Deep Learning Based Vehicle-Mounted Environmental Context Awareness via GNSS Signal
IEEE TRANSACTIONS ON INTELLIGENT TRANSPORTATION SYSTEMS(2024)
摘要
High-precision GNSS positioning plays a crucial role in intelligent transportation systems, and leveraging artificial intelligence can enable the development of reliable context-aware models that enhance context-adaptive GNSS algorithms. However, the complexity of contexts and the variability of features limit the performance of GNSS-based context-aware models. Therefore, this study aims to construct a context-aware model in kinematic vehicle-mounted environments. Firstly, due to the lack of publicly available datasets, a kinematic vehicle-mounted dataset comprising over 40,000 data samples is constructed. This dataset includes five contexts including open area, urban canyon, boulevard, under viaduct, and tunnel. Meanwhile, efficient feature selection methods are applied, namely SHAP (Shapley Additive exPlanations) and SFFS (Sequential Forward Floating Selection), to obtain effective data features. Secondly, considering the temporal correlation of GNSS signals, eight alternative models (SVM, MLP, CNN-b, CNN-r, CNN-i, ML-LSTM, ML-RNN, ML-GRU) are designed and trained. Context probability is introduced to indicate context transition area. Comparative studies show that the ML-LSTM model exhibits the best performance, with an an accuracy of 92.72% and a mean average precision (mAP) of 92.94% in context-separated areas, and with an accuracy of 87.49% and a mAP of 89.21% in context-continuous areas. Furthermore, to address the performance degradation when deploying the model on different receiver types, this study explores model-based transfer learning. Four transfer approaches, namely direct transfer, fully connected layer transfer, partial layer transfer, and all layer transfer, are applied. The all layer transfer approach demonstrates the best performance, exhibiting a 18.34% improvement compared to the non-transfer approach.
更多查看译文
关键词
GNSS context awareness,vehicle navigation,machine learning,transfer learning
AI 理解论文
溯源树
样例
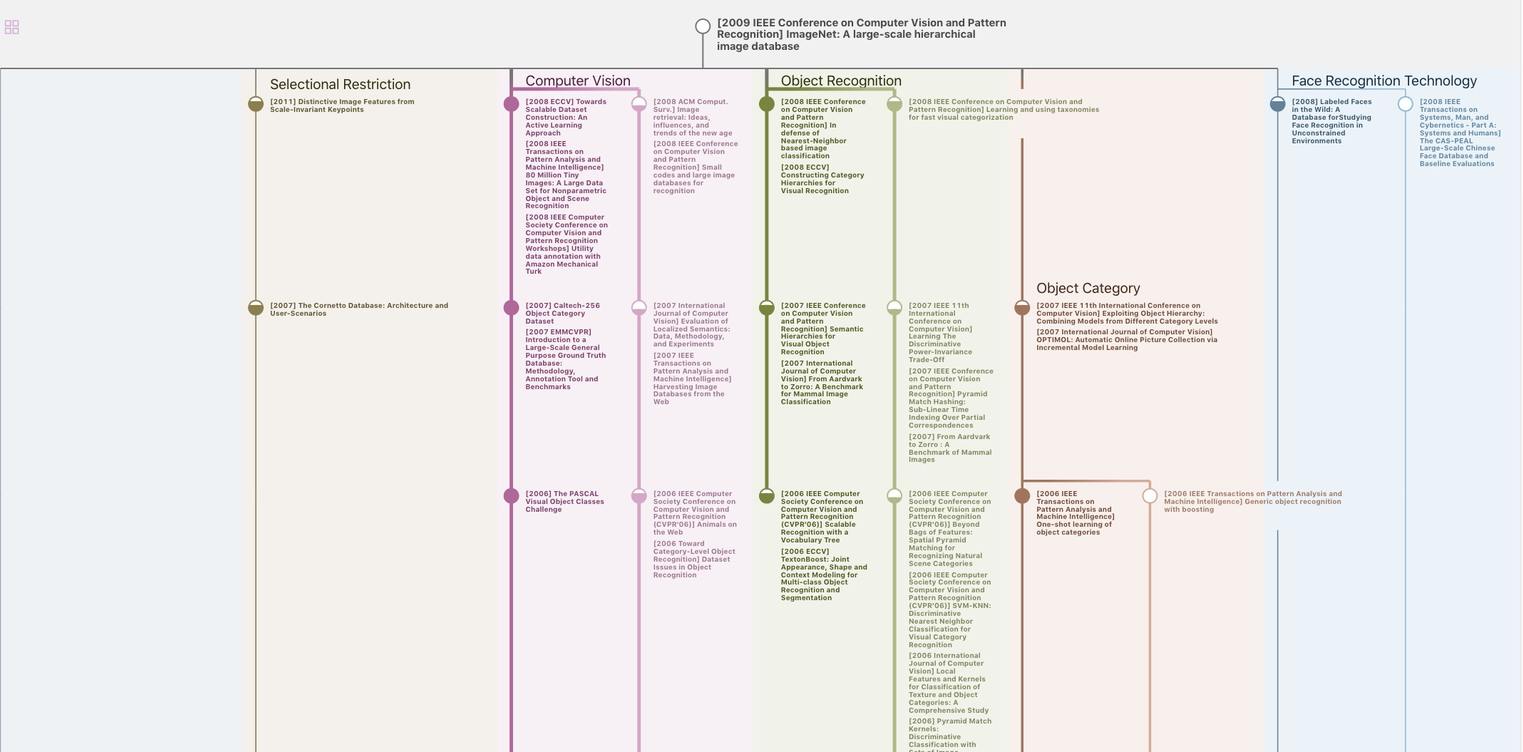
生成溯源树,研究论文发展脉络
Chat Paper
正在生成论文摘要