Dynamical Differential Covariance based Brain Network for Motor Intent Recognition
IEEE Sensors Journal(2024)
Abstract
In the field of motor imagery (MI) recognition based on electroencephalogram (EEG), complex network-based analysis of brain connectivity has gained significant attention. However, there are inherent limitations in both functional connectivity (FC) and effective connectivity (EC) approaches. FC-based networks commonly fail to capture directional information between channels, while EC-based networks require high computational and sample complexity. To address these challenges, we propose a novel algorithm called the dynamic differential covariance (DDC) algorithm for constructing EEG-based brain functional networks. This algorithm simultaneously considers the directionality of effective connectivity and the efficiency of functional connectivity, allowing for the detection of directional interactions and statistical estimates of causality. Using a motor imagery dataset based on the bowl-ball paradigm, we apply the proposed DDC algorithm to construct a causal brain network and extract global and local network topological attributes. To enhance classification performance, we fuse all features and perform feature selection using the ReliefF algorithm. Results demonstrate that our proposed method outperforms phase locking value (PLV)-based brain functional networks in terms of average classification accuracy on the virtual bowl-ball paradigm dataset. Furthermore, the selected feature set exhibits superior classification accuracy and robustness compared to using unfused features or fused features, achieving a classification accuracy of over 90%. These findings contribute to the development of more effective algorithms for EEG-based brain network analysis, emphasizing the importance of comprehensively considering both directionality and efficiency in network construction.
MoreTranslated text
Key words
Motor imagery,Dynamic differential covariance,Brain functional networks,Topological attributes,Feature classification
AI Read Science
Must-Reading Tree
Example
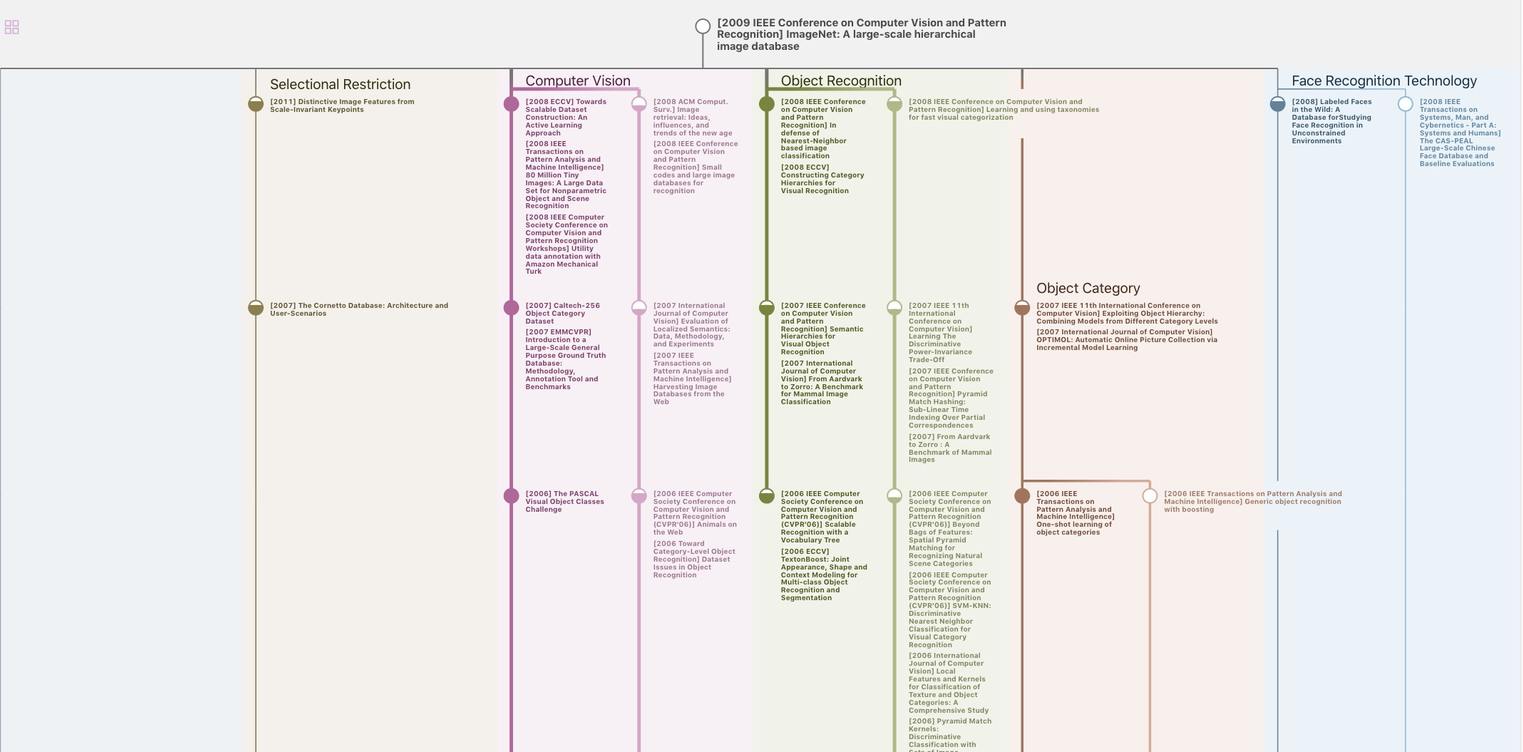
Generate MRT to find the research sequence of this paper
Chat Paper
Summary is being generated by the instructions you defined