Personalized Federation Learning with Model-Contrastive Learning for Multi-Modal User Modeling in Human-Centric Metaverse
IEEE Journal on Selected Areas in Communications(2024)
摘要
With the flourish of digital technologies and rapid development of 5G and beyond networks, Metaverse has become an increasingly hotly discussed topic, which offers users with multiple roles for diversified experience interacting with virtual services. How to capture and model users’ multi-platform or cross-space data/behaviors become essential to enrich people with more realistic and immersed experience in Metaverse-enabled smart applications over 5G and beyond networks. In this study, we propose a Personalized Federated Learning with Model-Contrastive Learning (PFL-MCL) framework, which may efficiently enhance the communication and interaction in human-centric Metaverse environments by making use of the large-scale, heterogeneous, and multi-modal Metaverse data. Differing from the conventional Federated Learning (FL) architecture, a multi-center aggregation structure to learn multiple global models based on the changes of dynamically updated local model weights, is developed in global, while a hierarchical neural network structure which includes a personalized module and a federated module to tackle both issues on data heterogeneity and model heterogeneity, is designed in local, so as to enhance the performance of PFL with unique characteristics of Metaverse data. In particular, a two-stage iterative clustering algorithm with a more precise initialization is developed to facilitate the personalized global aggregation with dynamically updated multiple aggregation centers. A personalized multi-modal fusion network is constructed to greatly reduce the computational cost and feature dimensions from the high-dimensional heterogeneous inputs for more efficient cross-modal fusion, based on a hierarchical shift-window attention mechanism and a newly designed bridge attention mechanism. A MCL scheme is then incorporated to speed up the model convergence with less communication overload between the local federated module and global model, while an embedding layer which effectively enables the delivered global model to better adapt to the local personality in each client is further integrated. Compared with five baseline methods, experiment and evaluation results based on two different real-world datasets demonstrate the excellent performance of our proposed PFL-MCL model in a fine-grain personalized training strategy, toward more efficient communication and networking among human-centric Metaverse enabled smart applications.
更多查看译文
关键词
Personalized Federated Learning,Model-Contrastive Learning,Attention Mechanism,Multi-Modal Fusion,Human-Centric,Metaverse
AI 理解论文
溯源树
样例
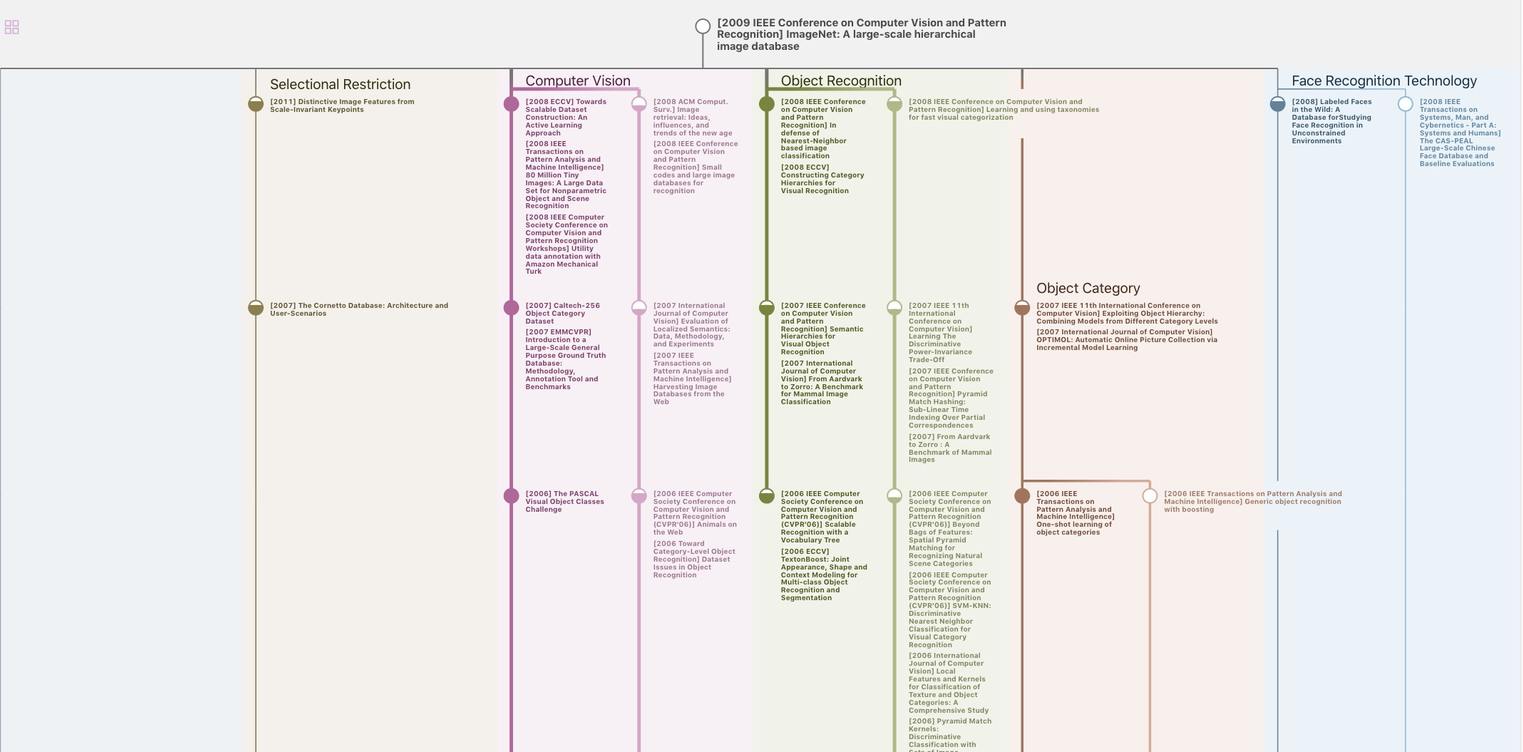
生成溯源树,研究论文发展脉络
Chat Paper
正在生成论文摘要