Efficient Reduced-Order Algorithm for Transient Simulation of Transformer Thermal Characteristics
2023 13th International Conference on Power and Energy Systems (ICPES)(2023)
摘要
Intelligent operation and maintenance of power transformers using digital twin technology is of vital importance for ensuring the safe operation of the power grid. Dynamic simulation and efficient calculation of the thermal characteristics of transformers are key tasks in this area. To achieve fast prediction of temperature rise in transformers under time-varying operating conditions and promote the application of digital twin in substation operation and maintenance, this paper proposes a reduced-order model with full-nodal and multi-time steps output for transient simulation of transformer temperature field based on multi-layer Autoencoder (MAE) and decomposition linear layers (DLinear), and evaluates its effectiveness in terms of computational accuracy and efficiency. The results demonstrate that the proposed reduced-order model for transient simulation achieves high computational accuracy, with a maximum relative error of only 1.82% compared to simulation calculations. At the same time, the model significantly improves computational efficiency, with the ability to make 200-step predictions in only 3s. The research findings of this paper provide strong technical support for monitoring the thermal performance of transformers and the operation and maintenance of substations.
更多查看译文
关键词
transformer thermal simulation,transient model reduction,full-nodal reduction,long-term series forecasting
AI 理解论文
溯源树
样例
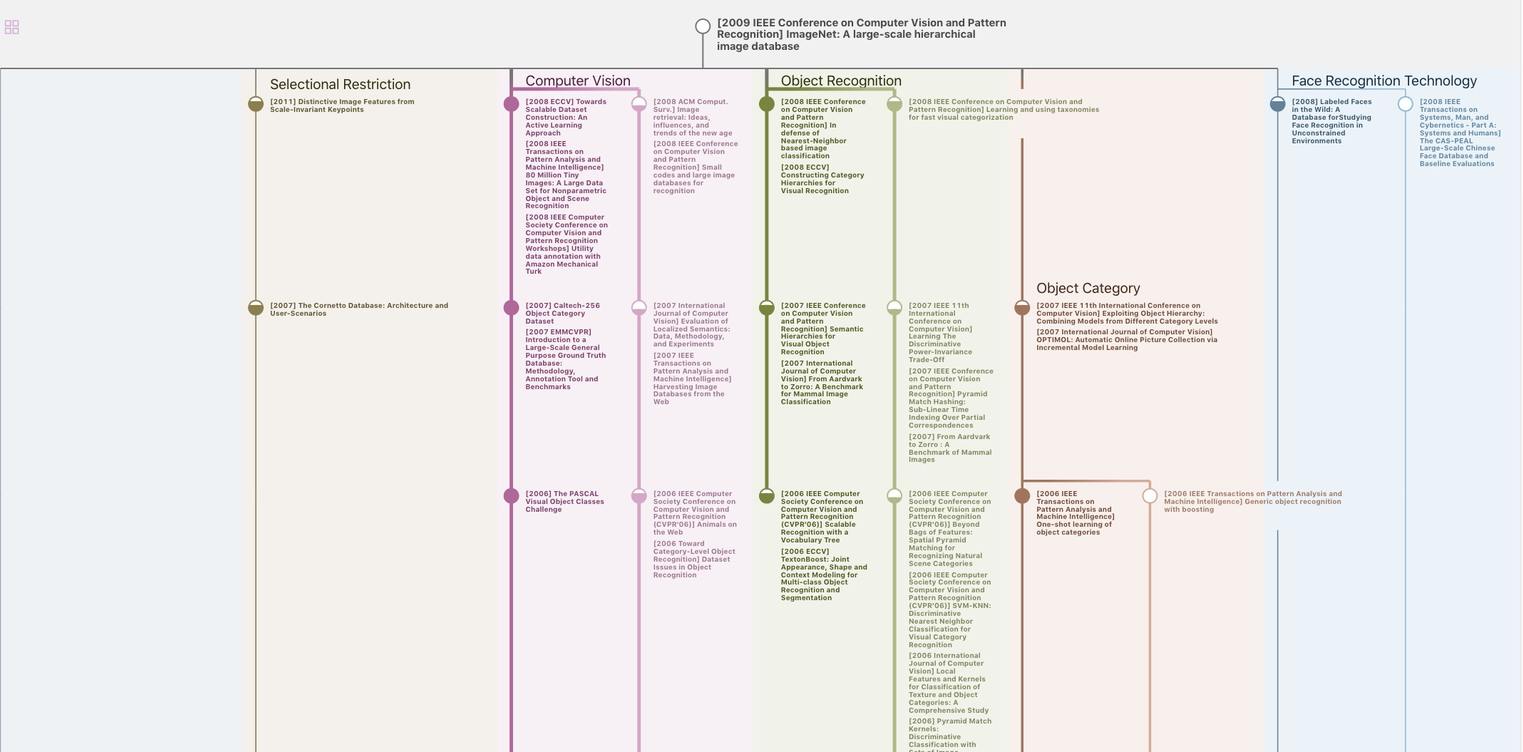
生成溯源树,研究论文发展脉络
Chat Paper
正在生成论文摘要