Human-Robot Collaboration Through a Multi-Scale Graph Convolution Neural Network With Temporal Attention
IEEE ROBOTICS AND AUTOMATION LETTERS(2024)
摘要
Collaborative robots sensing and understanding the movements and intentions of their human partners are crucial for realizing human-robot collaboration. Human skeleton sequences are widely recognized as a kind of data with great application potential in human action recognition. In this letter, a multi-scale skeleton-based human action recognition network is proposed, which leverages a spatio-temporal attention mechanism. The network achieves high-accuracy human action prediction by aggregating multi-level key point features of the skeleton and applying the spatio-temporal attention mechanism to extract key temporal information features. In addition, a human action skeleton dataset containing eight different categories is collected for a human-robot collaboration task, where the human activity recognition network predicts skeleton sequences from a camera and the collaborating robot makes collaborative actions based on the predicted actions. In this study, the performance of the proposed method is compared with state-of-the-art human action recognition methods and ablation experiments are performed. The results show that the multi-scale spatio-temporal graph convolutional neural network has an action recognition accuracy of 94.16%. The effectiveness of the method is also verified by performing human-robot collaboration experiments on a real robot platform in a laboratory environment.
更多查看译文
关键词
Robots,Collaboration,Skeleton,Task analysis,Human activity recognition,Feature extraction,Convolutional neural networks,Human-robot collaboration,intention recognition,skeleton,graph convolutional neural network
AI 理解论文
溯源树
样例
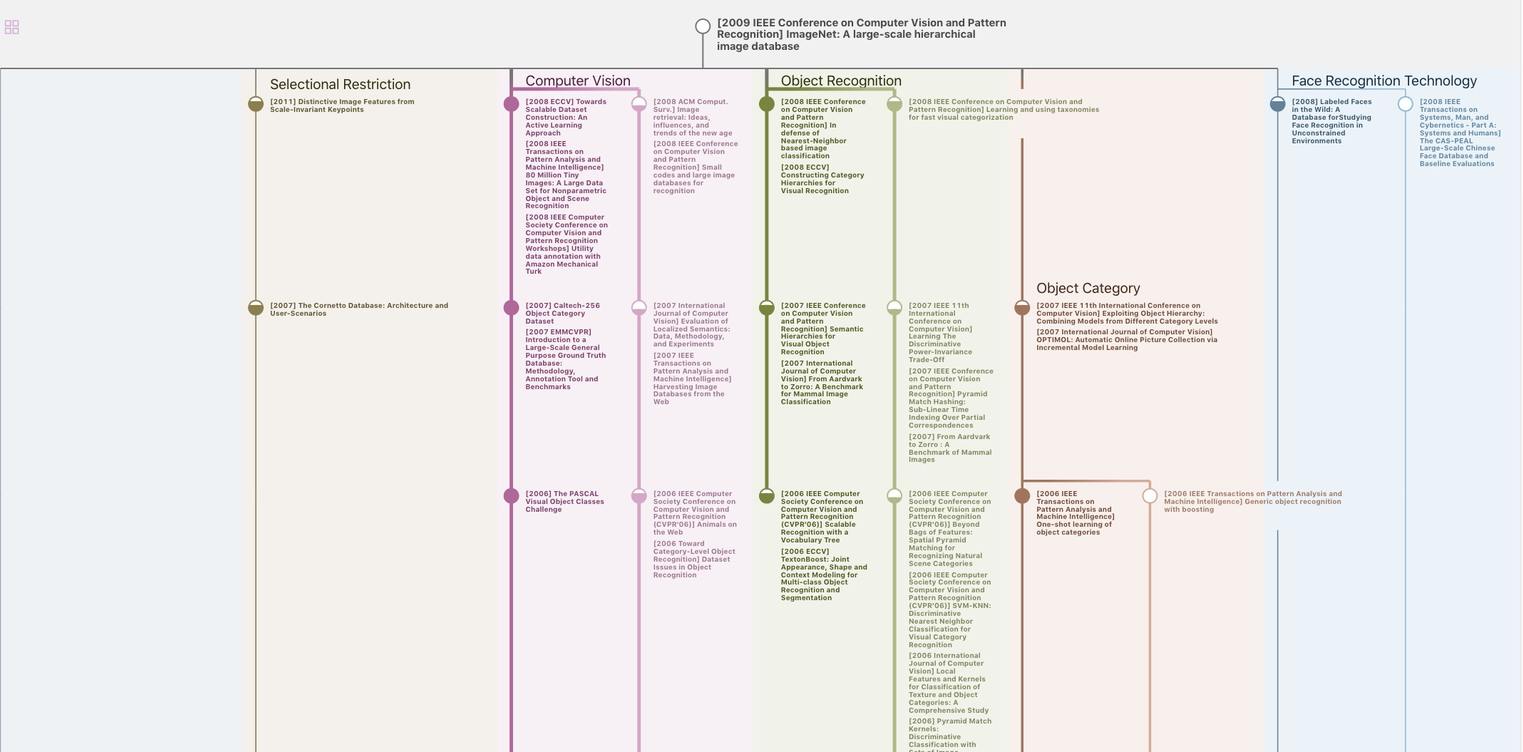
生成溯源树,研究论文发展脉络
Chat Paper
正在生成论文摘要