Enhancing Multi-UAV Reconnaissance and Search Through Double Critic DDPG with Belief Probability Maps
IEEE transactions on intelligent vehicles(2024)
Abstract
Unmanned Aerial Vehicles (UAVs) have recently attracted significant attention due to their potential applications in reconnaissance and search. This paper aims to investigate the issue of multi-UAV cooperative reconnaissance and search (MCRS) to ensure ample coverage of the mission area and precise localization of static targets. The MCRS problem is modeled as a multi-objective optimization problem, taking into account the credibility of search results. To achieve this, we design a belief probability map based on the Dempster-Shafer (DS) evidence theory, comprising an uncertainty map and two target maps. This representation enables a clear depiction of both the presence of the target and the uncertainty within the map. Subsequently, we reformulate this multi-objective optimization problem within the framework of Decentralized Partially Observable Markov Decision Process (Dec-POMDP). To address this reformulation, a new deep reinforcement learning approach called Double Critic Deep Deterministic Policy Gradient (DCDDPG) is proposed. Specifically, we introduce both a centralized critic and a local critic for each UAV agent to estimate the action-value function. This approach helps balance the bias in the action-value function estimation and the variance in the policy updates, thereby improving the coordination effect. Extensive simulation results demonstrate that DCDDPG outperforms existing techniques in terms of search efficiency and coverage.
MoreTranslated text
Key words
Autonomous aerial vehicles,Reconnaissance,Uncertainty,Reinforcement learning,Deep learning,Training,Search problems,Multi-UAV,reconnaissance and search,belief probability map,double critic deep deterministic policy gradient,bias and variance
AI Read Science
Must-Reading Tree
Example
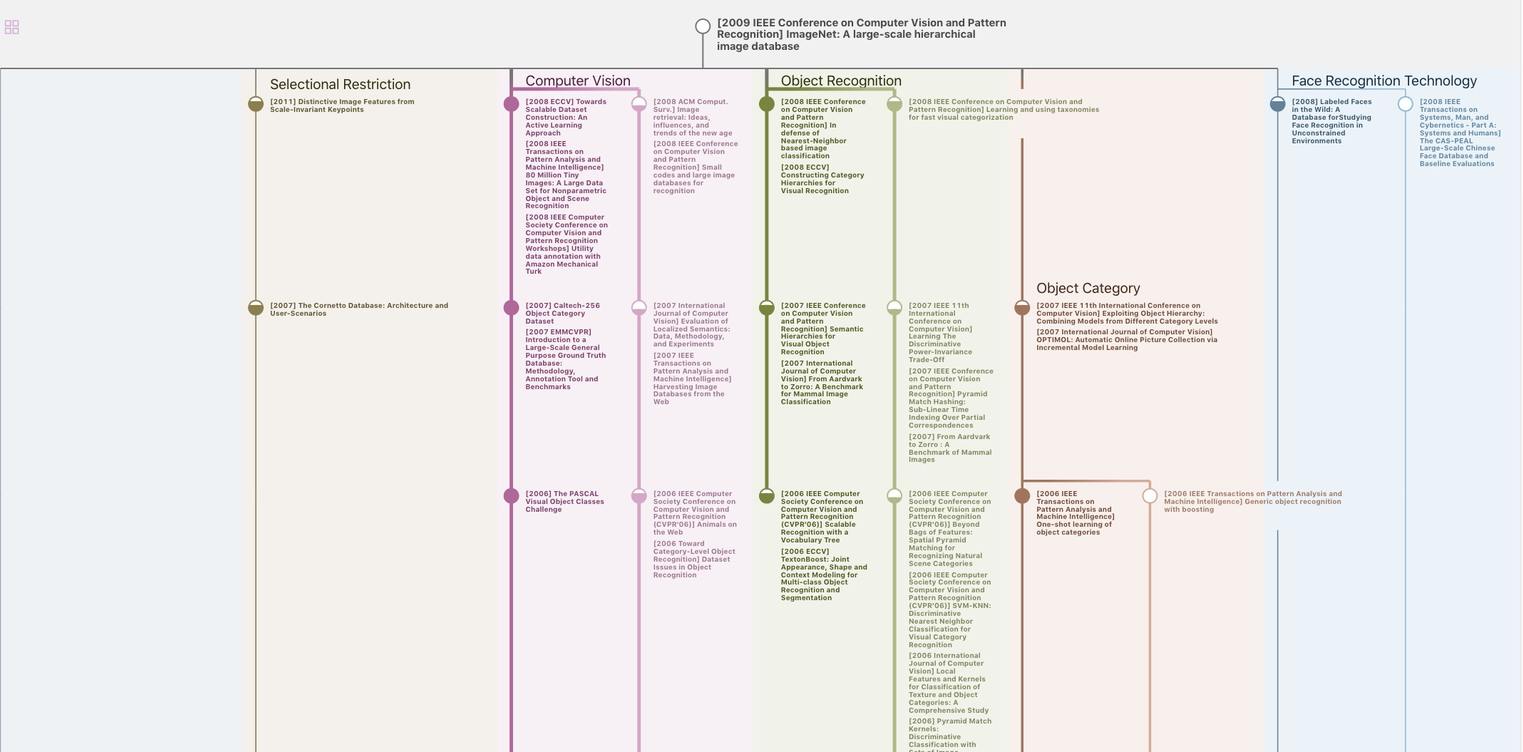
Generate MRT to find the research sequence of this paper
Chat Paper
Summary is being generated by the instructions you defined