A Dual-Critic Deep Deterministic Policy Gradient Approach for Task Offloading in Edge-Fog-Cloud Environment.
2023 International Conference on Computer and Applications (ICCA)(2023)
摘要
Computation intensive IoT applications have grown exponentially over the past few years, which has led to the rise in popularity of the edge, fog, and cloud computing paradigms among mobile devices with embedded smart chips. Mobile Edge Computing (MEC) and Fog Computing (FC) usually cooperate with cloud computing to fulfill the high-demand requirements and optimize the network resources of complex computational offloading environments. In this paper, the topic of computational offloading for the hierarchical Edge-Fog-Cloud (EFC) computing is examined where edge, fog, and cloud nodes function as relay nodes that are constructed for latency critical and computations intensive jobs. Hierarchical EFC makes use of both centralized and distributed computing architectures considering diverse channel quality, network selection, and cloud access. This study proposes an improved Deep Reinforcement Learning (DRL) algorithm to find the optimal decision for offloading a computational task in EFC networks. Simulation results validate the proposed DRL-based model by comparing it with other alternative algorithms. Experimental results demonstrate how the proposed approach can efficiently reduce the latency delay by 43% and energy cost by 32% of IoT end devices. Additionally, it can achieve a higher success rate compared with the other related algorithms with more than 16%.
更多查看译文
关键词
Deep Reinforcement Learning,Internet of Things,Fog Computing,Mobile Edge Computing,Task Offloading.
AI 理解论文
溯源树
样例
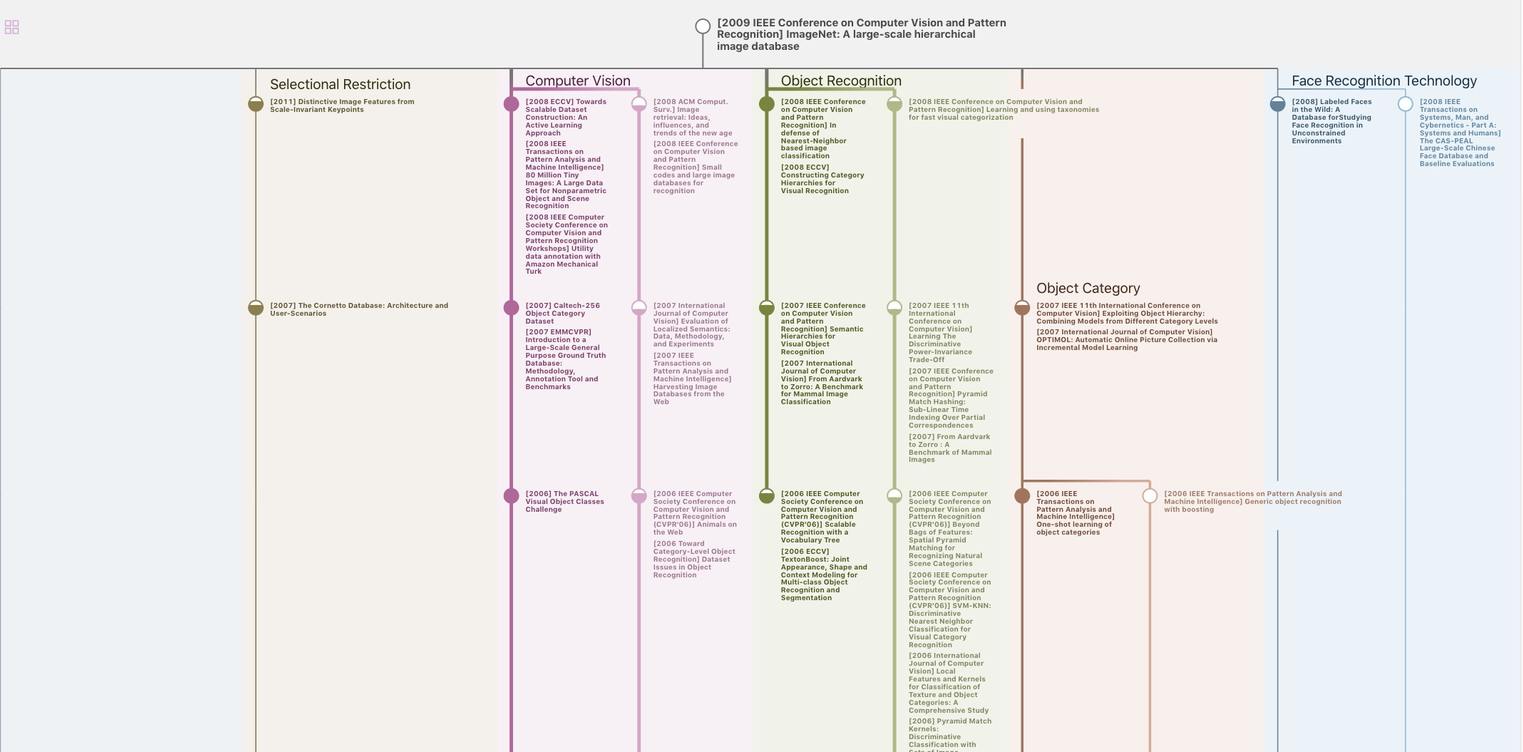
生成溯源树,研究论文发展脉络
Chat Paper
正在生成论文摘要