Conventional to Deep Ensemble Methods for Hyperspectral Image Classification: A Comprehensive Survey
IEEE JOURNAL OF SELECTED TOPICS IN APPLIED EARTH OBSERVATIONS AND REMOTE SENSING(2024)
摘要
Hyperspectral image classification (HSIC) has become a hot research topic. Hyperspectral imaging (HSI) has been widely used in a wide range of real-world application areas due to the in-depth spectral information stored within each pixel. Noticeably, the detailed features, i.e., a nonlinear correlation between the obtained spectral data and the correlating HSI data object, generate efficient classification results that are complex for traditional techniques. Deep learning (DL) has recently been validated as an influential feature extractor that efficiently identifies the nonlinear issues that have arisen in various computer vision challenges. This motivates using DL for HSIC, which shows promising results. This survey provides a brief description of DL for HSIC and compares cutting-edge methodologies in the field. We will first summarize the key challenges for HSIC, and then, we will discuss the superiority of DL and DL ensemble in addressing these issues. In this article, we divide state-of-the-art DL methodologies and DL with ensemble into spectral features, spatial features, and combined spatial-spectral features in order to comprehensively and critically evaluate the progress (future research directions as well) of such methodologies for HSIC. Furthermore, we will take into account that DL involves a substantial percentage of labeled training images, whereas obtaining such a number for HSI is time and cost consuming. As a result, this survey describes some methodologies for improving the classification performance of DL techniques, which can serve as future recommendations.
更多查看译文
关键词
Convolutional neural network (CNN),deep ensemble,deep learning (DL),hyperspectral image classification (HSIC),spatial features,spectral features
AI 理解论文
溯源树
样例
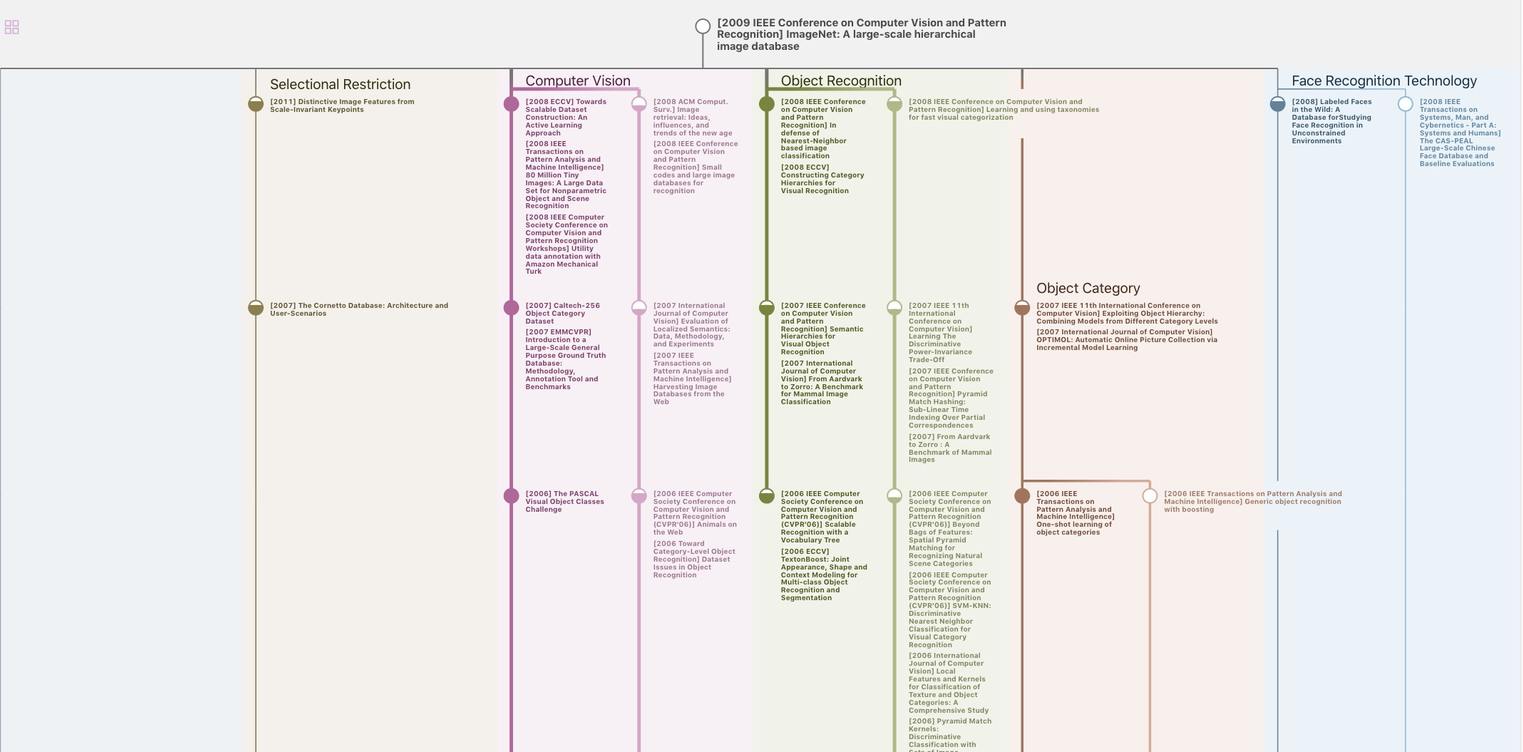
生成溯源树,研究论文发展脉络
Chat Paper
正在生成论文摘要