Robust Learning-based Model Predictive Control for Intelligent Vehicles with Unknown Dynamics and Unbounded Disturbances
IEEE Transactions on Intelligent Vehicles(2023)
摘要
Autonomous driving is producing various novel achievements in integrating different control technologies with machine learning to control intelligent vehicles, i.e., learning-based control. Yet, few work formally consider the confidence of learning-based models and their residual model uncertainties. As a result, existing methods lack safety guarantees in real-world applications. To address this issue, this work proposes a Linear-Bayesian-Regression-based Model Predictive Control (MPC) method for vehicles with unknown dynamics and subject to possibly unbounded disturbances. Model parameters can be obtained by using posterior calculations via prior estimations and systems’ historical data. The expected value of resulting posterior distribution is chosen as linear model's parameters for controller design. Furthermore, the corresponding posterior distribution can be used to derive an upper bound for the variance of residual model uncertainties. In analogy to the concept of Tube-MPC, this work introduces a dynamic feedback gain which is combined with the standard nominal MPC method. It derives provable security guarantees with respect to state and input chance constraints for complex unknown dynamical systems in the form of probabilities. A convex-reformulation-based optimization method is proposed to enable efficient computation of control laws and ensure the recursive feasibility. Simulations of a Vander Pol's oscillator's control and a vehicle's tracking control illustrate the design procedure and effectiveness of the proposed method.
更多查看译文
关键词
Unknow dynamical systems,linear Bayesian regression,model predictive control,unbounded disturbances,learning-based control
AI 理解论文
溯源树
样例
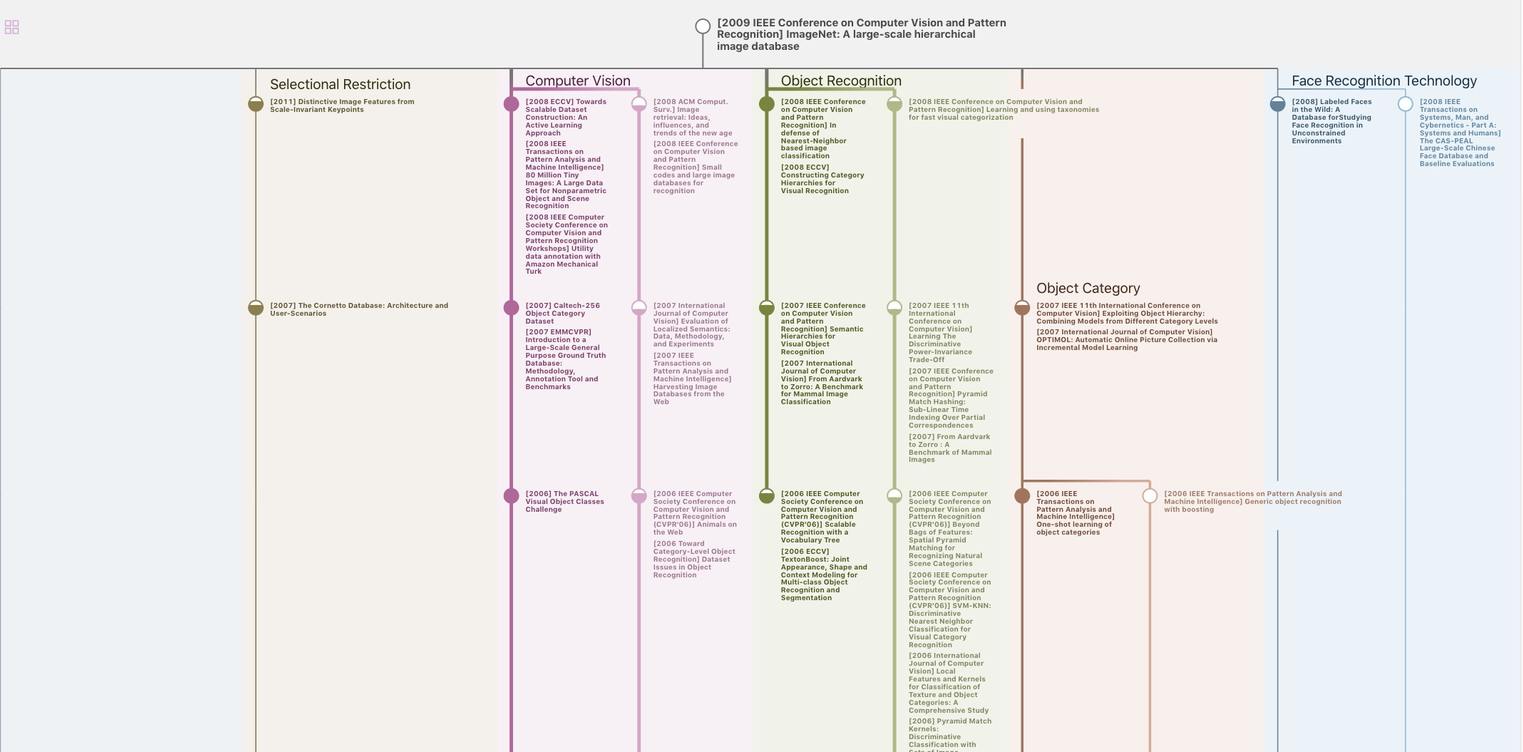
生成溯源树,研究论文发展脉络
Chat Paper
正在生成论文摘要