Class Semantics Modulation for Open-Set Instance Segmentation
IEEE ROBOTICS AND AUTOMATION LETTERS(2024)
摘要
This letter addresses the challenge of open-set instance segmentation (OSIS) which segments both known objects and unknown objects not seen in training, and thus is essential for enabling robots to safely work in the real world. Existing solutions adopt class-agnostic segmentation where all classes share the same mask output layer leading to inferior performance. Motivated by the superiority of the class-specific mask prediction in close-set instance segmentation, we propose SemSeg with class semantics extraction and mask prediction modulation for conducting class-specific segmentation in OSIS. To extract class semantics for both known and unknown objects in the absence of supervision on unknown objects, we use contrastive learning to construct an embedding space where objects from each known class cluster in an independent territory and the complementary region of known classes can accommodate unknown objects. To modulate the mask prediction, we convert class semantic embedding to convolutional parameters used to predict the mask. Class semantics modulated OSIS allows optimizing the mask output layer for each class independently without competition between each other. And class semantic information is engaged in the segmentation process directly so that can guide and facilitate the segmentation task, which benefits unknown objects with severe generalization challenges particularly. Experiments on the COCO and GraspNet-1Billion datasets demonstrate the merits of our proposed method, especially the strength of instance segmentation for unknown objects.
更多查看译文
关键词
Deep learning for visual perception,object detection,segmentation and categorization,visual learning
AI 理解论文
溯源树
样例
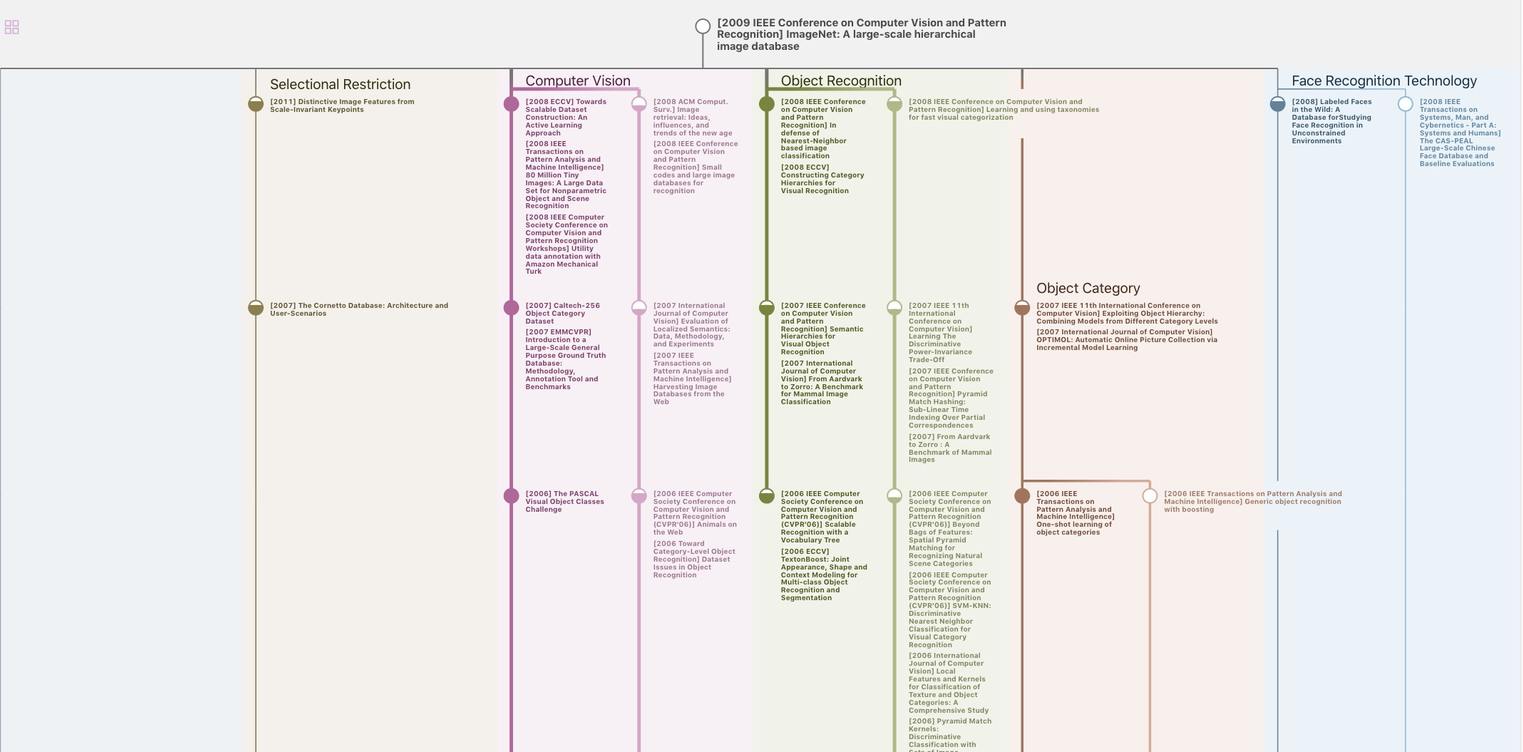
生成溯源树,研究论文发展脉络
Chat Paper
正在生成论文摘要