Decoupled Multi-Class Adaptive Calibration Model for Long-Tailed Recognition.
2023 8th IEEE International Conference on Network Intelligence and Digital Content (IC-NIDC)(2023)
摘要
Although neural networks have achieved high accuracy in image recognition, neural network models are often miscalibrated. Recently, some research has provided some universal methods for improving calibration, such as temperature scaling (TS). However, using the same temperature coefficient for long-tailed problems is inappropriate because the temperature coefficient will focus too much on the head samples. Some methods manually change the temperature coefficient of each category by constructing a function, such as class-conditioned scaling (CLS), but such methods require setting separate functions and hyperparameters for each problem, which is neither convenient nor accurate. In this study, we propose a decoupled multi-class adaptive calibration method(DCAC), which performs calibration processing in both the training stage and the post-calibration stage. Our experience shows that the calibration in the training phase and the post-calibration are independent of each other and can be superimposed to produce better results.
更多查看译文
关键词
Calibration,Long-Tail,Temperature Scaling,Decouple Module
AI 理解论文
溯源树
样例
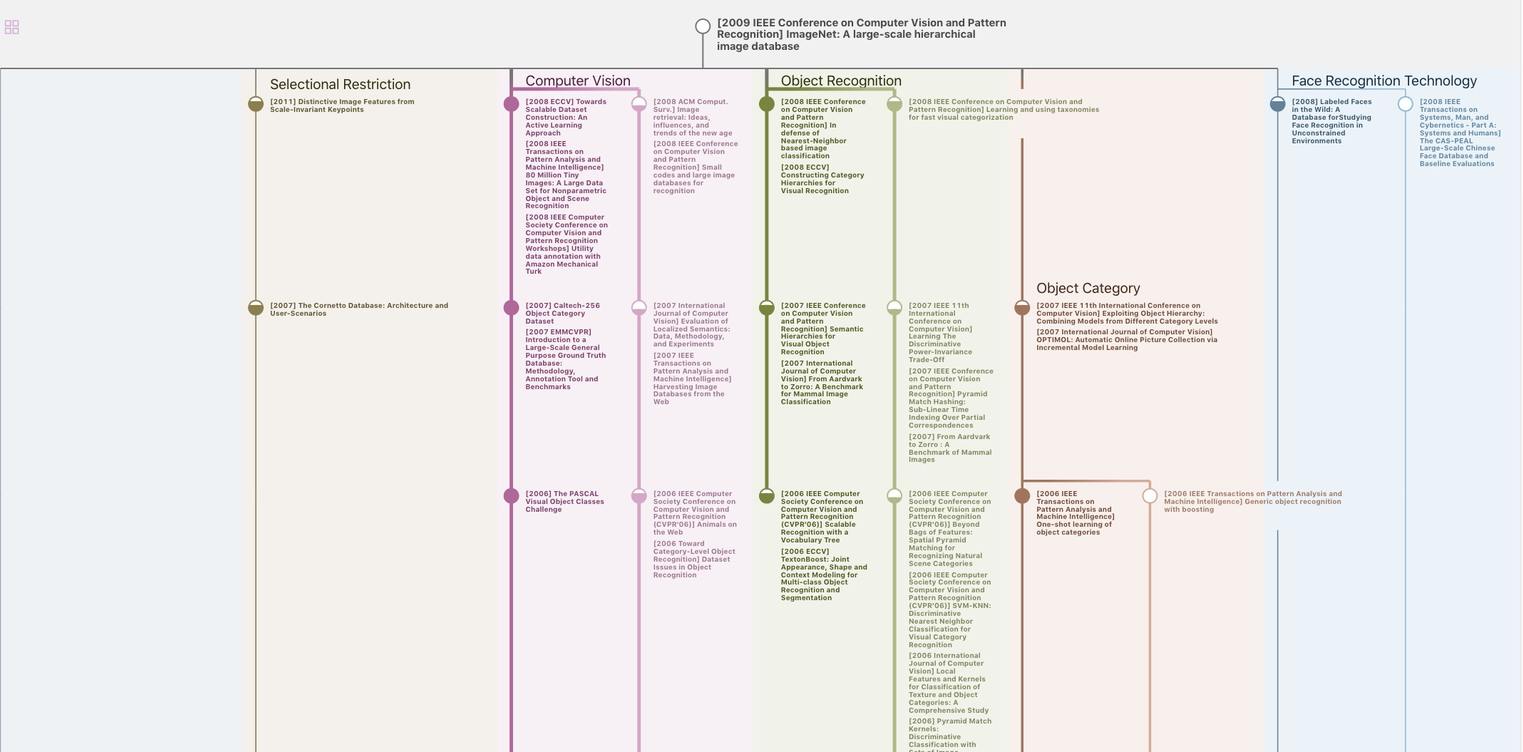
生成溯源树,研究论文发展脉络
Chat Paper
正在生成论文摘要