An Efficient Data-Driven Traffic Prediction Framework for Network Digital Twin
IEEE NETWORK(2024)
摘要
Network digital twin, as a fundamental enabling technology in emerging network scenarios and applications, establishes a connection between the physical and virtual networks through real-time representation and synchronization of physical entities. The value of network digital twins lies in their ability to accurately model future network states and behaviors, facilitating numerous downstream network tasks such as network optimization and management. However, the importance of network traffic prediction as an essential prerequisite module for these network tasks has been relatively overlooked. Limited surveys provide insights into the network traffic characteristics and advanced prediction models, resulting in performance degradation and unnecessary resource consumption. Hence, it deserves to explore an efficient data-driven traffic prediction framework that can adapt to different network tasks. This article first examines the general forms and characteristics of various network traffic based on specific datasets. Subsequently, we introduce a multi-module data-driven framework for network traffic prediction to effectively cater to diverse data types and downstream task requirements. A case study of cellular traffic prediction based on traffic characteristics and requirements is proposed to demonstrate the practicability of our framework. Finally, we outline several open challenges toward future traffic prediction in network digital twins.
更多查看译文
关键词
Time series analysis,Digital twins,Task analysis,Telecommunication traffic,Predictive models,Correlation,Complexity theory,Network Digital Twin,Traffic Prediction,Artificial Intelligence,Transfer Learning,Transformer,Performance Evaluation
AI 理解论文
溯源树
样例
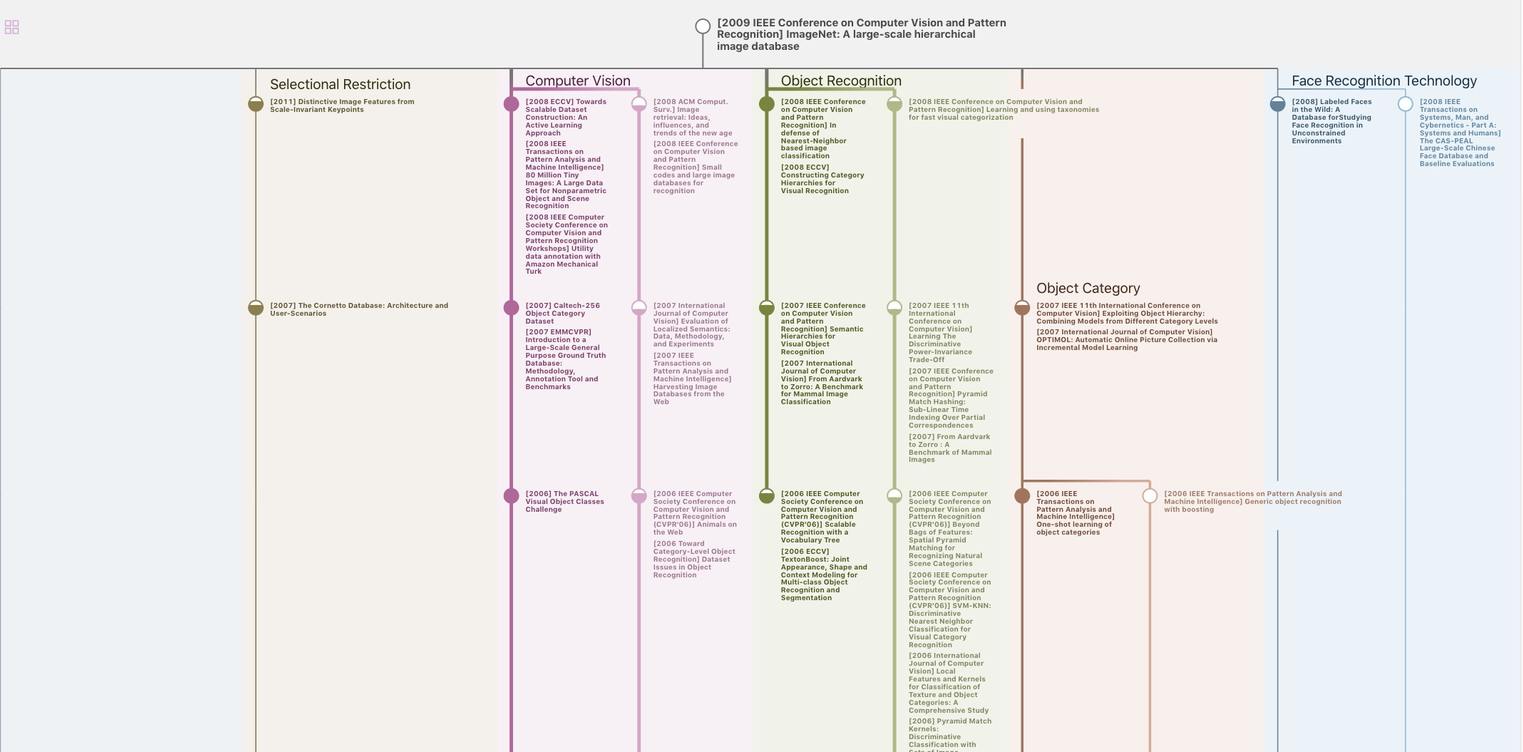
生成溯源树,研究论文发展脉络
Chat Paper
正在生成论文摘要