Assessing the Sensitivity of Semiempirical Models to Spectral Data Quality and Sensor Settings When Estimating Leaf Chlorophyll Content.
IEEE Journal of Selected Topics in Applied Earth Observations and Remote Sensing(2024)
摘要
Leaf chlorophyll content (LCC) is an important indicator of nitrogen content, and therefore, the accurate monitoring of LCC will benefit agronomists in guiding fertilizer applications. Remote sensing techniques have been widely used to estimate LCC from canopy reflectance spectra. However, there is no sensor specific to the estimation of LCC from canopy reflectance spectra, and it is unclear whether LCC estimation is sensitive to the reflectance quality (such as the noise level) or sensor settings (such as spectral resolution). To help design a sensor specific to the estimation of LCC, this study calibrated a semiempirical model based on the leaf area index-insensitive chlorophyll index (LICI) and evaluated its sensitivity to reflectance quality and sensor settings using simulated, measured, and artificial datasets. Our results indicated that random Gaussian noise in reflectance has limited effects on LCC estimation when the level of Gaussian noise is less than 2%, while the negative systematic bias in reflectance has clear effects on the LCC estimation. The LCC estimation accuracy is nearly independent of the spectral resolution (<30 nm) and the field of view (<100°). However, the LCC estimation is clearly sensitive to the shift toward longer wavelengths, which will lead to the overestimation of LCC. Overall, these findings will help design a sensor specific to the estimation of LCC and improve our understanding of the application of an LICI-based model, which is useful for fertilizer management in precision agriculture.
更多查看译文
关键词
Leaf area index-insensitive chlorophyll index (LICI),leaf chlorophyll content (LCC),semiempirical model,sensitivity analysis
AI 理解论文
溯源树
样例
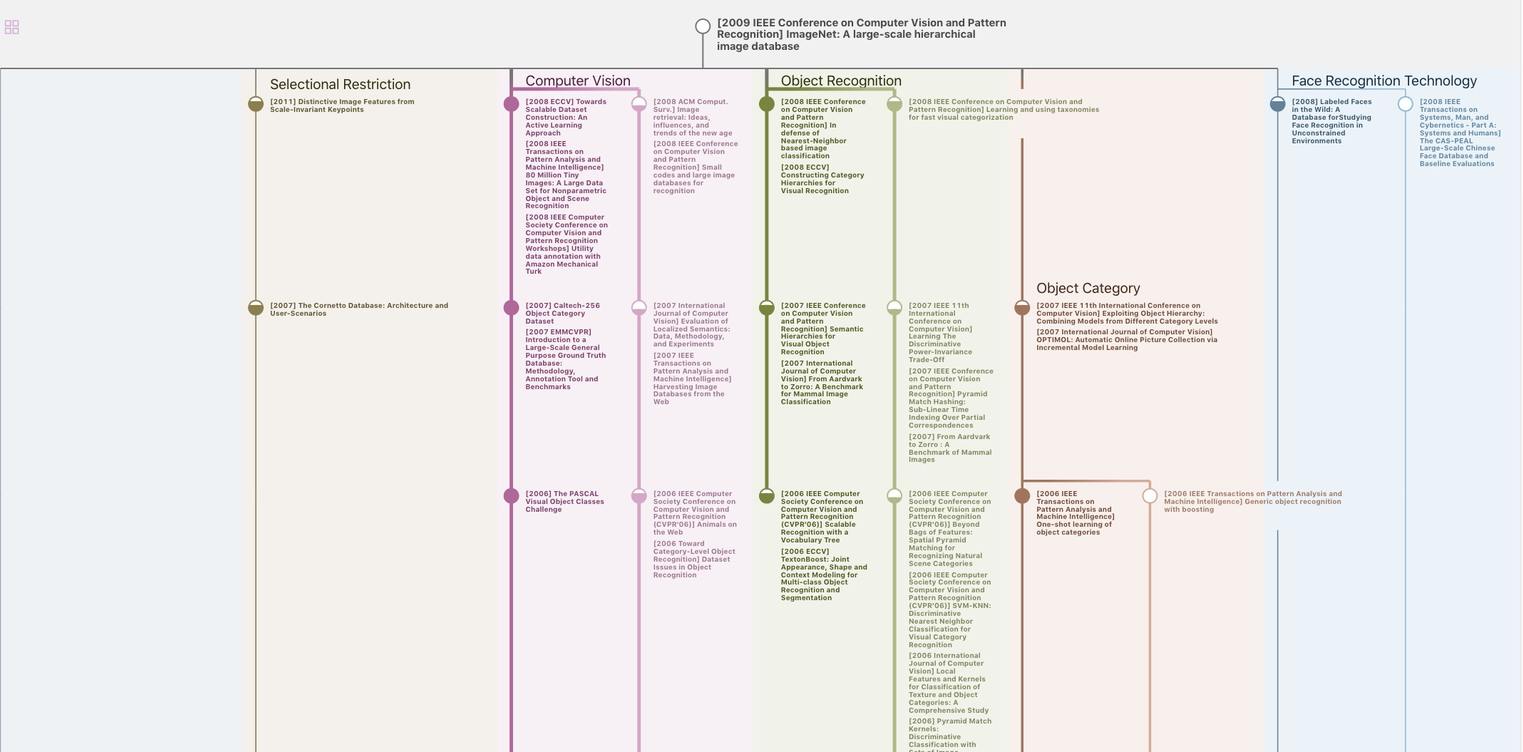
生成溯源树,研究论文发展脉络
Chat Paper
正在生成论文摘要