A Multivariate Normal Distribution Data Generative Model in Small-Sample-Based Fault Diagnosis: Taking Traction Circuit Breaker as an Example
IEEE TRANSACTIONS ON INTELLIGENT TRANSPORTATION SYSTEMS(2024)
摘要
Data-driven approaches have been widely used in the field of traction system and equipment fault diagnosis. However, limited training samples can cause data-driven models to face the dilemma of overfitting. In order to supplement sufficient training data in small-sample case, this paper proposes a data generative model based on the Multivariate Normal (MVN) distribution and Mahalanobis Distance (MD). The basic hypothesis of the method is that the diagnostic feature vectors representing the same fault state are subject to an identical MVN distribution. Afterward, its probability density function is unbiasedly estimated by the sample mean vector and sample covariance matrix, and then used to generate samples. During generation, the noise contained in the generated data is limited by the relationship between MD and Chi-square distribution. Finally, the generated samples are combined with original training samples to constitute a mix dataset to train data-driven fault diagnosis models. Taking the fault diagnosis of single-pole traction circuit breaker as an example, this paper illustrates the fault diagnosis framework with the proposed generative model and verifies its effectiveness. The results show that the generated samples cover the range of original samples well, thus increasing the prediction accuracy of the classifiers. Furthermore, three compared generative models are constructed. By comparison to these complicated models, the proposed method has better generation effect, although it limits the generative model capacity.
更多查看译文
关键词
Training,Fault diagnosis,Data models,Circuit faults,Feature extraction,Maximum likelihood estimation,Integrated circuit modeling,Small sample,generative model,fault diagnosis,circuit breakers
AI 理解论文
溯源树
样例
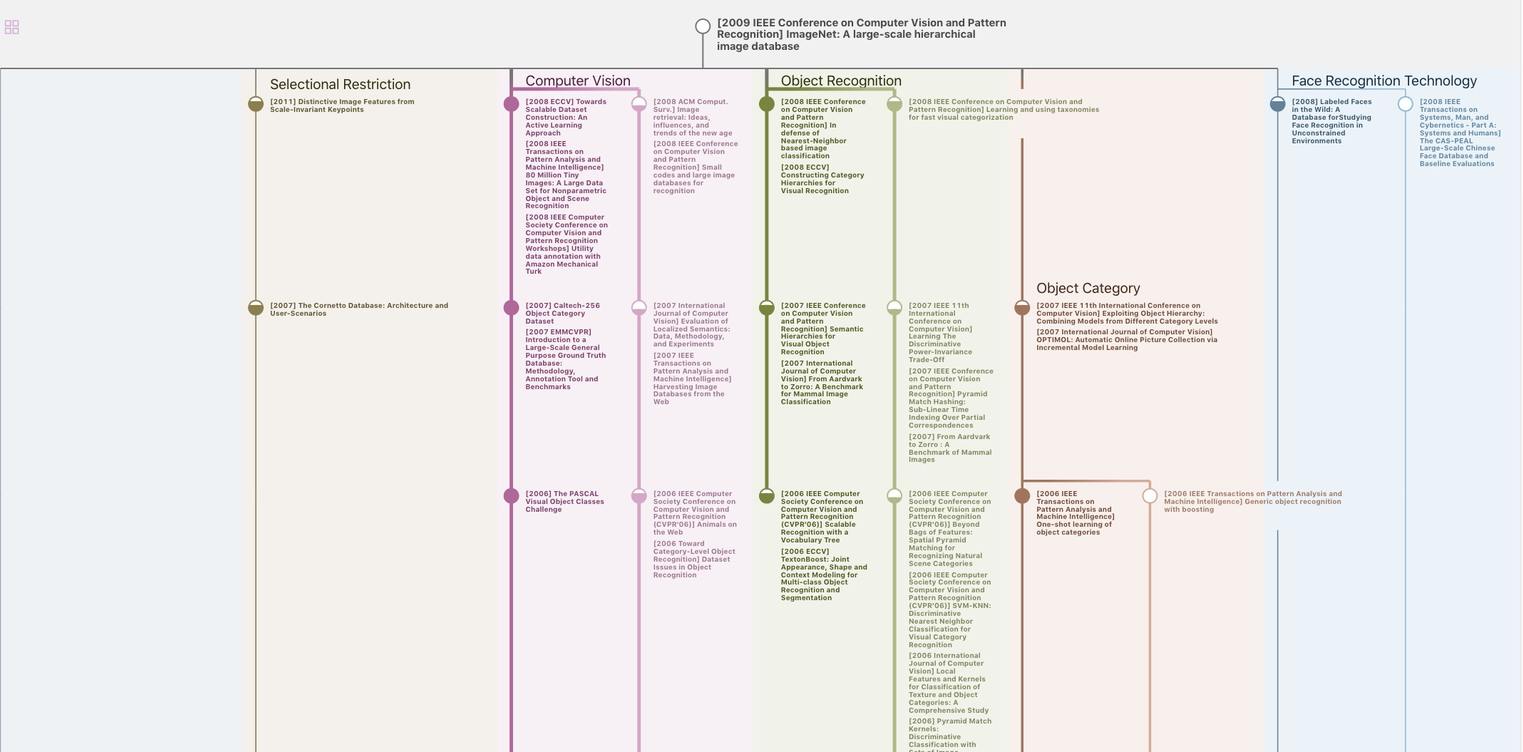
生成溯源树,研究论文发展脉络
Chat Paper
正在生成论文摘要