AFE-Net: Attention-Guided Feature Enhancement Network for Infrared Small Target Detection
IEEE JOURNAL OF SELECTED TOPICS IN APPLIED EARTH OBSERVATIONS AND REMOTE SENSING(2024)
摘要
Infrared small target detection is considerably challenging due to the few pixels in targets, low signal-to-noise ratio, and complex background. In this article, we propose an effective attention-guided feature enhancement network (AFE-Net), which can leverage the local and nonlocal features of targets and background in infrared images. The AFE-Net consists of three key modules, namely encoder and decoder interactive guidance (EDIG) module, cascading false alarm removal (CFAR) module, and random scale input (RSI) module. Specifically, in the EDIG module, we employ a CA mechanism on encoding and decoding layers to select feature channels with higher contribution. Then, we impose a bottom-up pointwise attention block to highlight the features of small infrared targets and suppress possible noise by incorporating the low-level detailed features into the high-level semantic features. The CFAR module extracts affluent global features by cascading nonlocal operations of different layers, which can remove clutters with similar features to infrared targets. The RSI module is placed in front of the entire detection network to extract multiscale features of infrared small targets, which can enhance the robustness of the proposed network. Experimental results on the SIRST dataset and comprehensive comparisons with representative methods demonstrate the superiority of our proposed method.
更多查看译文
关键词
Feature extraction,Object detection,Clutter,Sparse matrices,Semantics,Decoding,Task analysis,Attention mechanism,convolutional neural network (CNN),infrared small target detection,nonlocal dependency
AI 理解论文
溯源树
样例
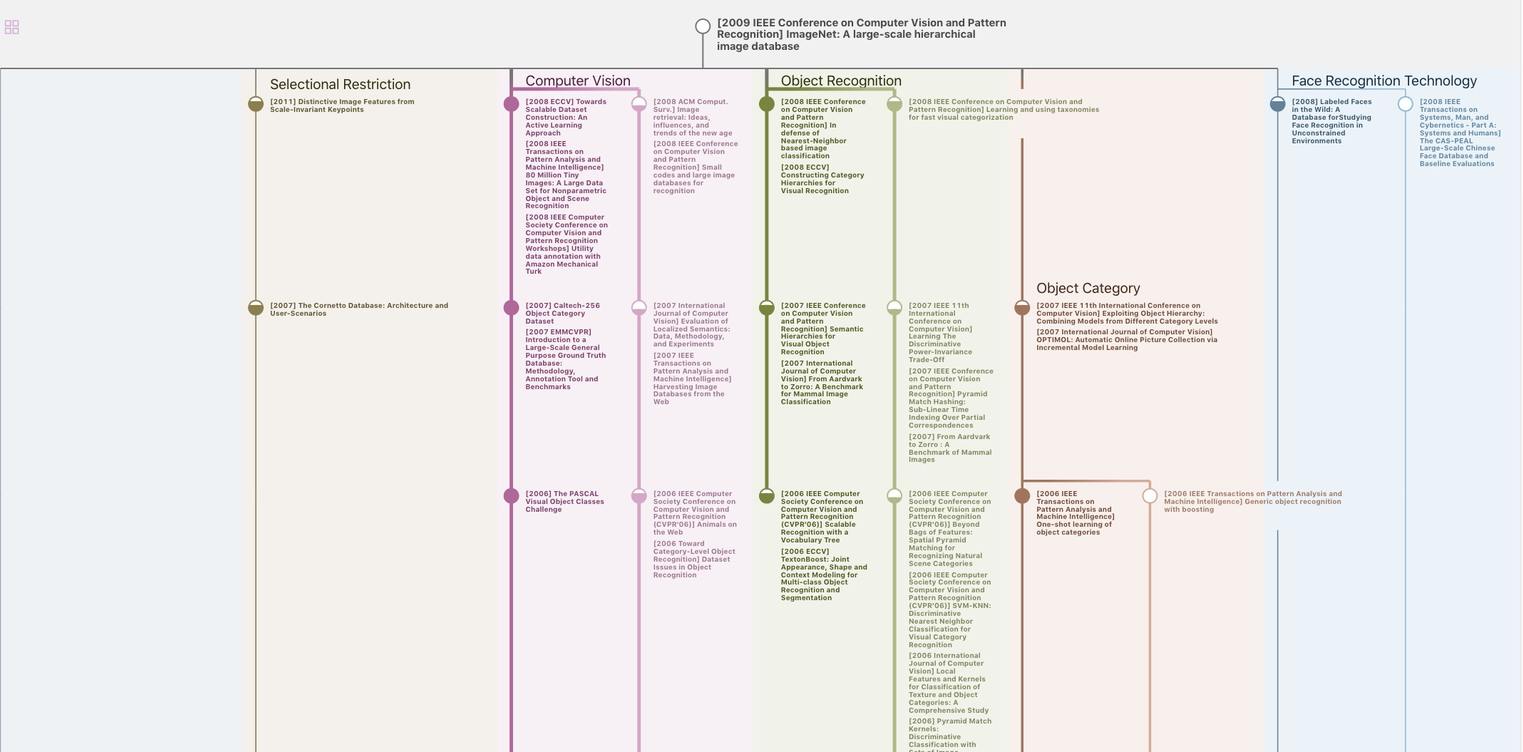
生成溯源树,研究论文发展脉络
Chat Paper
正在生成论文摘要