Dynamic QoS Prediction With Intelligent Route Estimation Via Inverse Reinforcement Learning
IEEE TRANSACTIONS ON SERVICES COMPUTING(2024)
摘要
Dynamic quality of service (QoS) measurement is crucial for discovering services and developing online service systems. Collaborative filtering-based approaches perform dynamic QoS prediction by incorporating temporal information only but never consider the dynamic network environment and suffer from poor performance. Considering different service invocation routes directly reflect the dynamic environment and further lead to QoS fluctuations, we coin the problem of Dynamic QoS Prediction (DQP) with Intelligent Route Estimation (IRE) and propose a novel framework named IRE4DQP. Under the IRE4DQP framework, the dynamic environment is captured by Network Status Representation, and the IRE is modeled as a Markov decision process and implemented by a deep learning agent. After that, the DQP is achieved by a specific neural model with the estimated route as input. Through collaborative training with reinforcement and inverse reinforcement learning, eventually, based on the updated representations of the network status, IRE learns an optimal route policy that matches well with observed QoS values, and DQP achieves accurate predictions. Experimental results demonstrate that IRE4DQP outperforms SOTA methods on the accuracy of response-time prediction by 5.79-31.34% in MAE, by 1.29-20.18% in RMSE, and by 4.43-27.73% in NMAE and with a success rate of nearly 45% on finding routes.
更多查看译文
关键词
Quality of service,Reinforcement learning,Estimation,Predictive models,Peer-to-peer computing,Network topology,Heuristic algorithms,Deep learning,inverse reinforcement learning,QoS prediction,reinforcement learning,route estimation
AI 理解论文
溯源树
样例
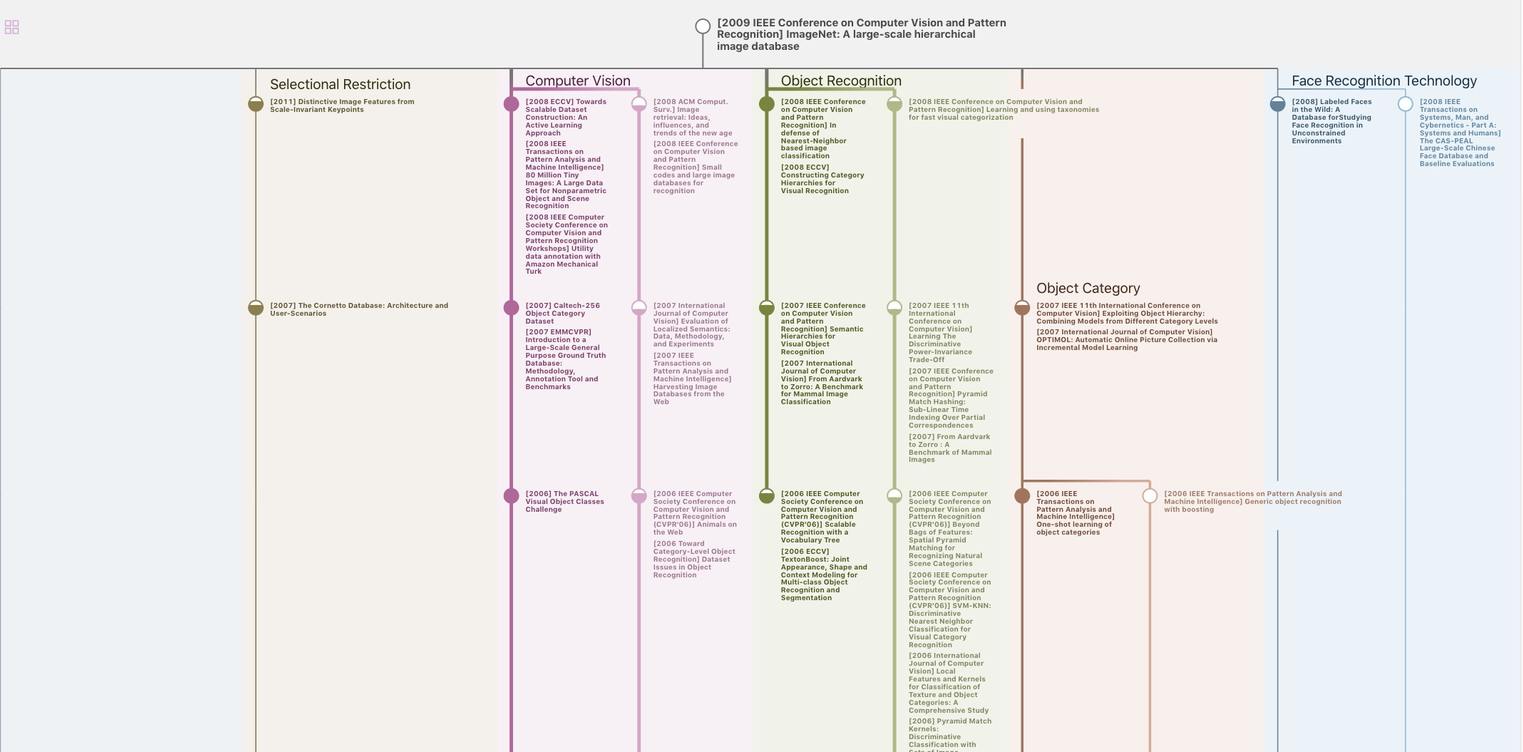
生成溯源树,研究论文发展脉络
Chat Paper
正在生成论文摘要