ESC-Net: Alleviating Triple Sparsity on 3D LiDAR Point Clouds for Extreme Sparse Scene Completion.
IEEE Trans. Multim.(2024)
摘要
3D scene completion (SC) has made progress in the last three years. From the application of mobile robot system, SC should support the downstream task (i.e. mapping or perception), instead of only predicting the completed scenes. However, as the low-cost few-beam LiDAR is widely applied in mobile robot, gap between SC and downstream tasks is large. To generate the high quality completion result, the bottleneck lies in the triple sparsity of input, ground truth (GT) occupancy, and GT foreground. To deal with the triple sparsity, we present an extreme sparse scene completion network (ESC-Net). At first, input sparsity hides most of the spatial information of the scene. A feature completion (FC) decoder is designed to mine the spatial feature using feature-level completion. Then, GT occupancy sparsity hinders representation learning of the real scene with continuous surfaces. A multi-view multi-task attention (MMA) loss is presented to recover the high-quality object boundaries via correcting occupancy and semantic labels of regions from 3D and bird's eye view (BEV) spaces. After that, GT foreground sparsity is the imbalance of foreground and background GT labels. It causes the inaccuracy of local 3D object completion. A combination network (ESC-Net-D) is presented to recover 3D structural details of both foreground and background. Experiment is conducted on KITTI and SemanticPOSS datasets. It shows that ESC-Net has the performance higher than current methods not only on completion task, but also on the downstream tasks (i.e. 3D registration, 3D object detection). Hence, we believe that ESC-Net benefits to the community of mobile robot. Source code is released soon.
更多查看译文
关键词
Light detection and ranging,Sparse point cloud,Voxel representation,Scene completion,Multi-task learning
AI 理解论文
溯源树
样例
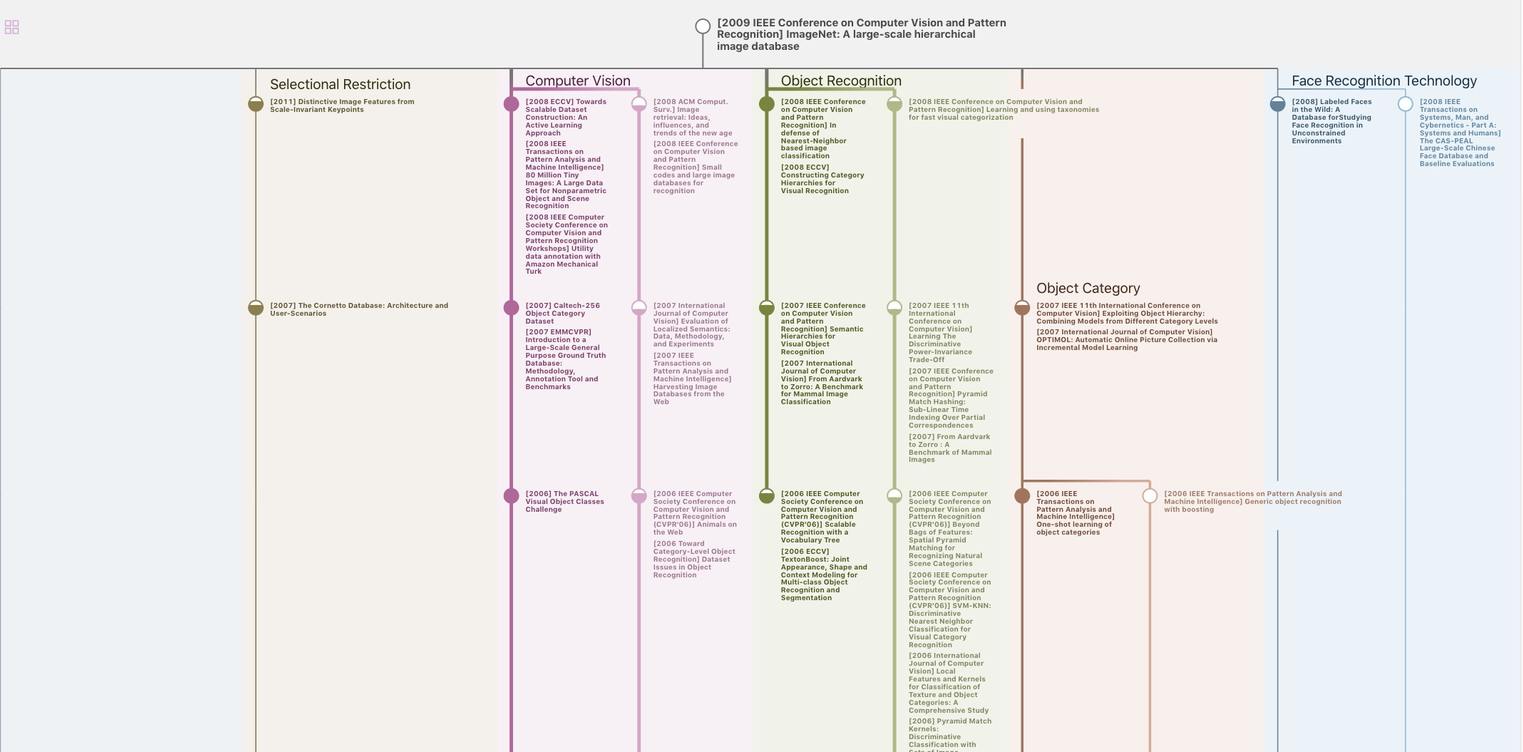
生成溯源树,研究论文发展脉络
Chat Paper
正在生成论文摘要