Learning Dynamical Position Embedding for Discriminative Segmentation Tracking
IEEE Transactions on Intelligent Transportation Systems(2024)
摘要
Visual tracking plays a pivotal role in intelligent transportation systems and has a wide range of practical applications such as autonomous driving and traffic counting. Recently, the attention mechanism in Transformers has been successfully applied to the field of visual tracking, leading to a significant improvement in tracking performance. However, Transformer-based trackers directly flatten two-dimensional image features into one-dimensional vectors to compute attention scores. This process unavoidably results in the omission of crucial position distribution information necessary for precise target localization. To address this issue, we propose a novel cross-attention based tracking-by-segmentation framework, called Dynamical Position Embedding based Tracking framework (DPET). DPET incorporates an additional network for modeling position information to complement the cross-attention module. To be specific, a dynamical position embedding network is introduced to adaptively encode position information. This network is then integrated into the cross-attention based feature fusion network to compensate for the loss of position distribution information. As a result, the fused feature incorporates abundant contextual semantic cues for target classification and precise position information for target localization simultaneously. To overcome the constraints imposed by bounding-boxes, a segmentation network that takes the fused feature as input is designed to achieve accurate pixel-wise tracking. Extensive experiments on eight challenging tracking benchmarks show that our DPET tracker enables real-time operations and achieves promising tracking performance on the GOT-10K benchmark. Especially, DPET tracker achieves the top accuracy scores on VOT2016, VOT2018 and VOT2019 benchmarks.
更多查看译文
关键词
Visual object tracking,attention mechanism,tracking-by-segmentation,dynamical position embedding
AI 理解论文
溯源树
样例
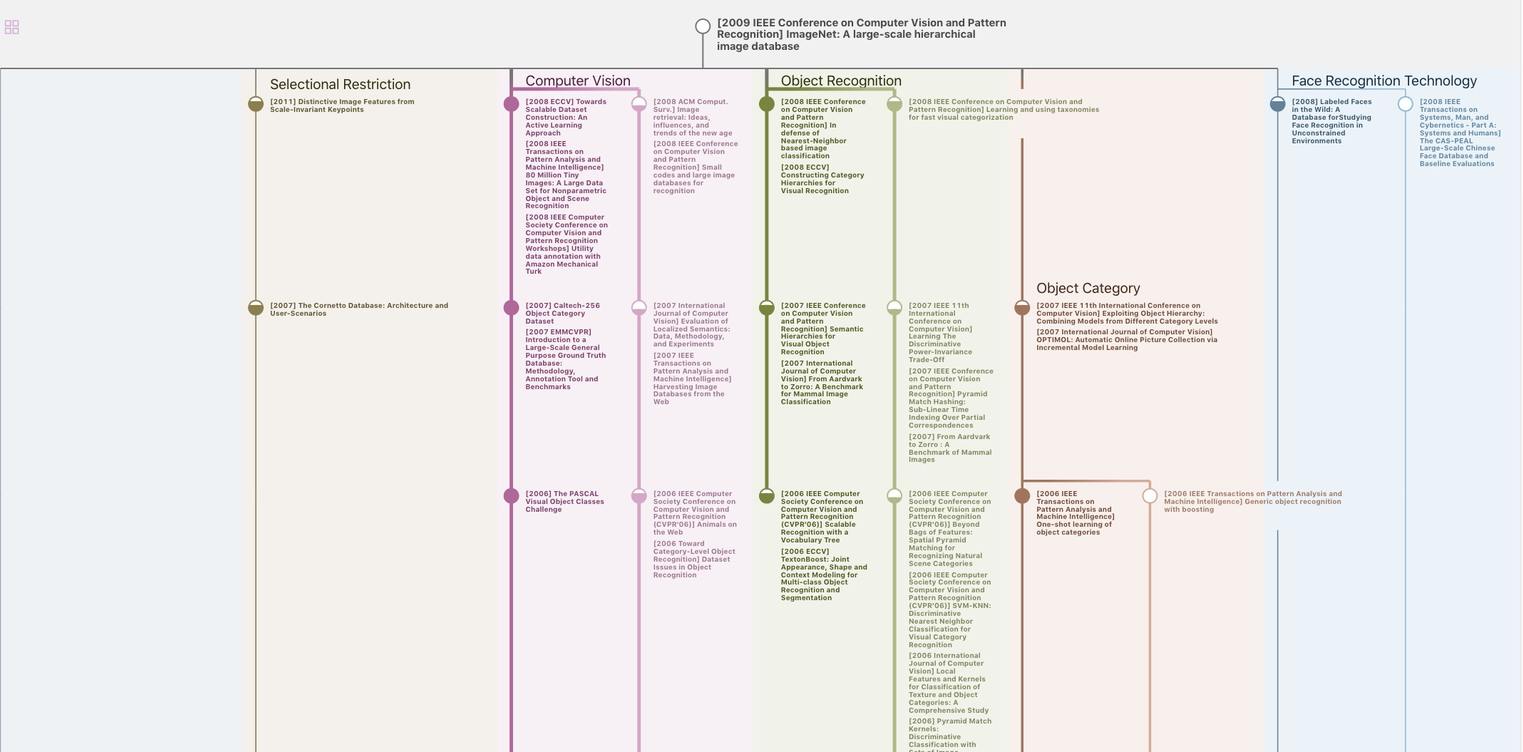
生成溯源树,研究论文发展脉络
Chat Paper
正在生成论文摘要