Coupled Matrix Factorization Constrained Deep Hyperspectral and Multispectral Image Fusion
IEEE Sensors Journal(2024)
摘要
The application of convolutional neural networks (CNNs) has yielded remarkable outcomes in the fusion of hyperspectral and multispectral images (HSI+MSI). However, most existing researches design black-box models for the direct reconstruction of high-resolution images from low-resolution images, which cannot theoretically guarantee the fusion mechanism throughout the network flow, thus limiting the restoration accuracy. This paper proposes a novel coupled matrix factorization (CMF) constrained deep interpretable network for HSI+MSI fusion, termed as CMF-FUSnet. Specifically, the iterative process of coupled matrix factorization is unfolded into a two-branch network interwoven with multiple denoiser modules and matrix factorization modules. In each iteration, the CMF-encoded two-branch sub-network alternately decomposes HSI and MSI to estimate their abundance and endmember matrices respectively. High-resolution HSI can be obtained by multiplying the endmembers extracted from the HSI and the abundances extracted from the MSI. The benefit is that the proposed CMF-FUSnet breaks through the black-box operation mode while adopting an end-to-end data-driven model, realizes the embedding of physical meaning, and improves the generalization of the model. Numerical experiments show that our proposed CMF-FUSnet compares favorably with both state-of-the-art model-driven and data-driven fusion methods in terms of visual analysis and quality assessment.
更多查看译文
关键词
Convolutional neural networks,image fusion,coupled matrix factorization,interpretable
AI 理解论文
溯源树
样例
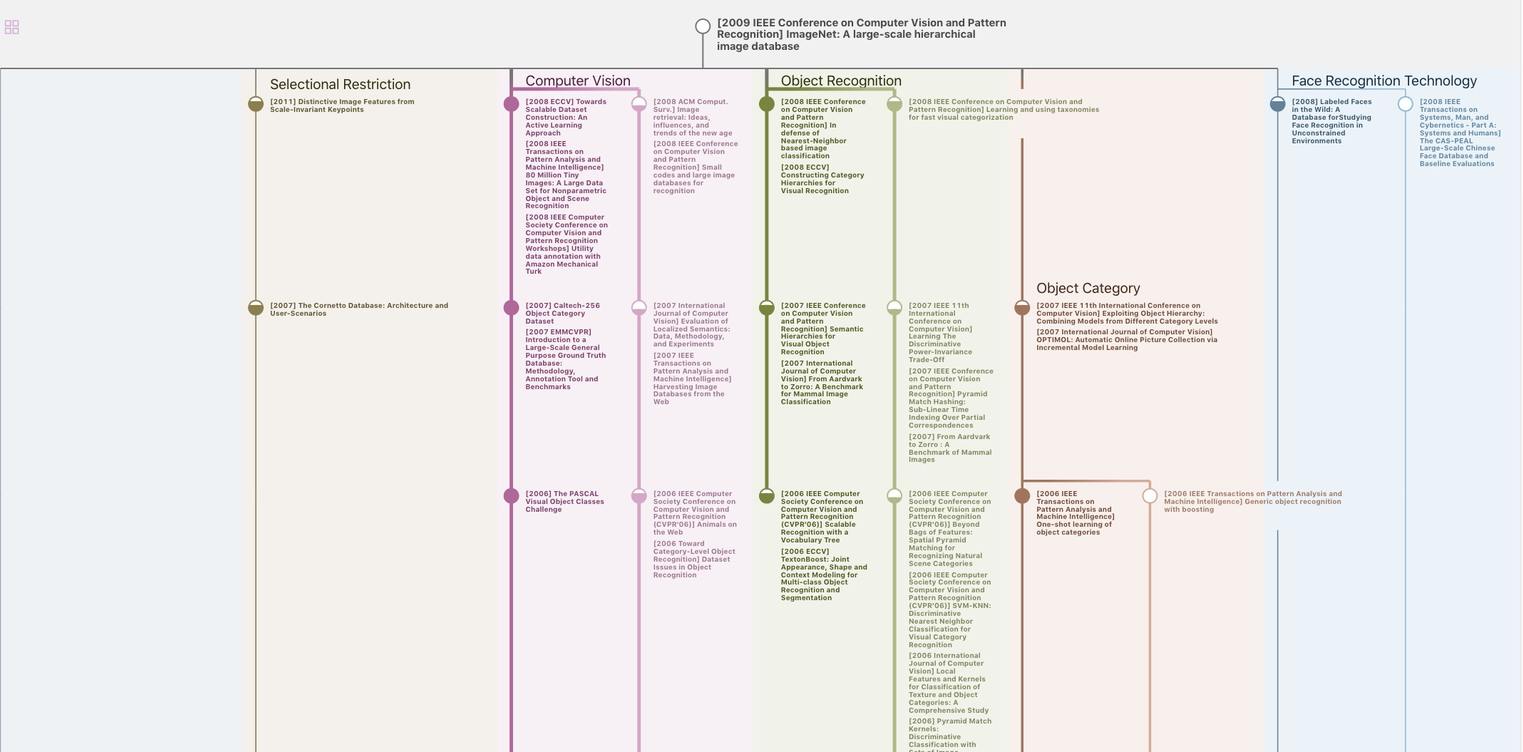
生成溯源树,研究论文发展脉络
Chat Paper
正在生成论文摘要