Benchmarking Anomaly Detection Algorithms using Hyperparameter Optimization.
2023 IEEE 19th International Conference on Intelligent Computer Communication and Processing (ICCP)(2023)
摘要
Anomaly detection is the technique of finding out-of-the-ordinary occurrences inside datasets. A multitude of anomaly detection methods was developed for this purpose in a wide variety of domains (e.g., economy, industrial processes, health, environmental monitoring, etc.), using as support more generic artificial intelligence and signal processing algorithms. The performance of these algorithms is strongly influenced by the initial dataset characteristics and by the hyperparameter settings that users choose for each algorithm. There is no a-priory best solution for a given domain or type of dataset; therefore, the researchers must test a multitude of methods with multiple hyperparameter settings. The research community does not yet have a multi-perspective assessment of the performance of these algorithms. Most of the work is focused on very specific problems or domains, and the algorithms are evaluated only from one or a few perspectives, using the ”default” settings, without hyperparameter optimization. In this article, we will discuss the findings obtained from an extensive anomaly detection benchmarking that covers 34 anomaly detection algorithms, tested on several relevant datasets from a wide area of domains. The results are presented from multiple perspectives. For each algorithm and dataset, a hyperparameter optimization approach is used in order to establish the optimal settings for each algorithm in each test case. This study discloses both the merits and limitations of the various techniques and proposes a taxonomy of anomaly detection algorithms based on the nature of the problem and the characteristics of the input data.
更多查看译文
关键词
Artificial Intelligence,Anomaly Detection,Benchmarking,Taxonomy of Anomaly Detection Algorithms
AI 理解论文
溯源树
样例
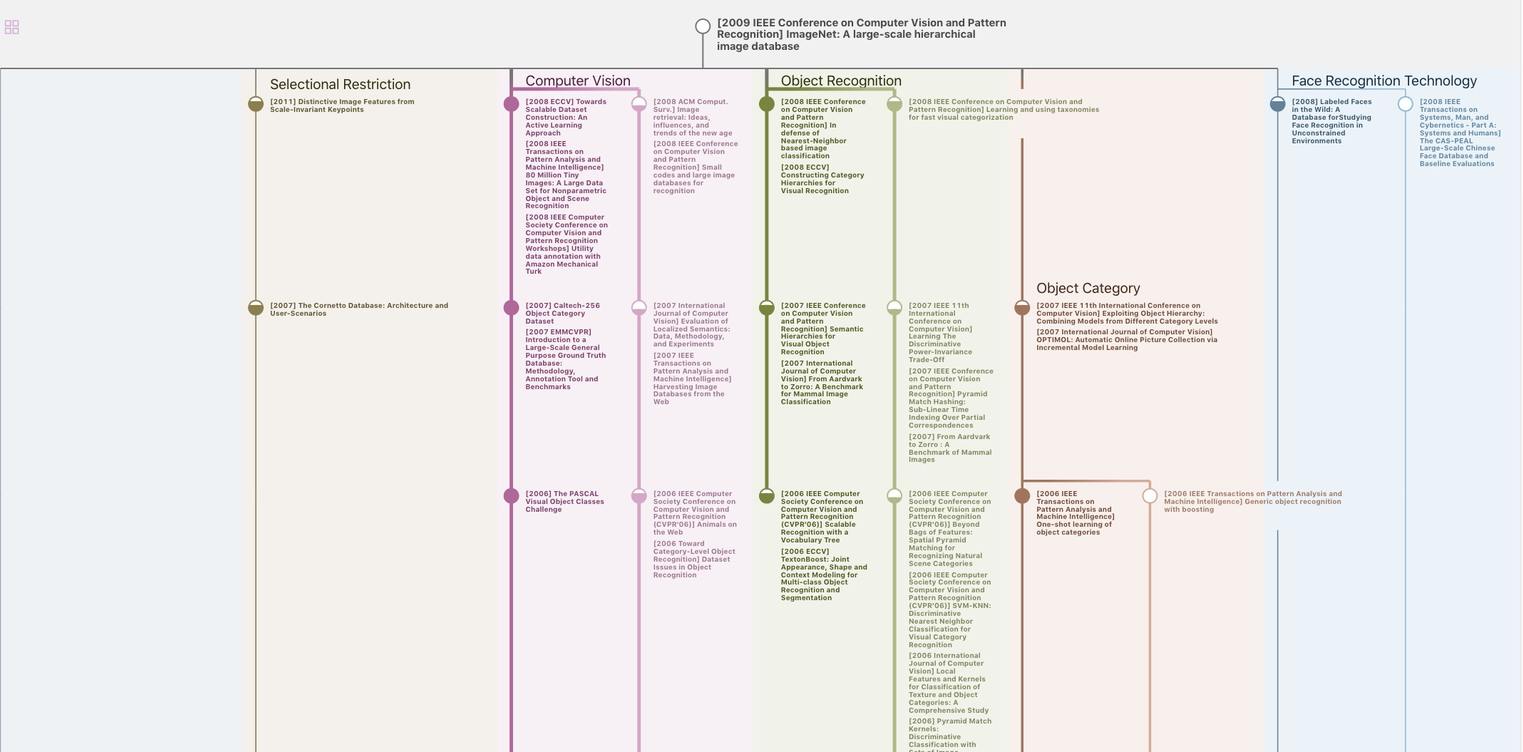
生成溯源树,研究论文发展脉络
Chat Paper
正在生成论文摘要