Dense Local Consistency Loss for Video Semantic Segmentation.
2023 8th IEEE International Conference on Network Intelligence and Digital Content (IC-NIDC)(2023)
摘要
Existing image semantic segmentation models often suffer from temporal inconsistency between consecutive frames when processing continuous video inputs. While using optical flow or incorporating historical frame information can alleviate this issue, the resulting increase in parameters and computational complexity is detrimental to real-time tasks. In contrast, we propose a dense local consistency loss dubbed DLCL, which introduces spatial local semantic consistency constraints between consecutive frames in the task of video semantic segmentation. During training, DLCL is calculated based on the cosine similarity of feature embeddings for the same object in consecutive frames. Our DLCL is simple yet effective, easily integrated into both single-frame and video semantic segmentation models, and improves the temporal consistency and segmentation accuracy of predicted frames without adding any parameters or computational overhead during inference. We conduct experiments on the large-scale multi-scene video semantic segmentation dataset: VSPW, to demonstrate the effectiveness of our approach. The results consistently show performance improvements in both singleframe and video semantic segmentation models, validating the efficacy of our method.
更多查看译文
关键词
Video semantic segmentation,temporal consistency,cosine similarity
AI 理解论文
溯源树
样例
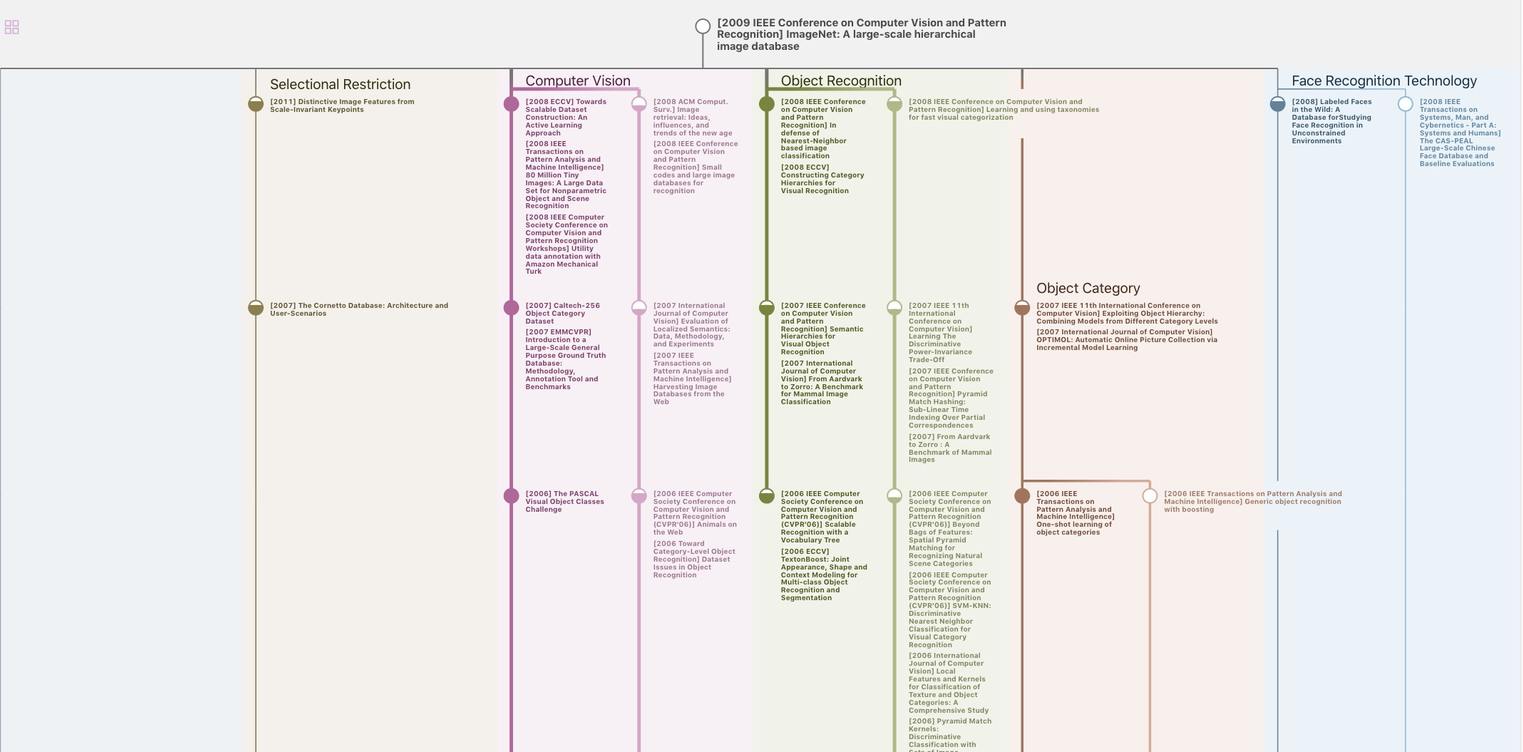
生成溯源树,研究论文发展脉络
Chat Paper
正在生成论文摘要