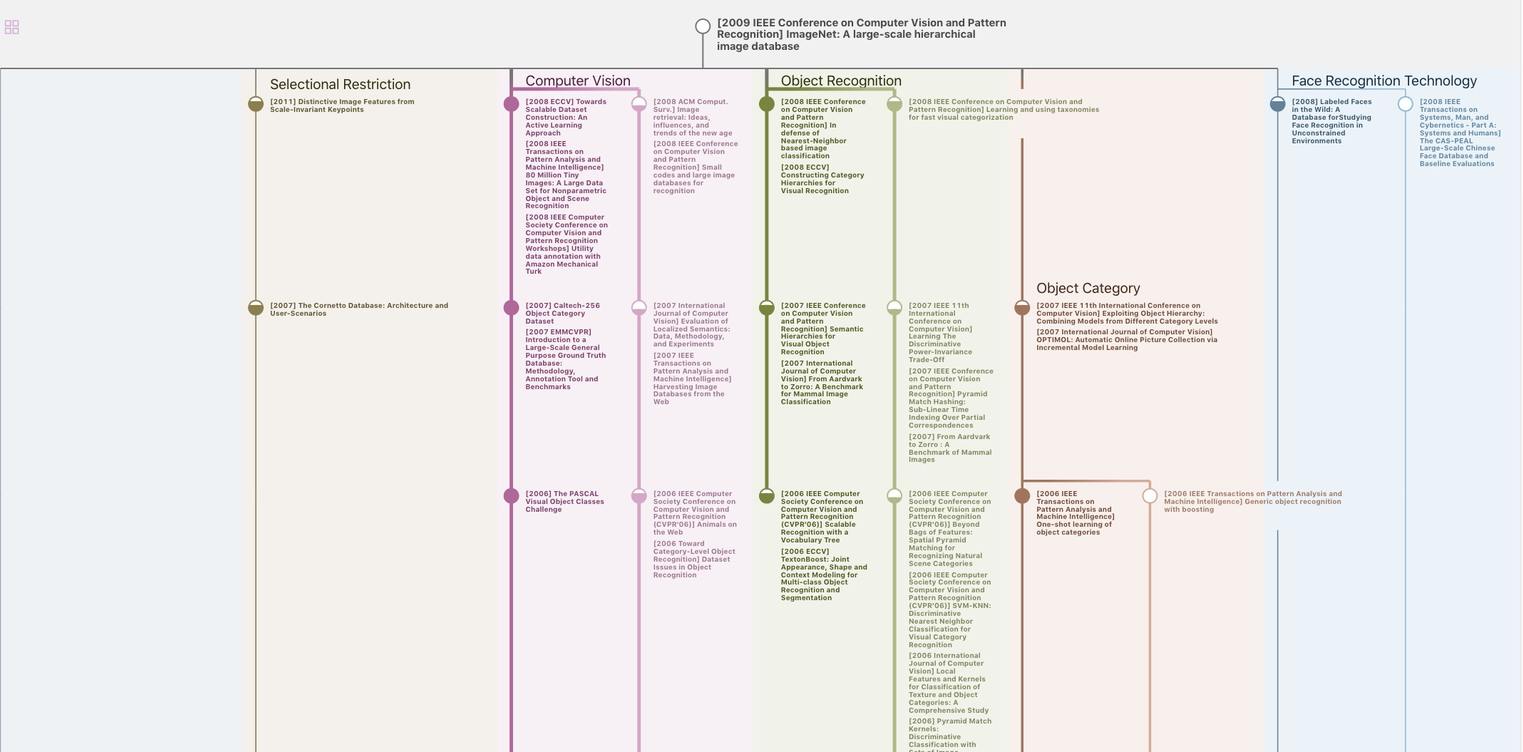
生成溯源树,研究论文发展脉络
Chat Paper
正在生成论文摘要