Network-Wide Traffic Flow Dynamics Prediction Leveraging Macroscopic Traffic Flow Model and Deep Neural Networks
IEEE TRANSACTIONS ON INTELLIGENT TRANSPORTATION SYSTEMS(2024)
摘要
Obtaining future traffic state evolution information is critical to traffic control algorithms design and further to intelligent transportation systems. However, accurately predicting traffic state evolution is not an easy task, although the traffic prediction-related study attracted a lot of attention. This study develops a macroscopic traffic flow model-integrated deep learning framework ( MTFD ) for the high-resolution temporal-spatial traffic state dynamic propagation on a road network, integrating temporal-spatial traffic dependency, traffic flow theory, and data analysis techniques. First, traffic state propagation on every road section is mathematically described by the CTM model given traffic initial and boundary conditions. Next, a temporal-spatial traffic dependency attention ( TSTD ) recurrent neural network is developed to predict boundary conditions factoring the traffic temporal-spatial dependency. Also, this paper develops a graph theory-based method to capture the temporal-spatial traffic dependency among the traffic on neighboring road sections. Last, the extended Kalman Filter ( EKF ) is introduced to adjust the predicted traffic state at an intersection to satisfy the conservation law. The numerical experiments illustrate that the proposed method predicts the traffic state evolution in a freeway network within 30 minutes with accuracy varying from 75%-95%. It has a better performance compared to the tested baseline models (APTN, Graph CNN-LSTM, and so on). The experimental results also illustrate that factoring traffic dependency and integrating data assimilation techniques can improve prediction accuracy.
更多查看译文
关键词
Predictive models,Roads,Hidden Markov models,Data models,Boundary conditions,Traffic control,Deep learning,Traffic state evolution,traffic flow model,deep learning,graph theory
AI 理解论文
溯源树
样例
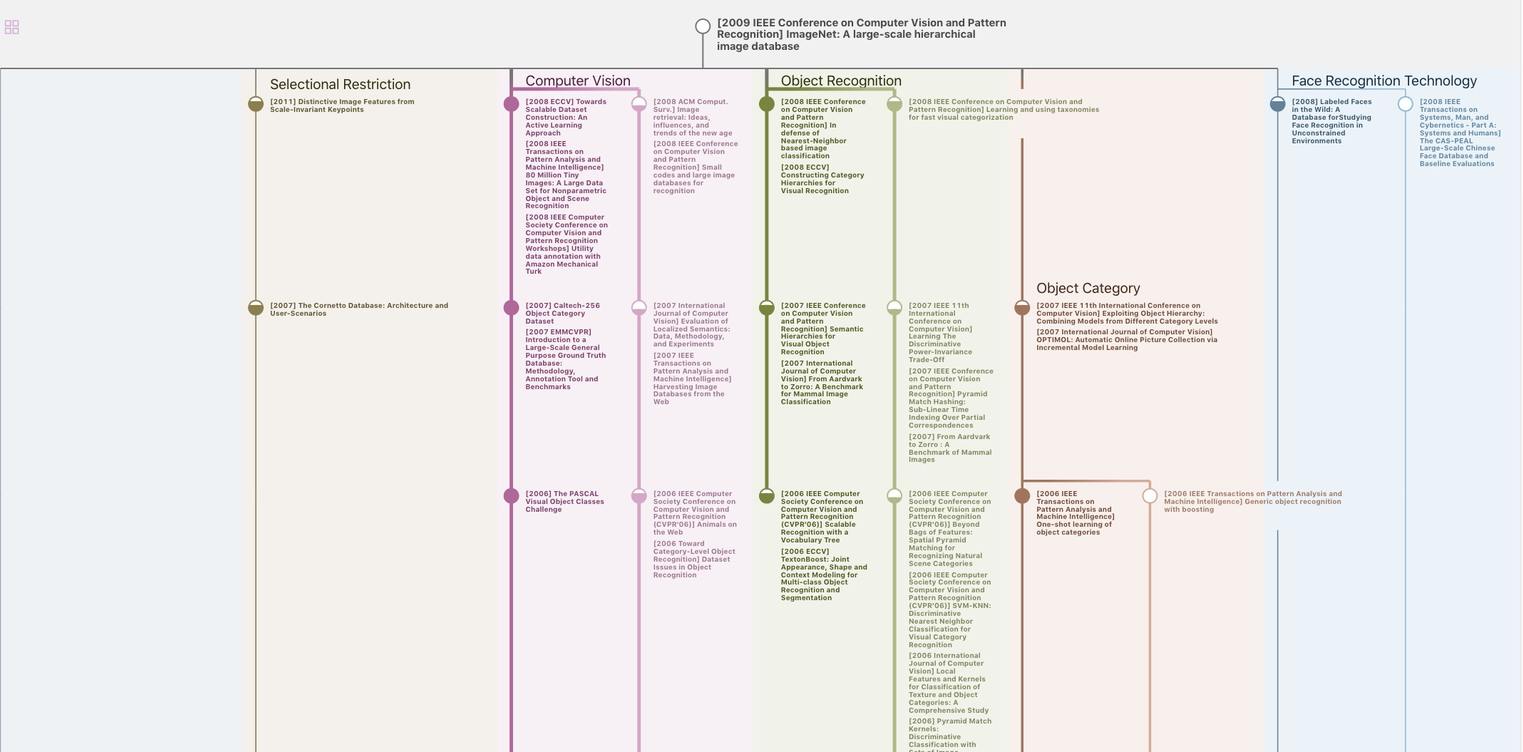
生成溯源树,研究论文发展脉络
Chat Paper
正在生成论文摘要